Exploring architectural identity of the city with 3D GAN and Stable Diffusion with case study of Kazan
This research proposes to explore the ability and accuracy in styding and representing architectural identity of the city through the set of artistic experiments with all generated 3d geometry and 2d images in order to meet expectations of the community of the building’s appearance. By converging supervised and unsupervised 3D Generative Adversarial Networks (GANs) with Stable Diffusion (+ControlNet) and recreation 3d geometry from the flat image, later on shaping it with loops of feedback from the local community, the workflow is presented.
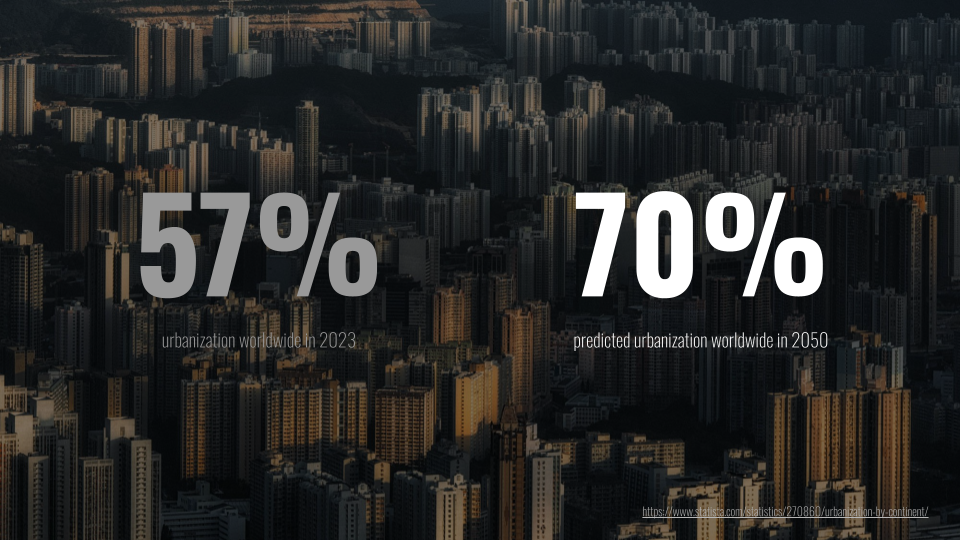
Cities evolve, transform as a result of numerous factors- technological, social, economic, environmental which in turn leads to similar homogenous styles being adopted.
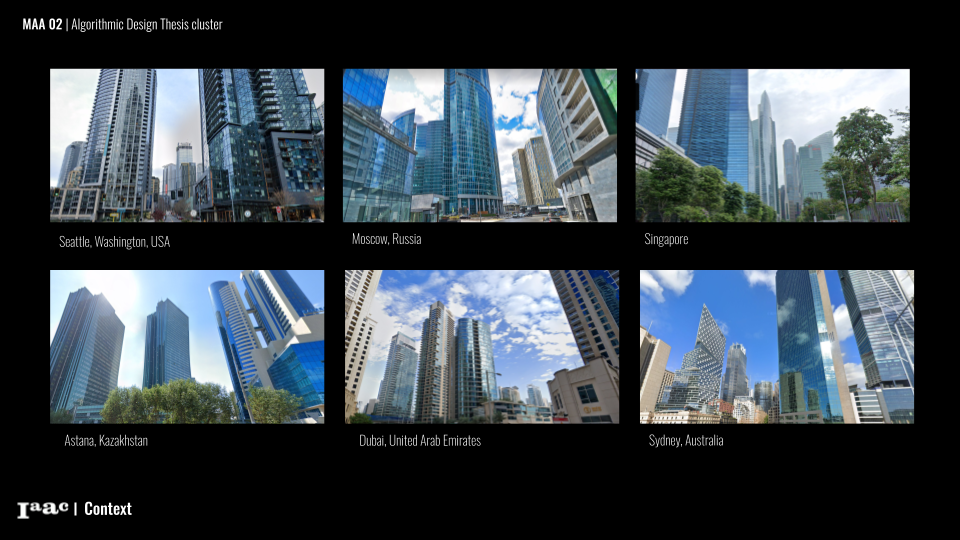
I’ll give you a few seconds to look at these photos of the new areas and try to guess from which places they are. They are not only from different countries, they represent different continents. Though, can cities continue developing without losing their unique urban identity?
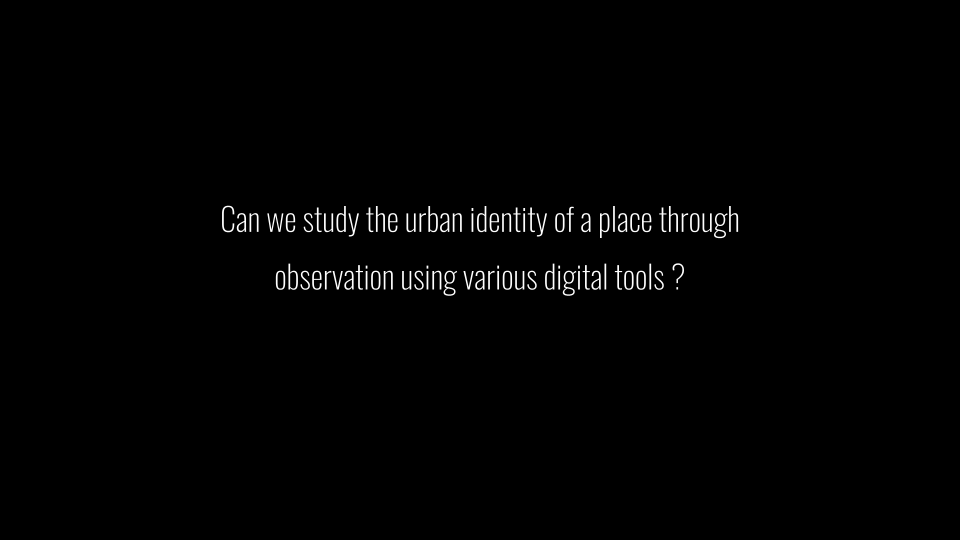
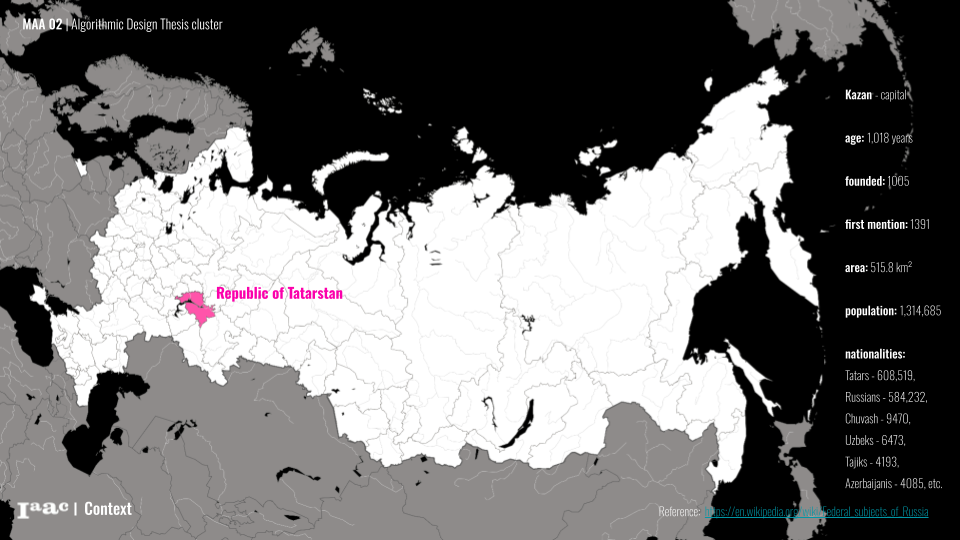
I am from the Republic of Tatarstan, with a capital of Kazan, which is located in the western part of Russia. As you may know, the Russian Federation is a multinational state, and is home to over 190 ethnic groups nationwide.
The Tatars, a Turkic group from Central Asia, have a history shaped by nomadic roots, Ivan the Terrible’s conquest, and being part of the Russian Empire and Soviet Union. These influences are reflected in their city’s architecture, language, and traditions.
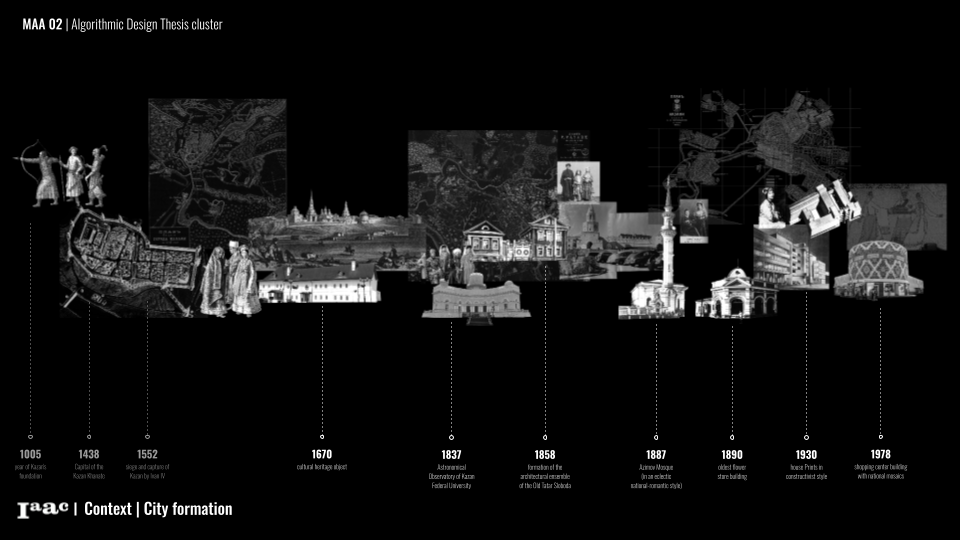
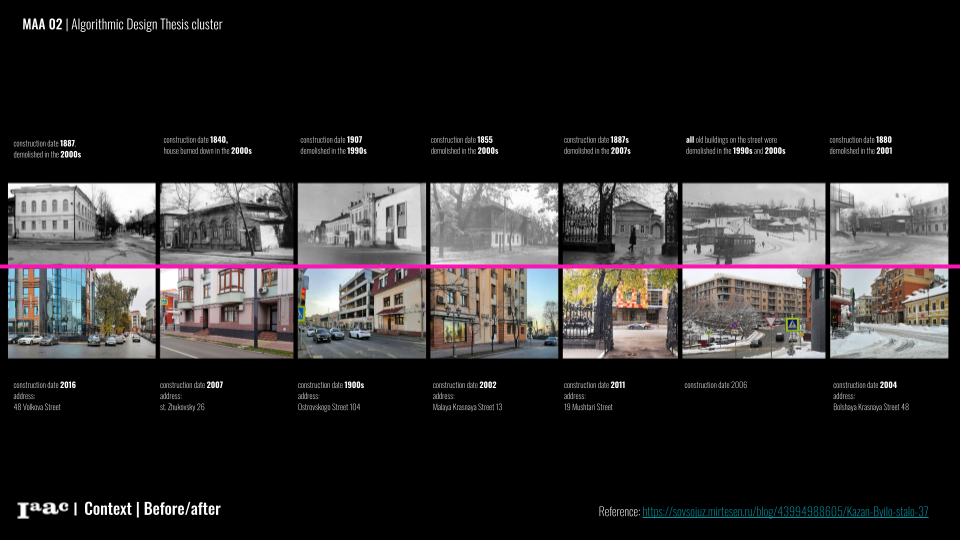
Here are before-and-after photos of Kazan from the same viewpoint, showing how demolishing and constructing buildings give new meanings to the environment. Noticing these remarkable changes in the city led me to initiation of this thesis project aimed at exploring architectural identity and understanding its importance for the community.
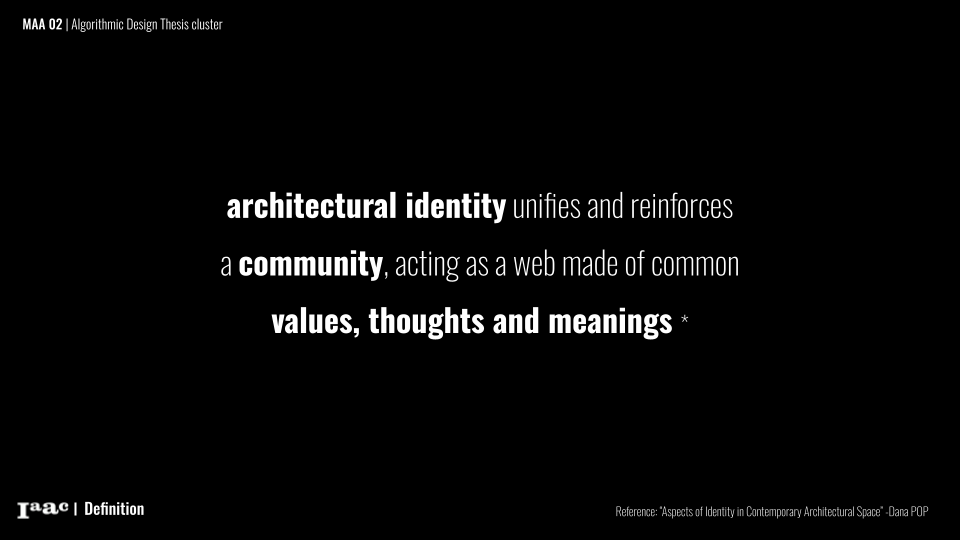
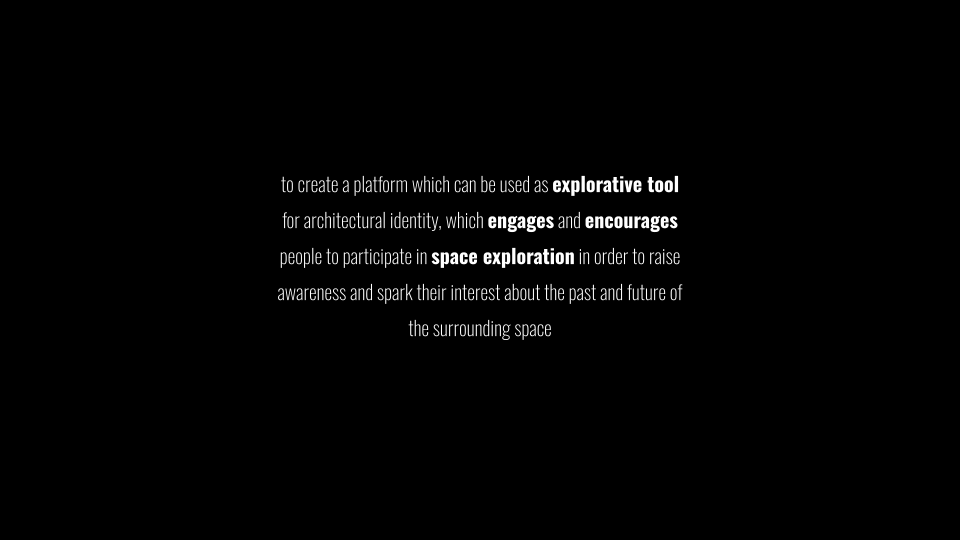
I found some related projects while going through the references. Upon reviewing 30 different projects, I selected 3 particular ones that are suitable for my work as they share the same idea.
Project 1 explores and compares outputs of textual and visual information in capturing the place identity of 31 global cities. On the one hand, the results indicate that generative AI models have the potential to capture the collective image of cities that can make them distinguishable. On the other hand, it works only with texts and images, without involving 3d.
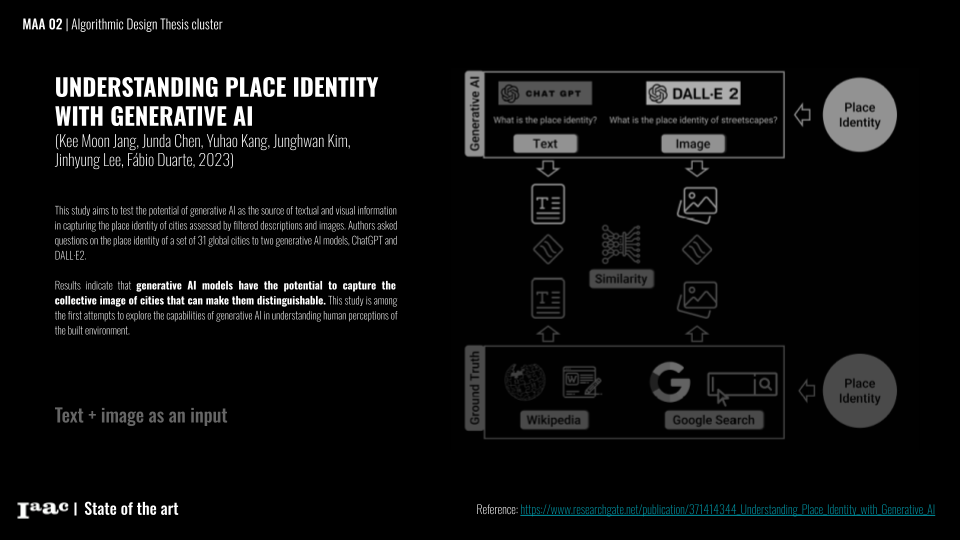
The project 2 outlines a method for generating building massings and layouts by integrating precise 3D building models with site context from cadastral and topographic data from open datasets in the Netherlands. The thing is that this project is very useful in terms of detailed explanation of workflow and methodology, but it is not aimed at exploring the city identity, track it or develop this concept, which can bring you to another level of discussion.
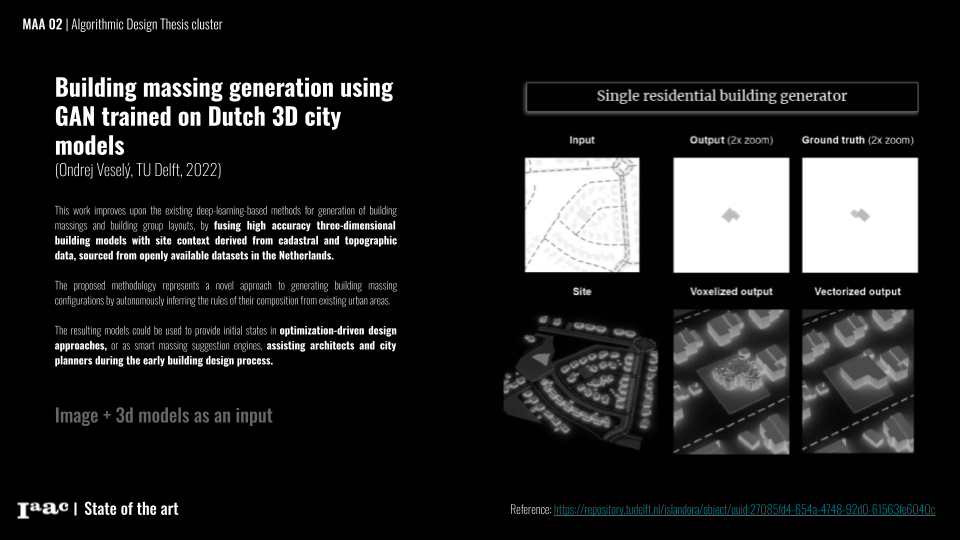
As an aesthetical reference and another approach to the existing technology I took the results of the project 3, which is a workshop of Benjamin Ennemoser and Ingrid Mayrhofer. Main advantage of their approach is that it uses comprehensive building datasets to create unique spatial configurations with interconnections across scales.
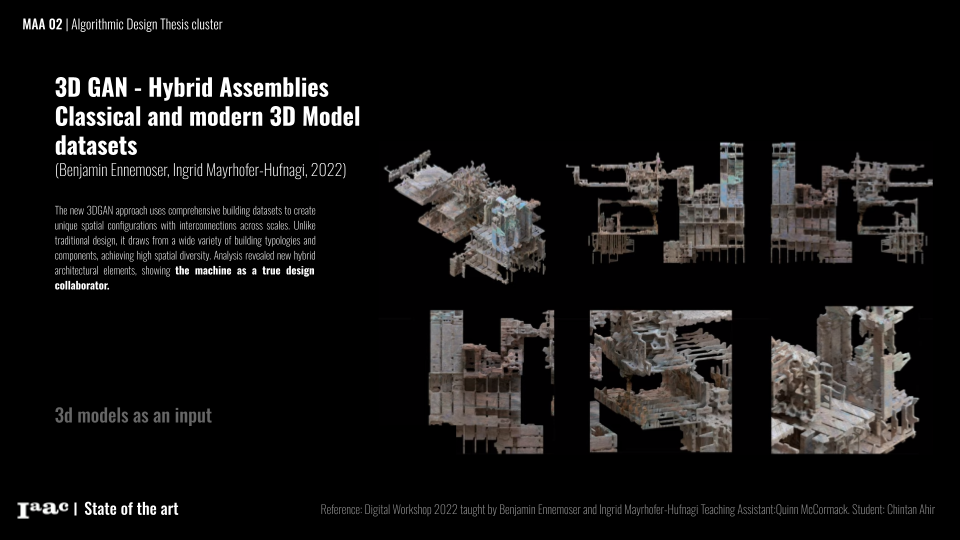
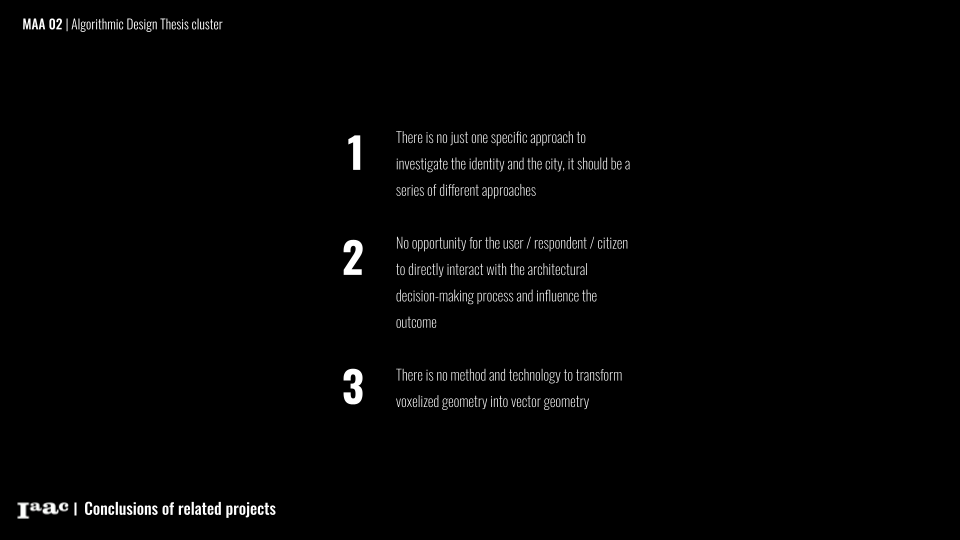
I decided to take a research paper called “Quantifying the City’s Identity” as a starting point and look at the elements of cultural heritage and aesthetic significance. I used both quantitative and qualitative methods in the research process.
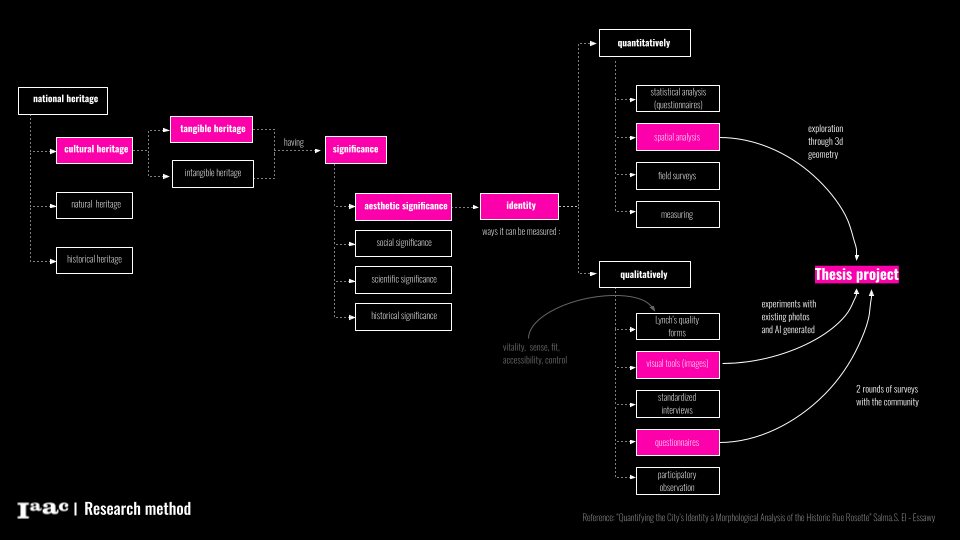
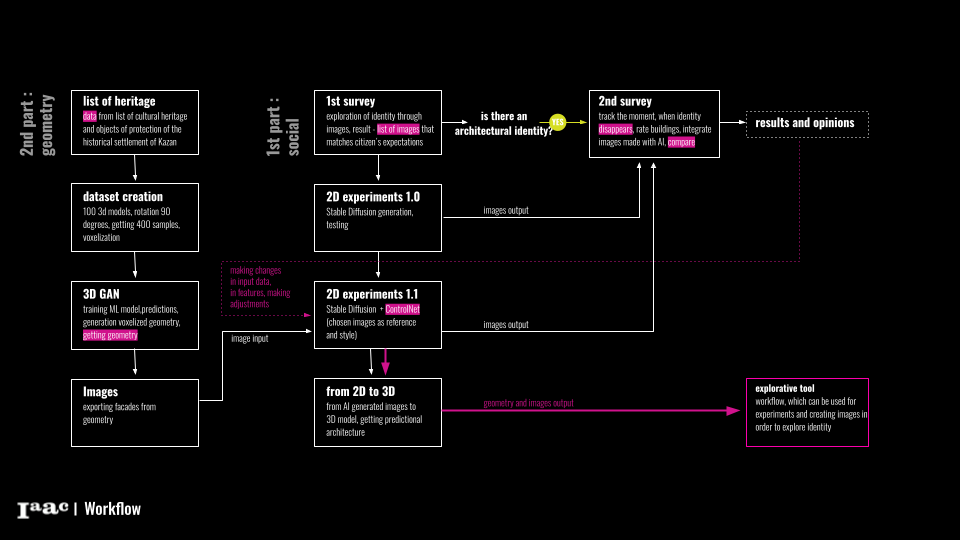
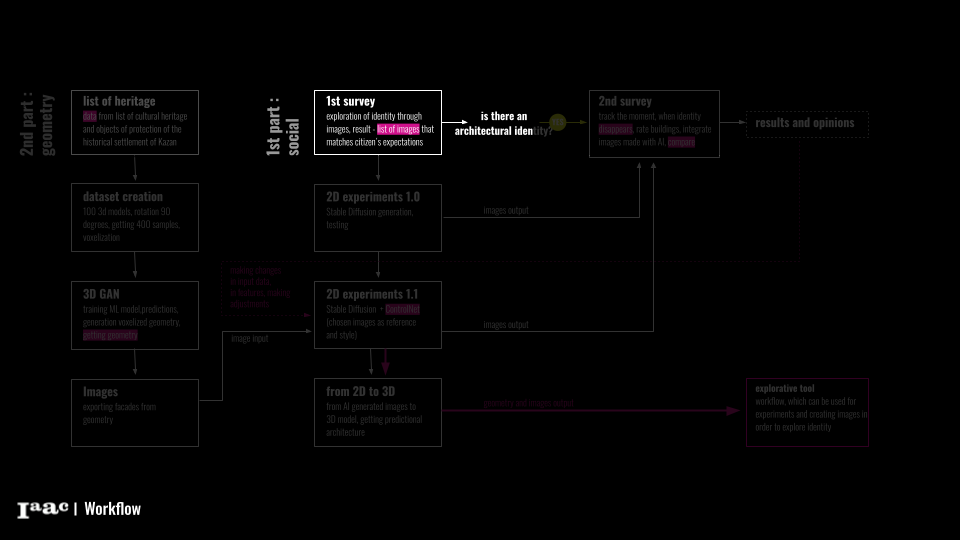
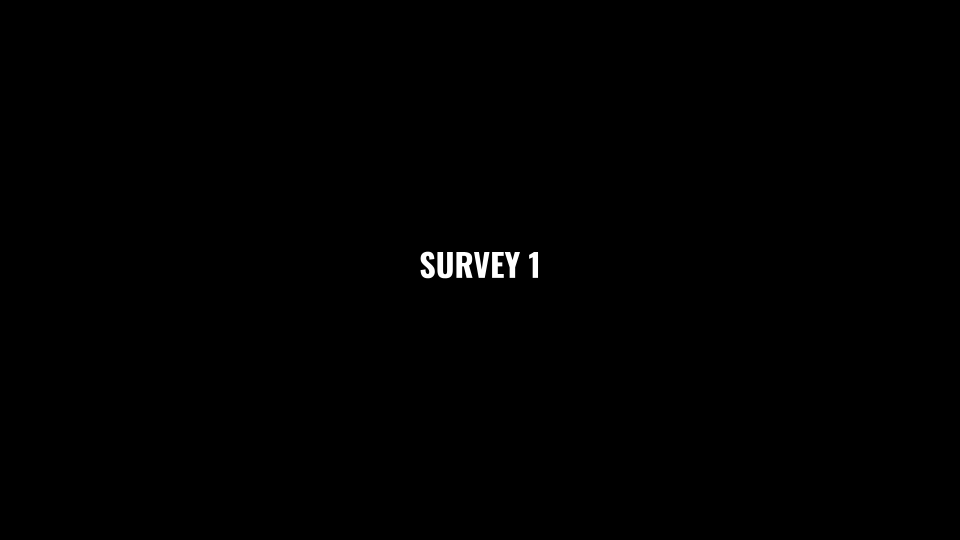
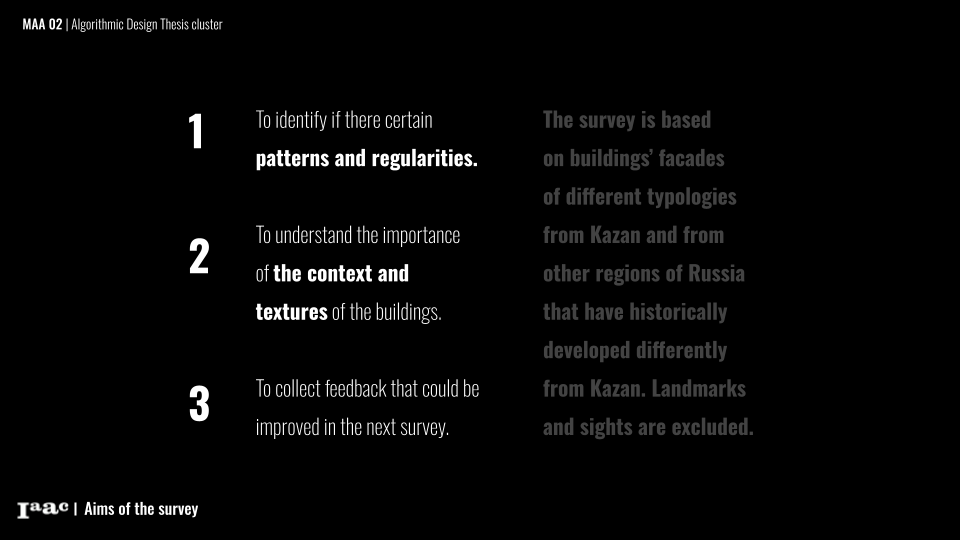
The respondents are 250 Kazan citizens, of different age groups and professions who subscribed to the telegram chat about architecture with 6000 subscribers overall.
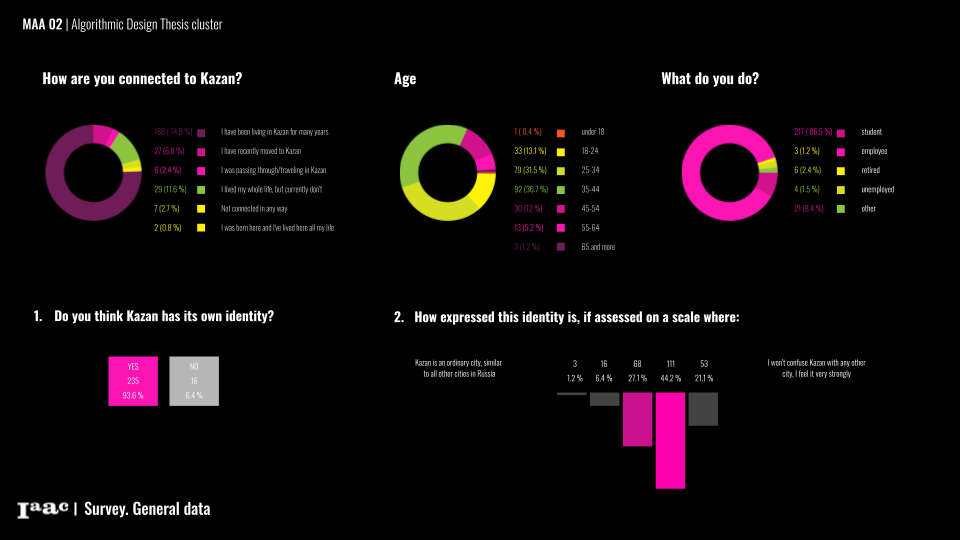
The main set of questions was about picking photos of the buildings from Kazan compared to other cities and regions. Color coding here and in follow-up questions is used to show the right answer. Green border color is used for showing results which are wrong but were chosen by the majority.
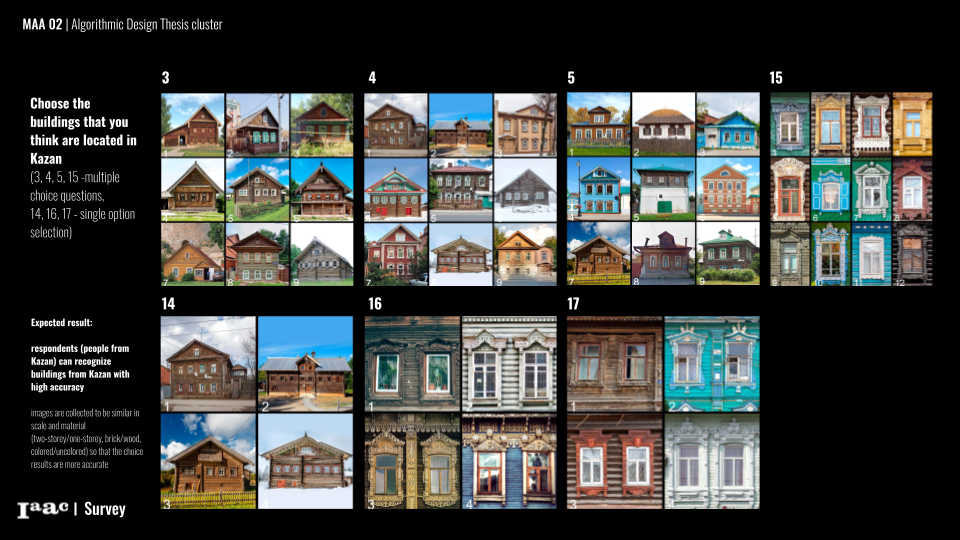
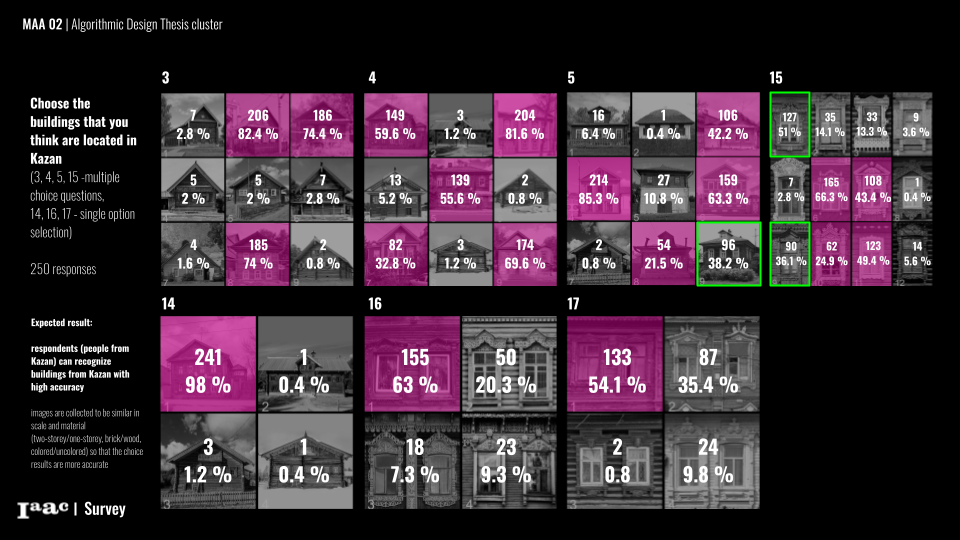
Next set consists of single option selection questions, and I expected people to recognize buildings that are located in Kazan.
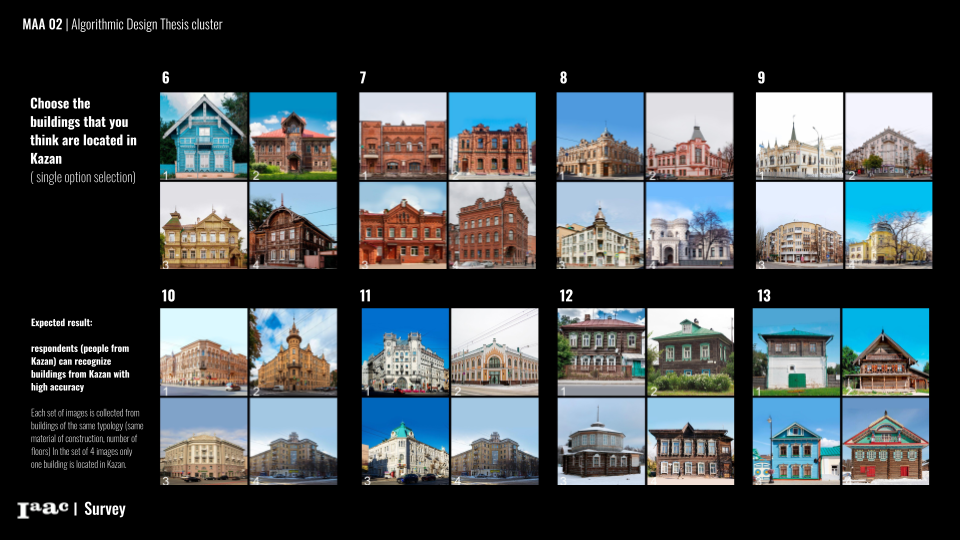
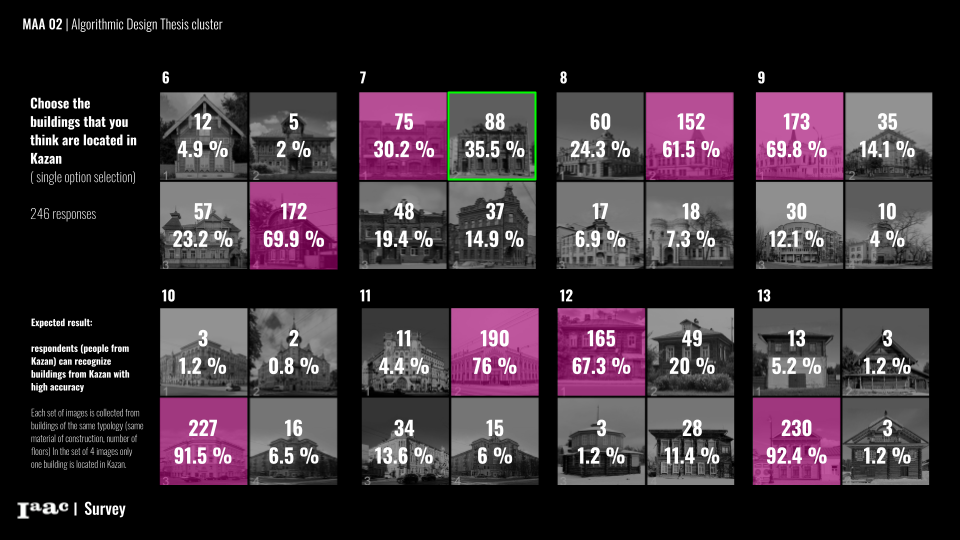
Next interesting set of questions is about silhouettes, composition and rhythms. And results are low, compare photos from previous parts. These results show that it’s impossible to isolate buildings from the context and materiality.
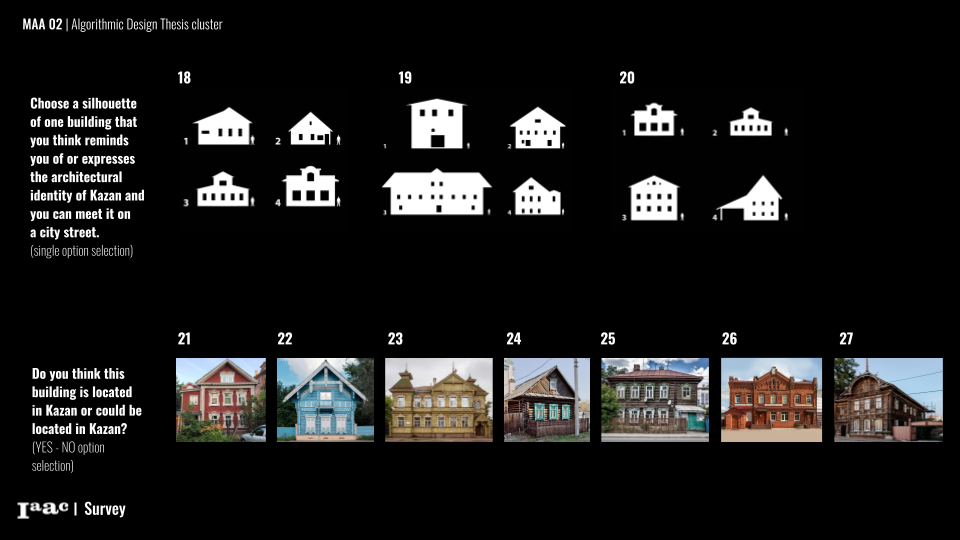
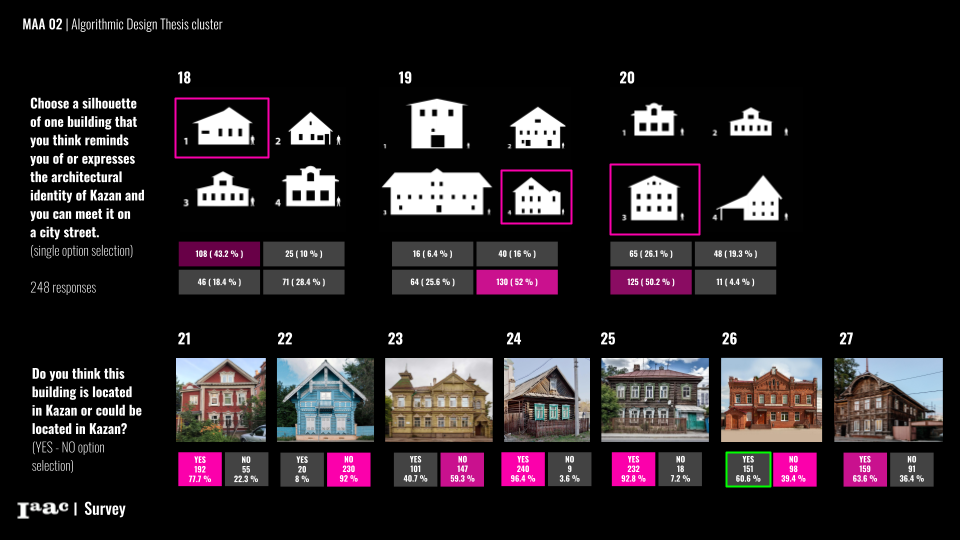
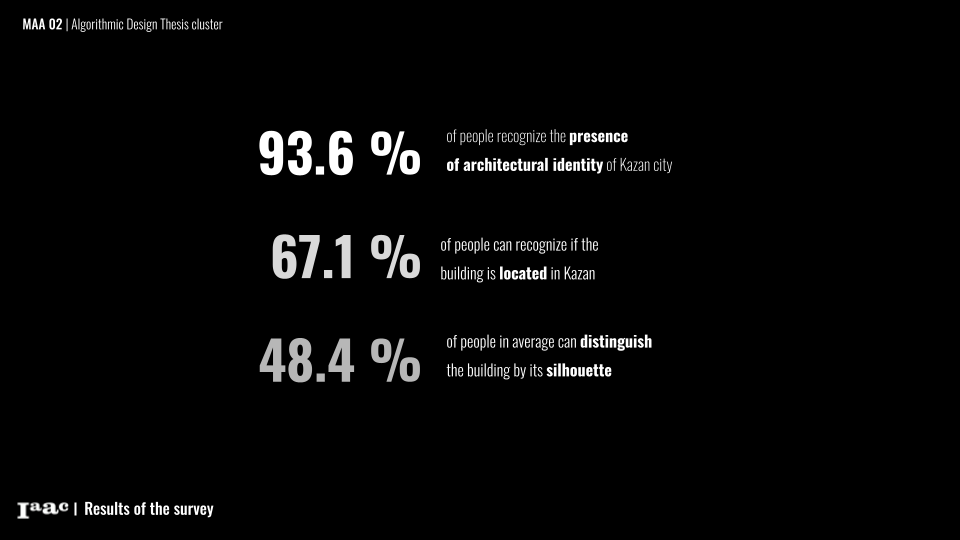
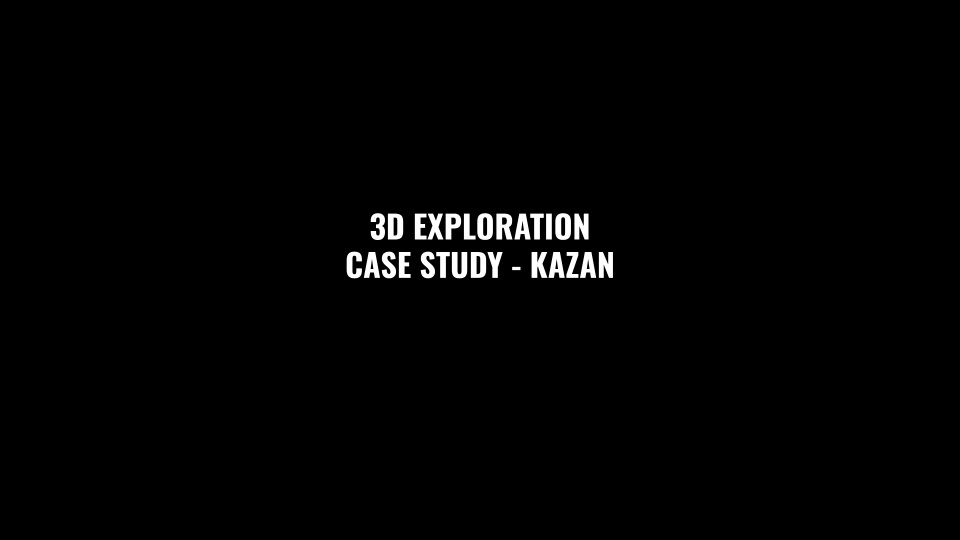
For the digital tool I chose 3d generative adversarial networks, it is a leading deep generative model that uses deep neural networks (DNNs) to train on a set of training examples to generate new design instances with a degree of flexibility and accuracy that is superior to competing generative approaches.
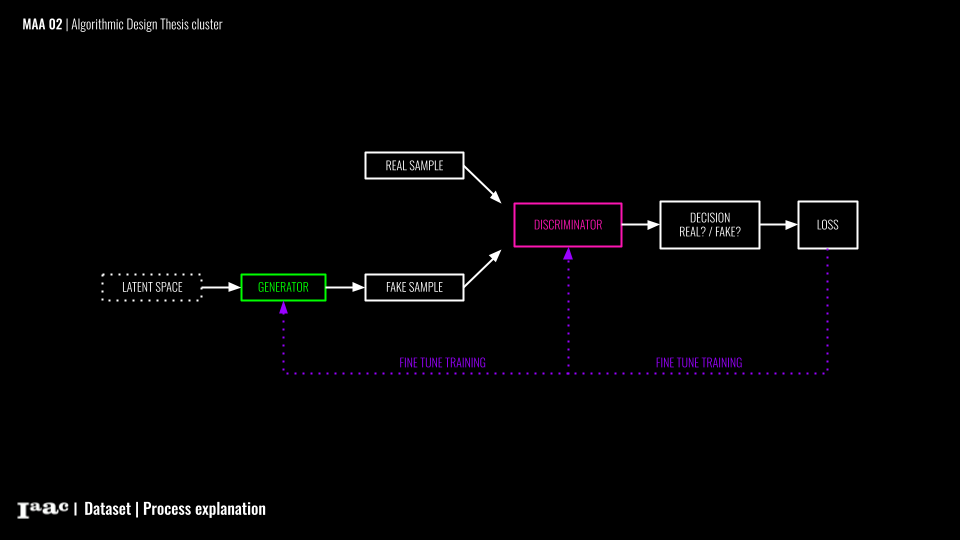
I created a dataset by manually making 100 3D models of buildings from two official documents on Kazan’s historical and cultural heritage. Due to the lack of available 3D models in Russia, I used satellite and open-source data. Since I couldn’t explore all typologies separately, I merged buildings of different scales and morphologies to see how the GAN model would interpret their geometry independently.
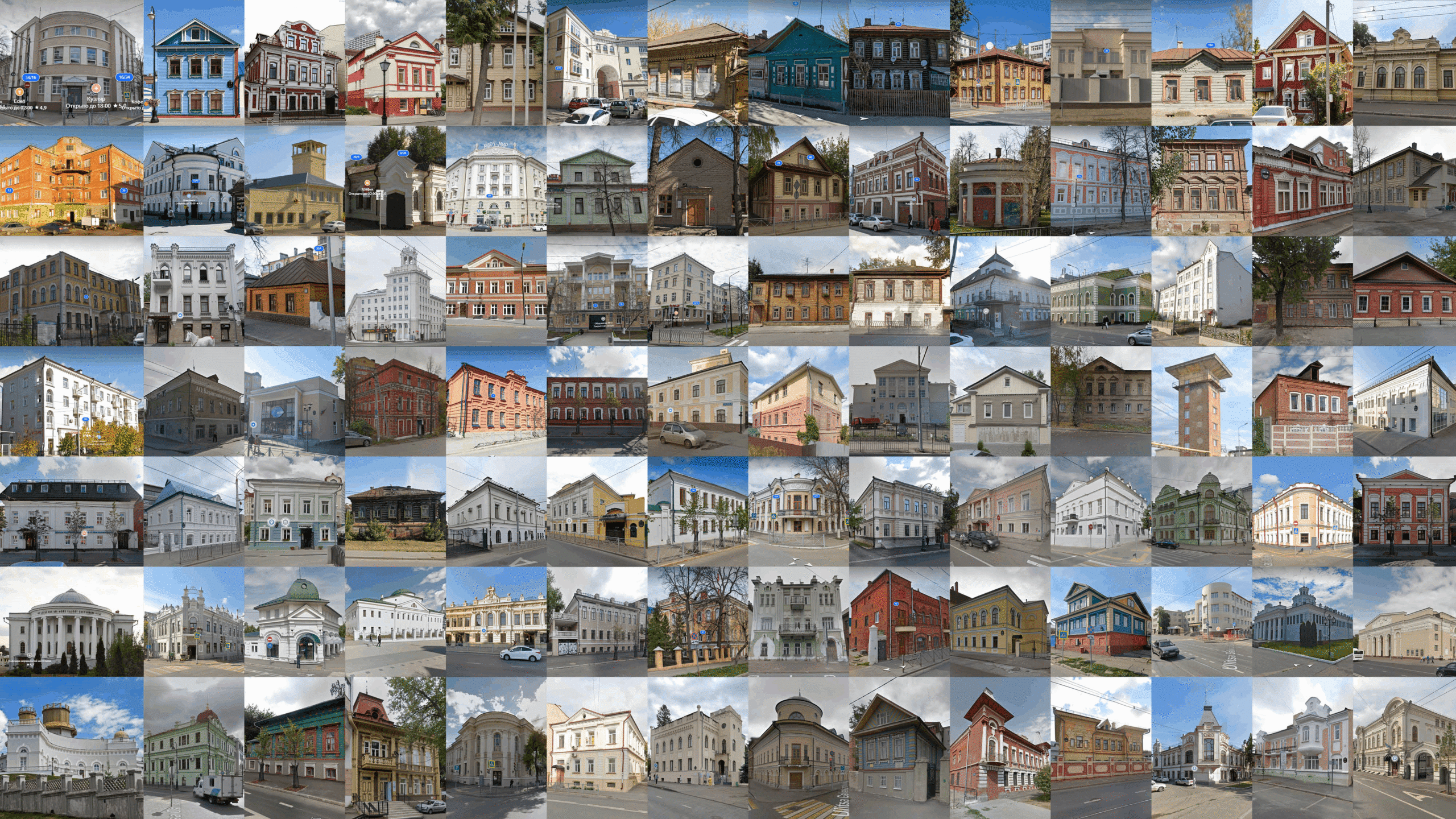
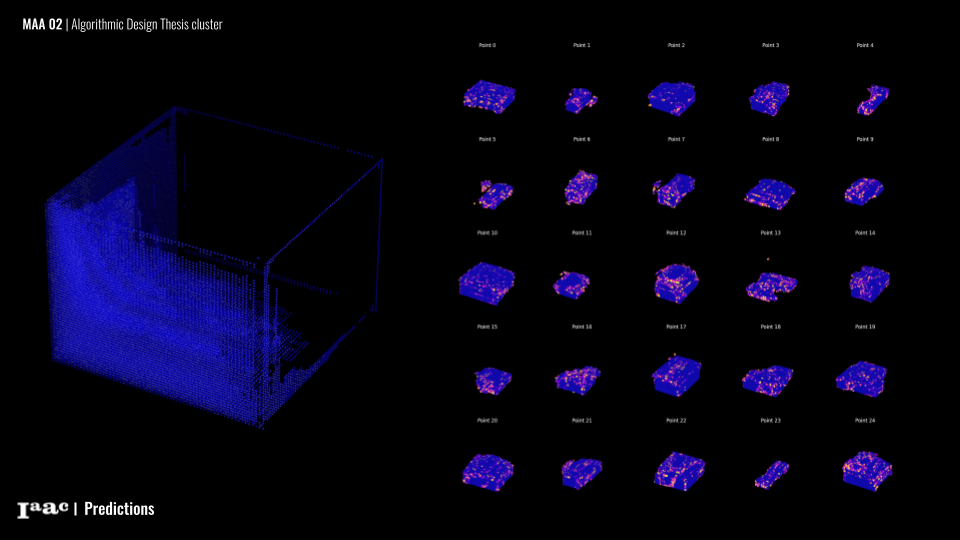
Here are voxel shapes reconstructed from text data. Predicted results appear as lines of 1s and 0s, needing reconstruction for visual clarity. The slide compares predictions: shell (empty inside) vs. solid geometry. Solid data inputs yield more stable results.
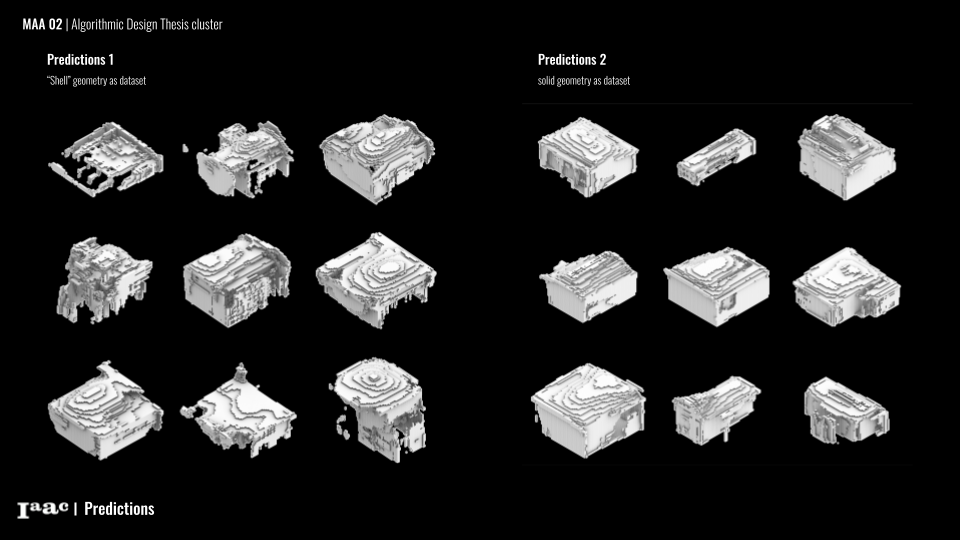
To recap, looking at the research map. We have a predicted geometry, but it doesn’t contain data about textures that are needed for people to recognize buildings better. We have results of the 1st survey that gave a general idea of architectural identity existence. Now I’ll combine two branches to generate images of buildings, not just as shapes but as textured structures within the city’s context.
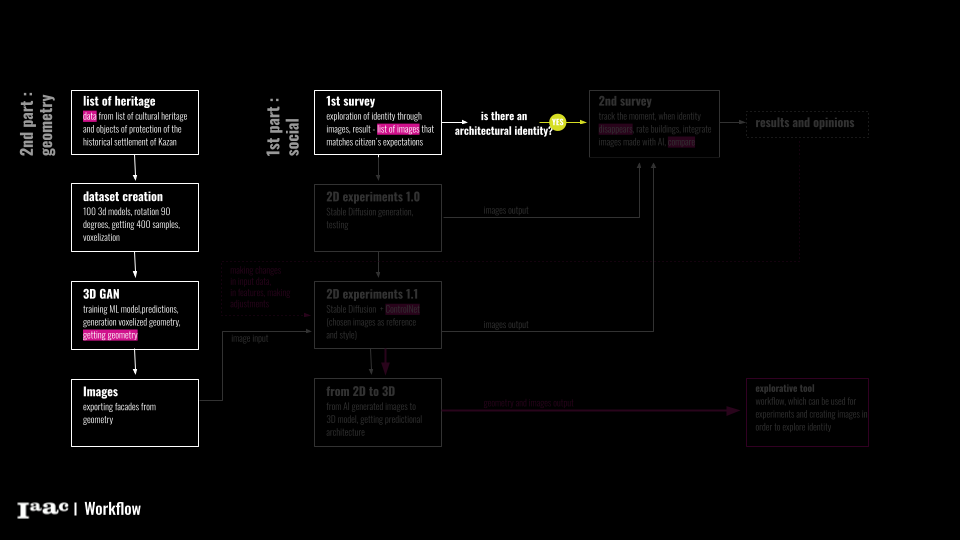
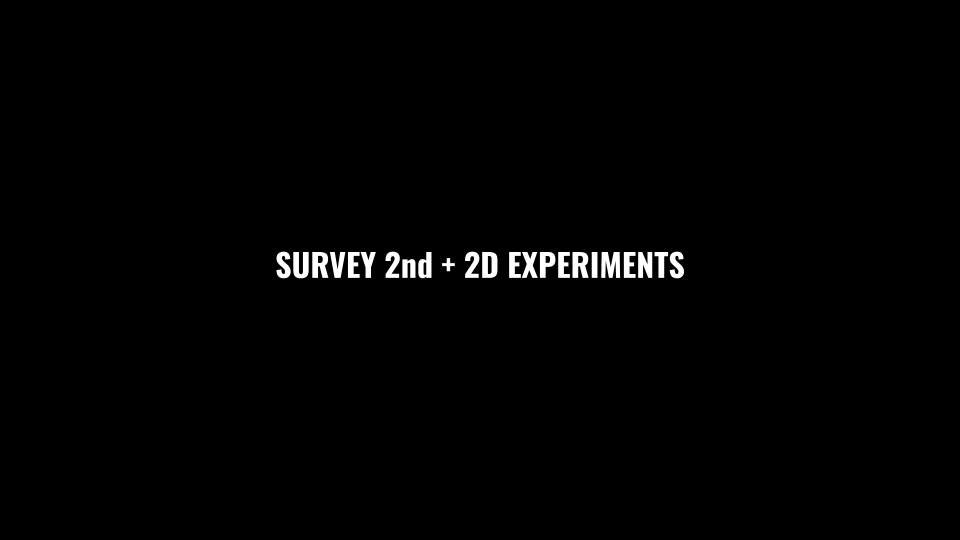
The main goal of the 2nd survey was to designate boundaries and limits of people’s perceptions and emotions, what they are ready to accept as an architectural identity of Kazan and what is far from their vision and expectations.
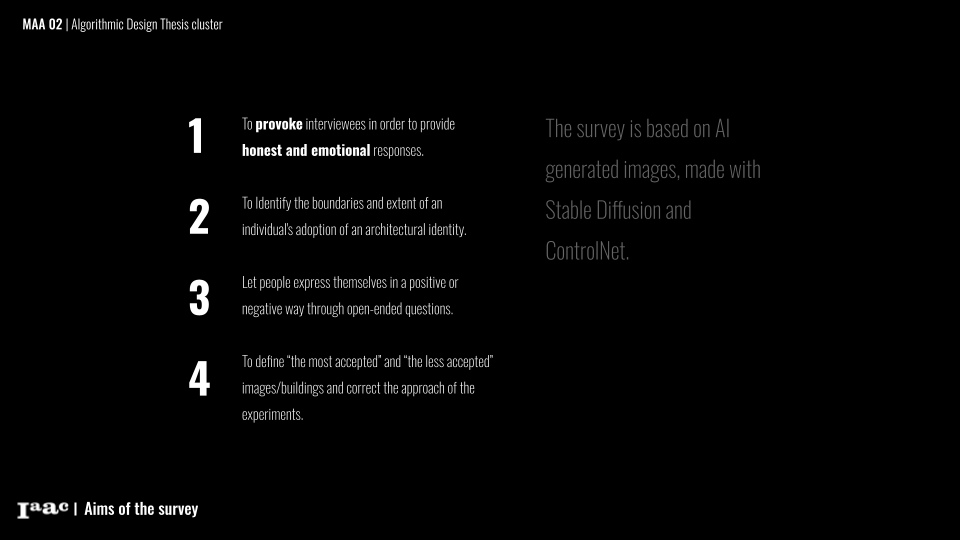
In survey 2, 40 respondents were the same individuals from survey 1. The first question was open-ended, asking them to describe their overall perception of Kazan’s architectural identity.
The architectural identity of Kazan, as described by the survey responses, emphasizes Tatar national elements and historical influences, with a preference for low-rise, symmetrical buildings that incorporate traditional motifs and natural materials. Kazan’s architecture is seen as a blend of classical and modern styles, often featuring bright colors, wooden decorations, and intricate details. Respondents appreciate a mix of old and new, highlighting the city’s unique blend of Eastern and Western influences, its cozy, green environment, and memorable, culturally resonant structures.
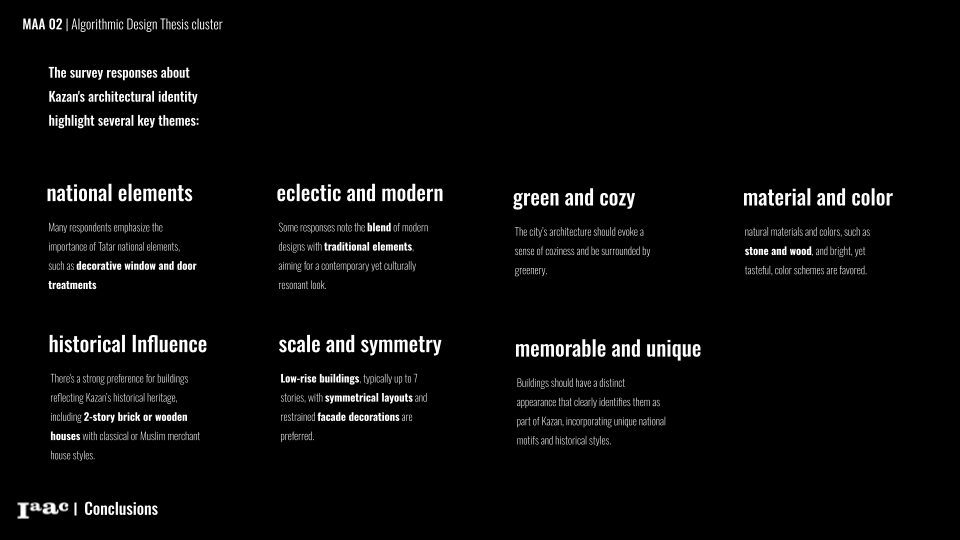
The survey uses AI-generated images, specifically leveraging Stable Diffusion and ControlNet. One building was selected as the initial image and then mixed with different styles within the program.
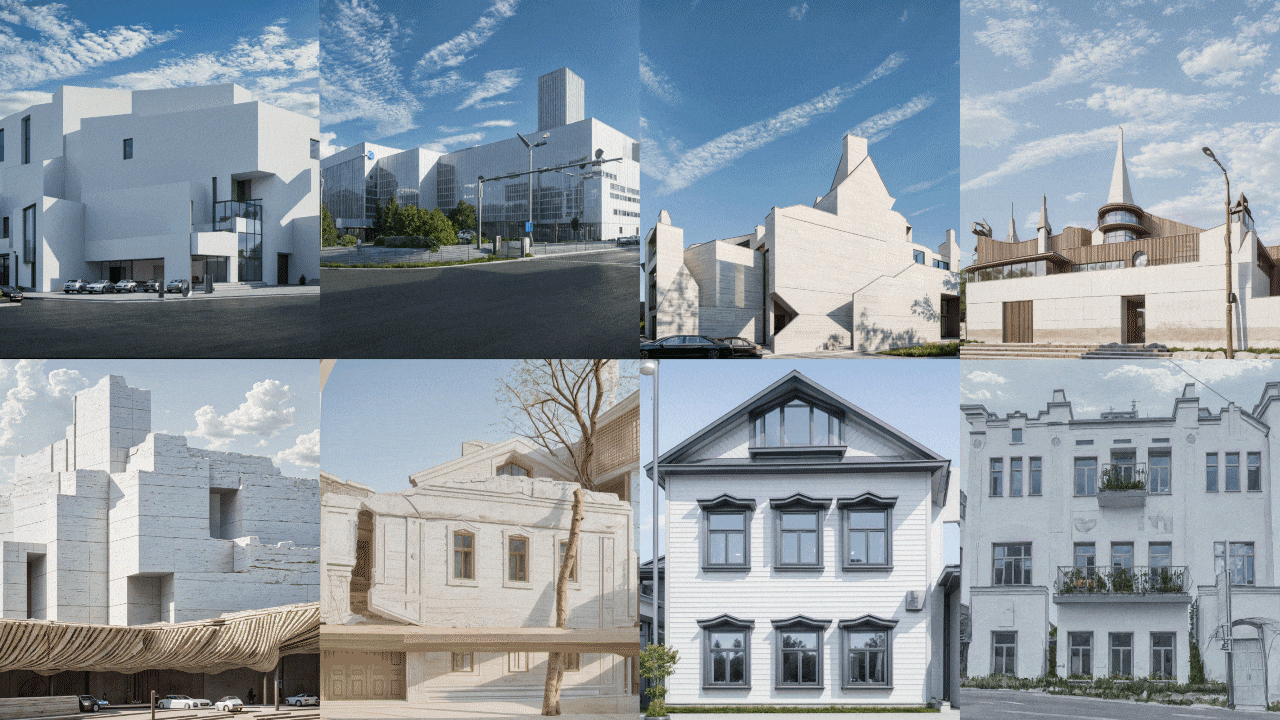
Here we have a set of questions with initial images and their iterations, and the question was: Which of the images of buildings maintain their belonging to the architectural identity of Kazan after the experiments? As an answer people had an option “none, so none of these options maintain their belongings to Kazan identity. Focus on the pink “none” rectangle and its percentage in different cases, as well as the buildings with higher positive percentages. This will help you visually compare which buildings are better recognized (more accepted) and which are not. I’ve included a wide range of options, some of which are very unconventional.
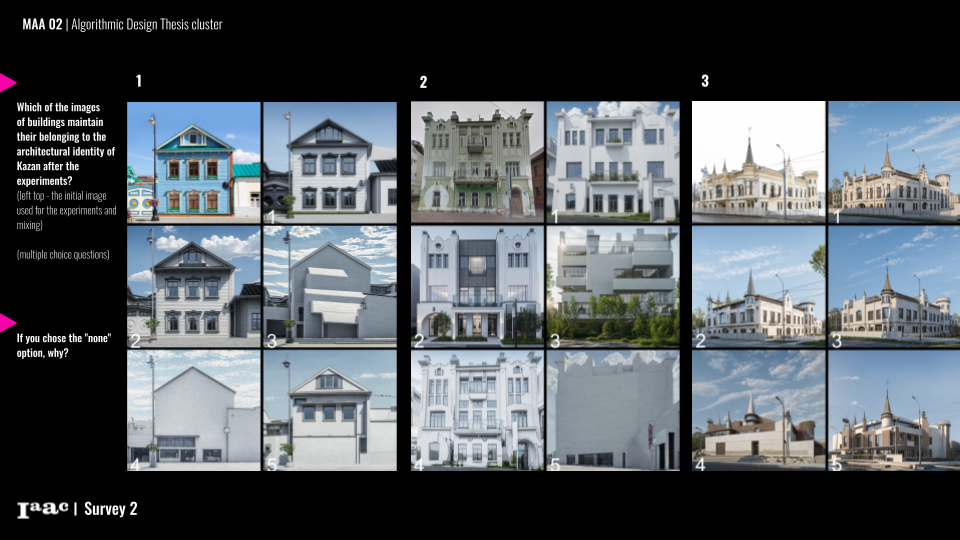
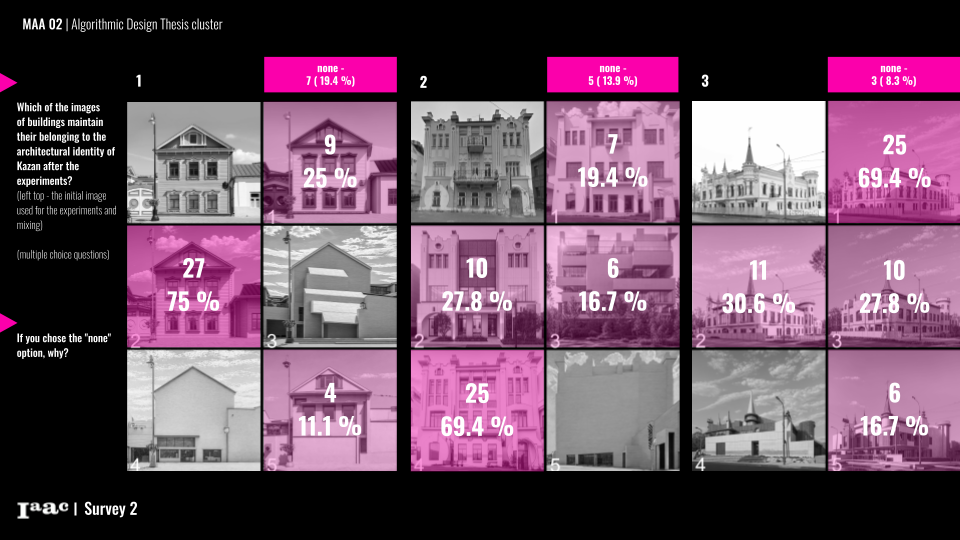
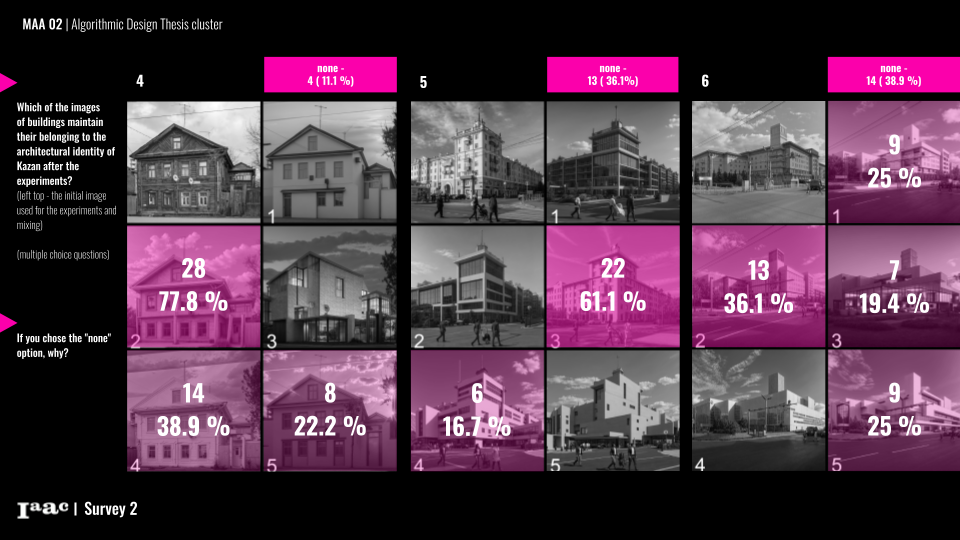
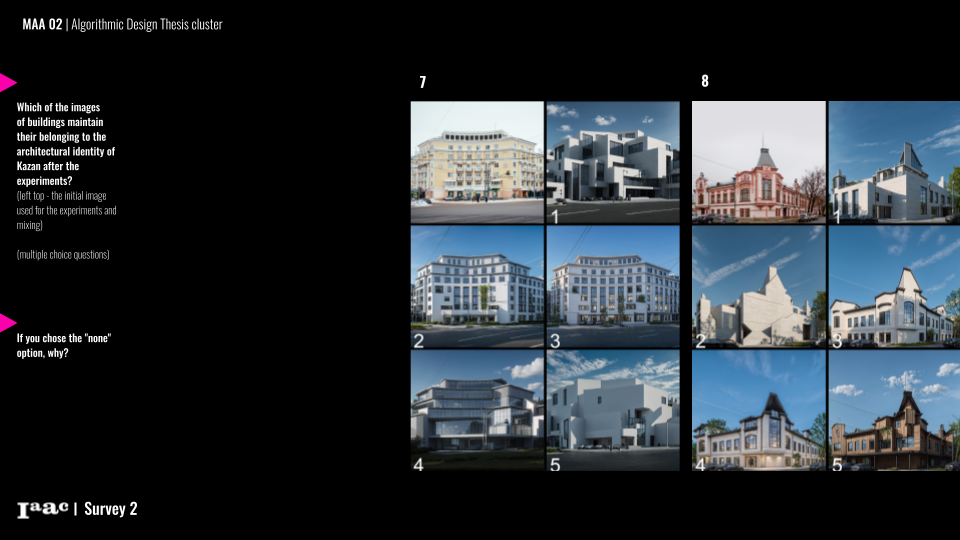
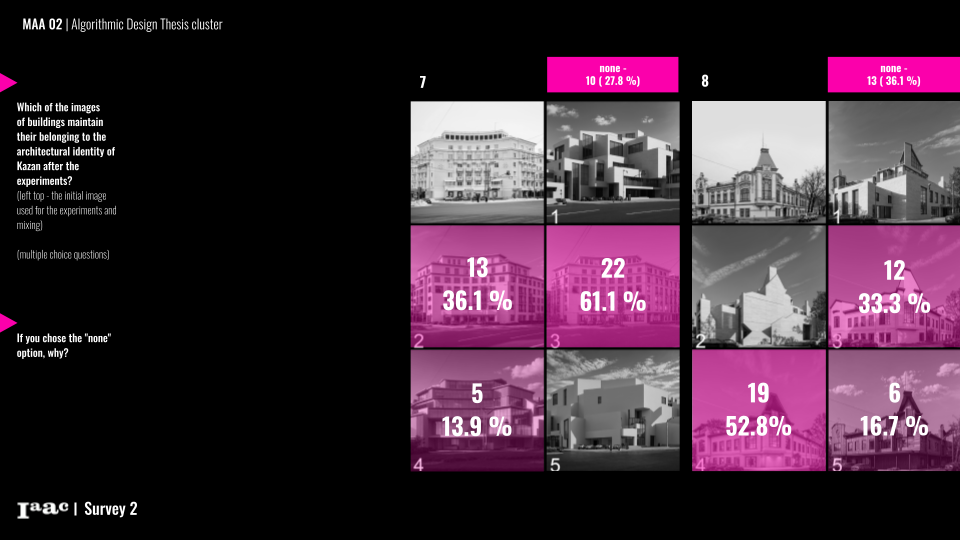
In the second part of the survey, I used a different image generation approach to test geometry. Since predicted voxelized geometry lacks texture, material, and context data, I mixed a predicted building with an existing one that reflects architectural identity. Using various filters in ControlNet and Stable Diffusion, I obtained diverse results for comparison.
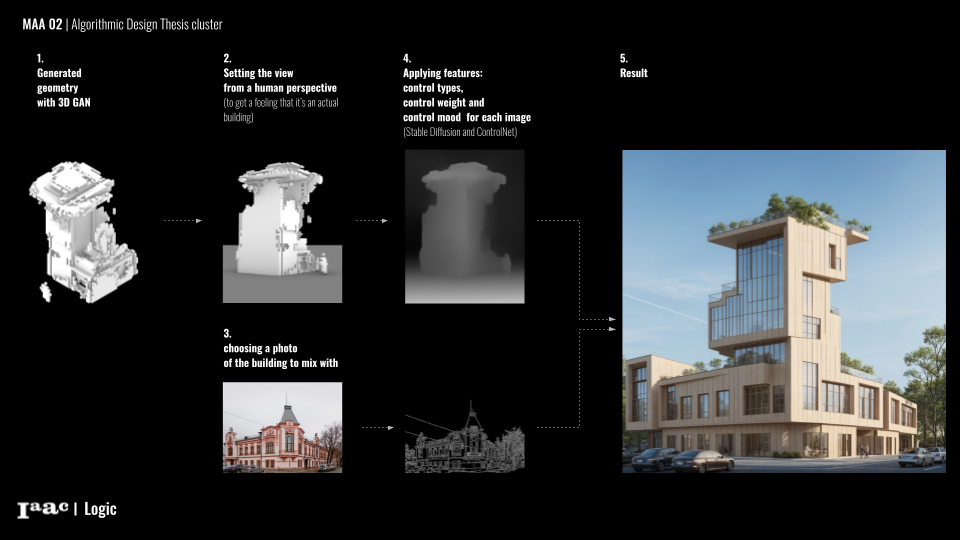
Moving to questions. People were asked, Are there any buildings among these images that, in your opinion, could be built in Kazan in the far future and would match the architectural identity of Kazan? And as additional questions there are If you chose the “none” option, why?
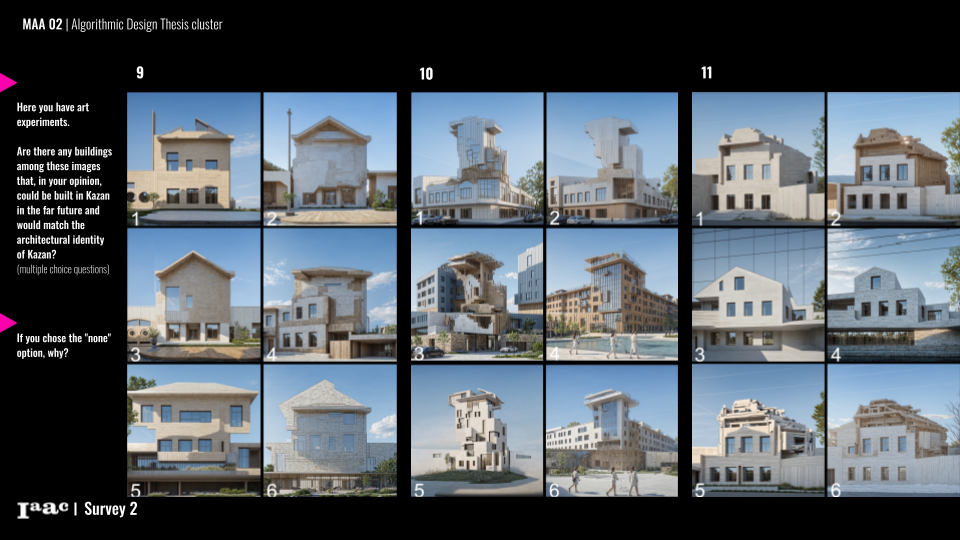
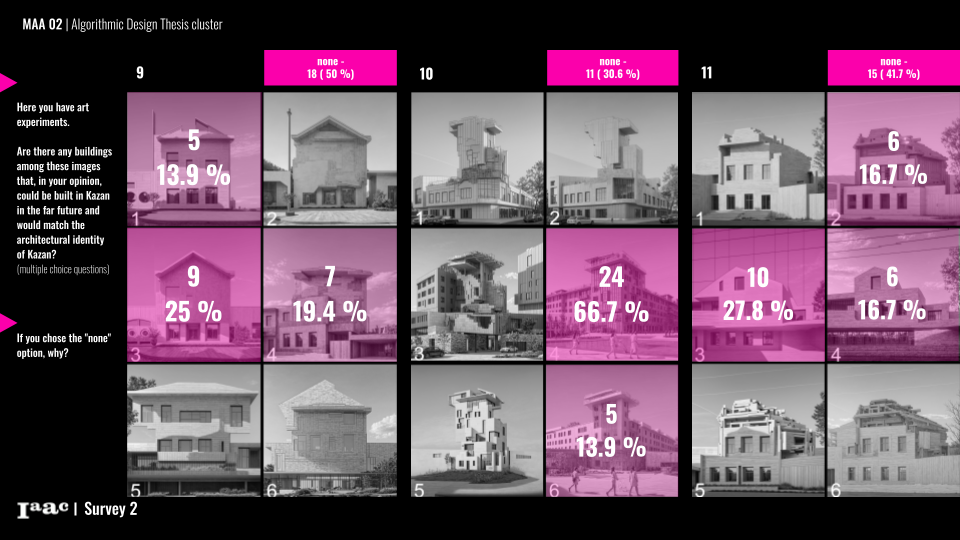
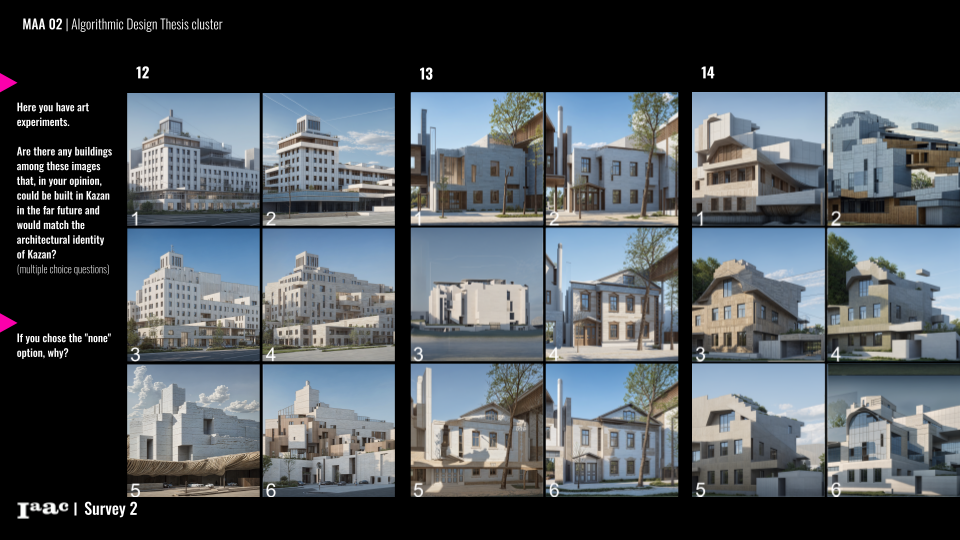
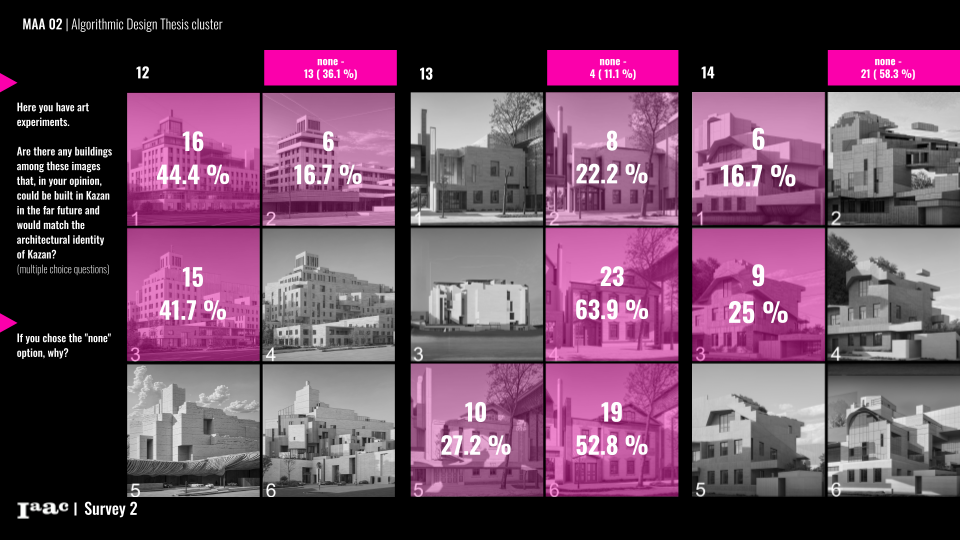
After each group of images in the survey, participants were asked what reminded them of Kazan’s identity or why they felt none of the images did. Most feedbacks mentioned a lack of national motifs, ornaments, and appropriate color schemes as major issues. Common descriptions included “too modern,” “monotonous,” “no identity,” and “ordinary new development.” Participants desired elements reflecting Tatarstan’s and Kazan’s unique architectural styles, such as historical features, national details, and vibrant colors.
For images that did remind participants of Kazan’s identity, the most frequently mentioned aspects were shape, windows, volume, simplicity, proportions, materials, and details. References to Stalin-era buildings, historical homes, and specific existing structures in Kazan were common. Themes also included the use of glass, wood, traditional elements, and a preference for clean, balanced, and harmonious designs, emphasizing historical continuity with modern touches.
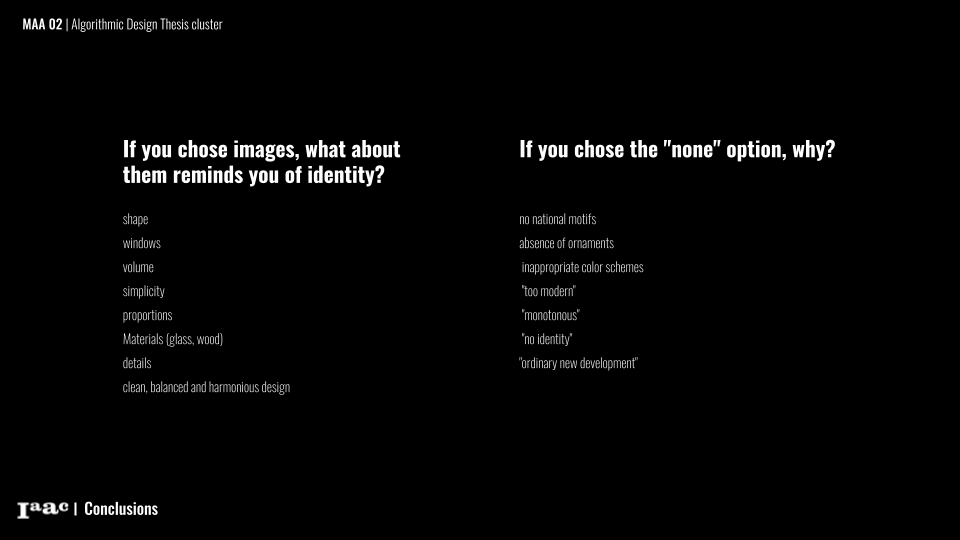
As part of my experiments, I’ve attempted to convert 2D images back into 3D geometry to visualize buildings with all their features. However, a significant challenge is the lack of an open-source tool that offers precise control over this transformation. While the results from the Tripo platform are promising, further development is necessary. It’s intriguing to witness AI’s role as a co-author in shaping forms and structures through latent space, suggesting potential for growth despite current limitations.
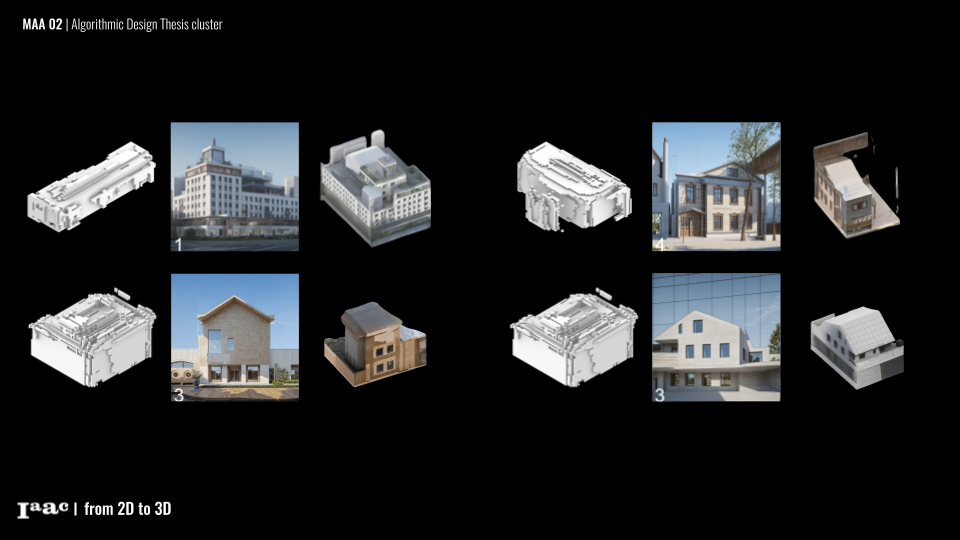
After all the experiments, let’s go back to the main goal of the project – creating a platform that can be used as an explorative tool for architectural identity. I’ve created a short video that shows user experience on this platform.
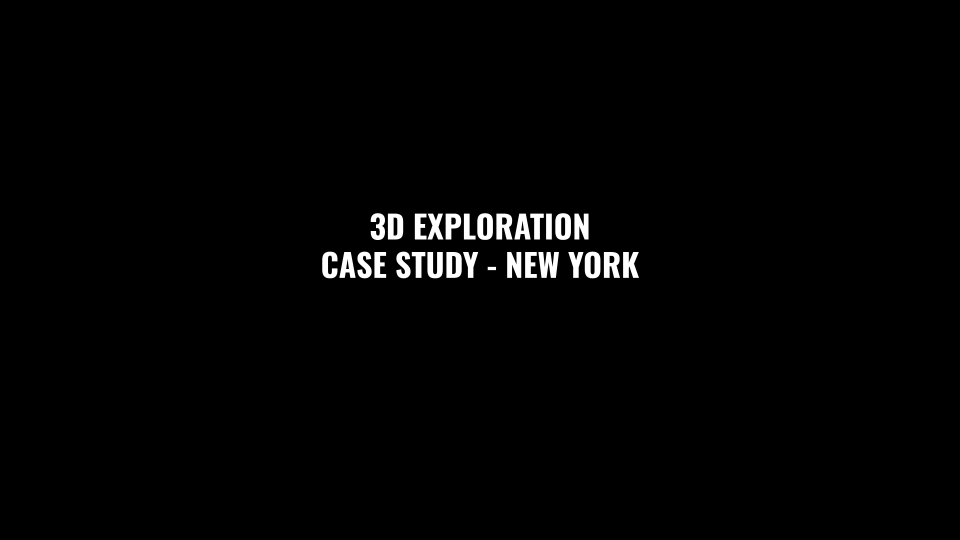
To test the workflow that I already demonstrated, I decided to experiment with a more recognizable city in mass culture, so.. New York. For the dataset I choose 140 3d models, mostly from Manhattan.
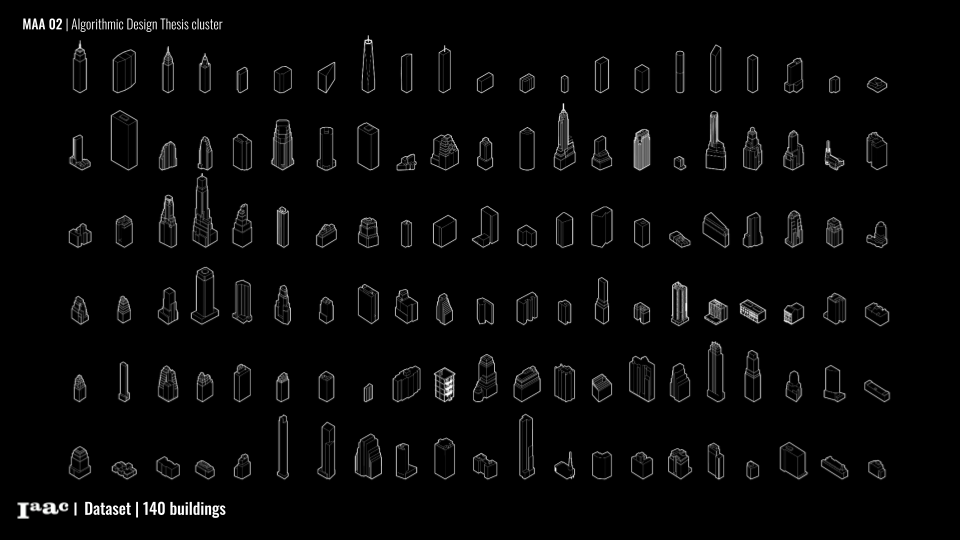
I trained the model with a new dataset and got interesting predictions. Here we have a voxelized geometry, predictions of the GAN model, how buildings can look like according to the input data.
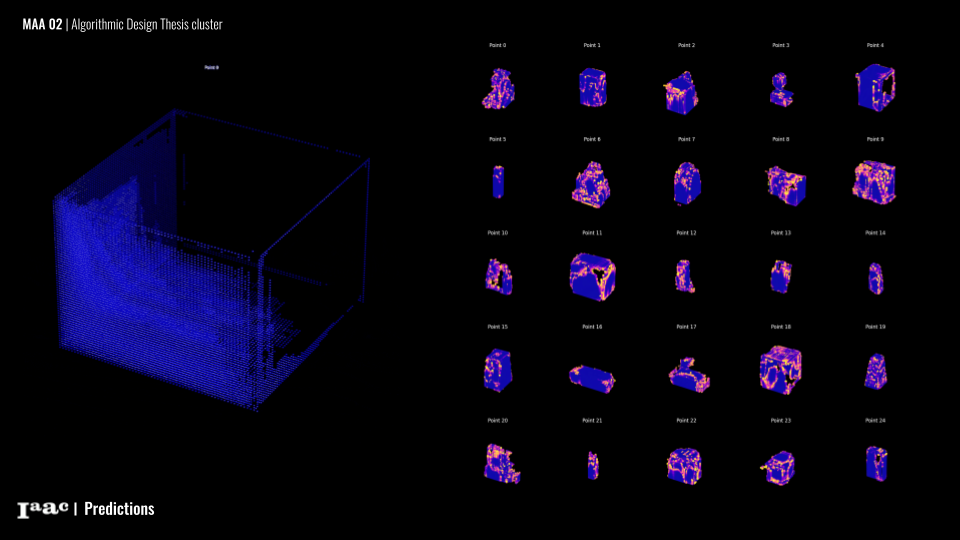
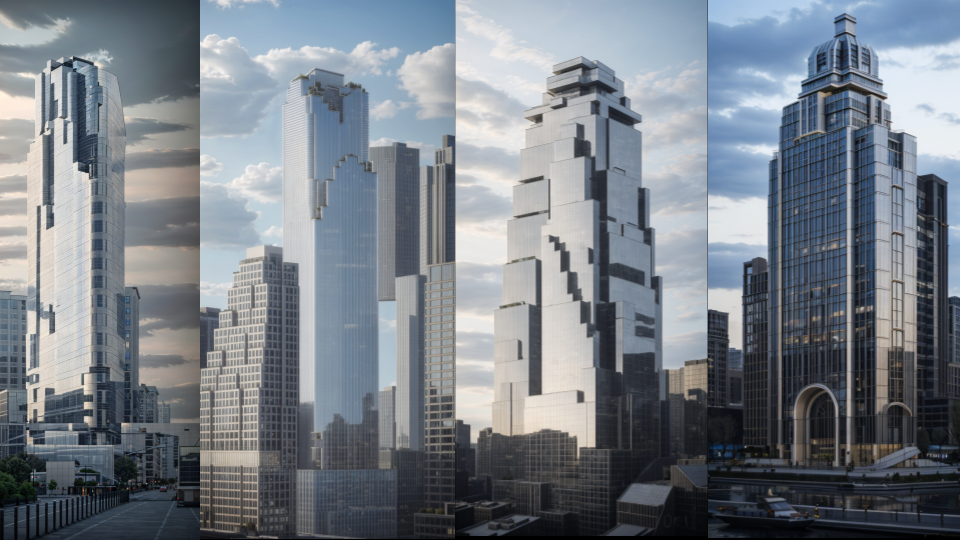
Here you have generated images, predictions, how in the future buildings with New York architectural identity can look like based on the collaboration with digital tools. So, now it’s your turn to decide, if the buildings have this identity or no.