The BCN Building Stock project aims to assess the condition of the building stock in Barcelona (BCN) and identify potential buildings that require renovation or demolition. This analysis is crucial for urban planning and sustainable development initiatives, as it provides insights into the state of the city’s building stock. By leveraging data analysis techniques and machine learning algorithms, we can classify and cluster buildings based on various attributes and identify areas with the highest concentration of structures needing attention. This analysis provides recommendations for prioritizing renovation efforts and optimizing urban resources.
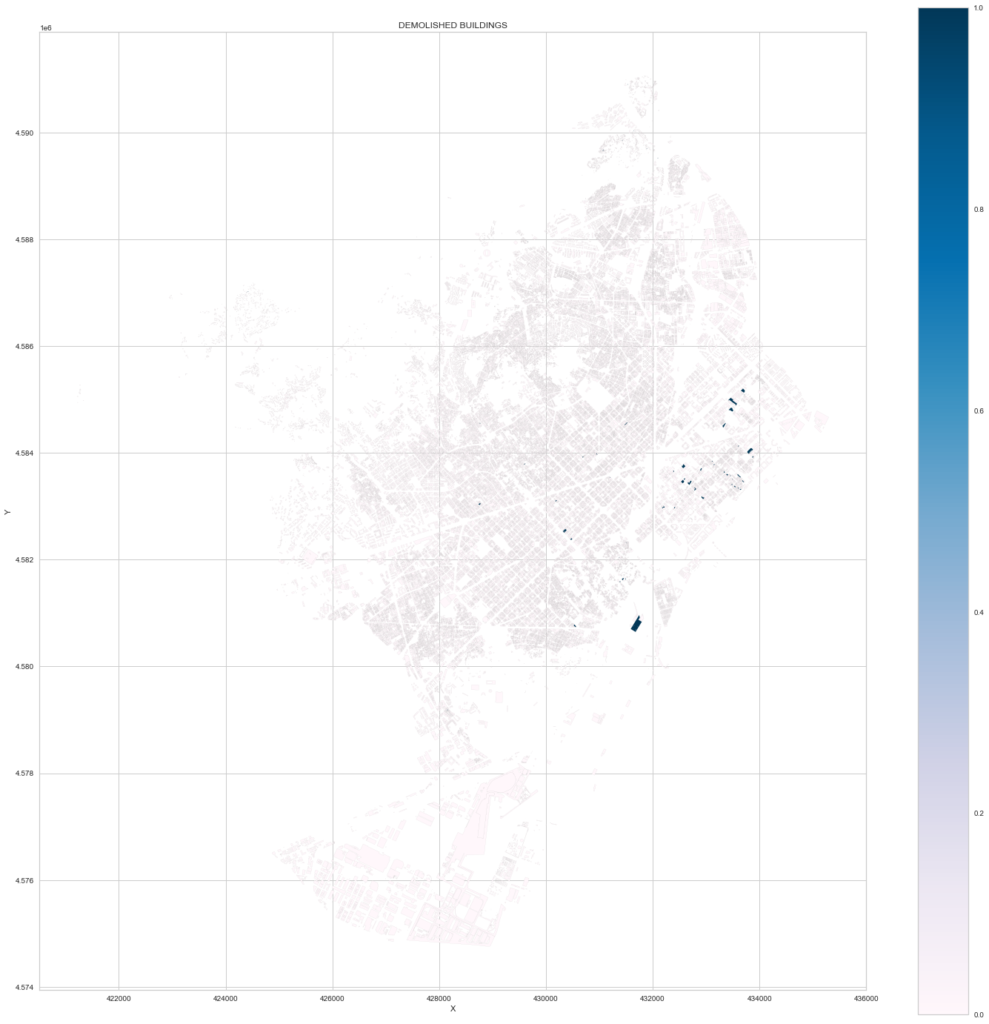
To conduct the analysis, I collected a comprehensive dataset containing information about various attributes of the buildings in Barcelona, such as their condition, usage, energy efficiency, age, and density. I performed classification and clustering tasks using machine-learning techniques to understand the building stock.
In the classification phase, I focused on predicting the condition of buildings based on their attributes. By training and evaluating different classification models, I achieved an impressive accuracy of 95% in predicting the condition of buildings. This enabled me to identify buildings that are likely candidates for renovation or demolition based on their overall condition.
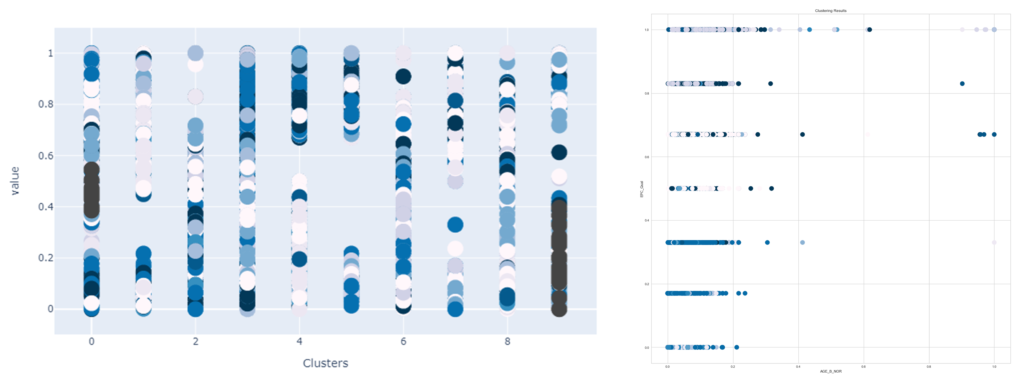
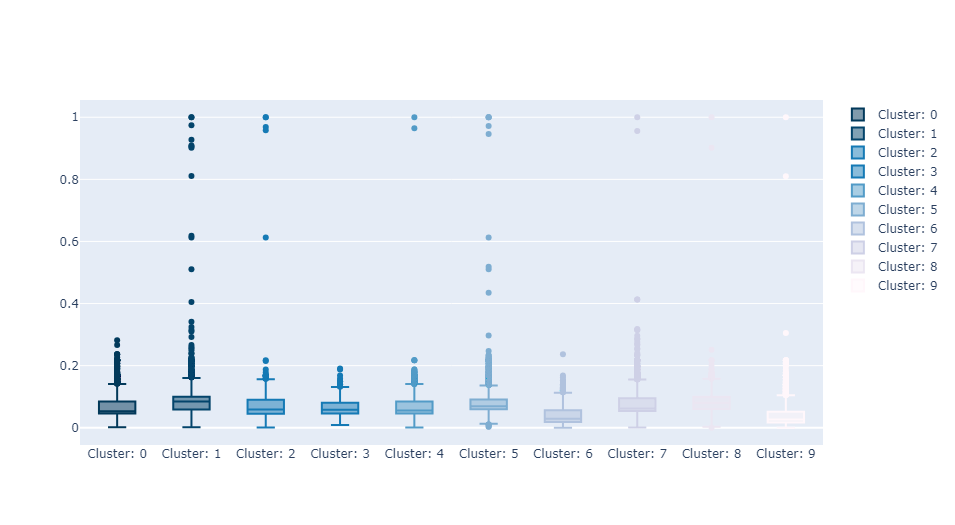
In the clustering phase, I grouped similar buildings based on their attributes. This allowed me to identify clusters of buildings that exhibit similar characteristics and potentially have similar renovation needs. By visualizing the clusters on a map, I gained insights into the spatial distribution of buildings requiring attention. I observed that certain areas in Barcelona have a higher concentration of buildings needing renovation or demolition, highlighting the need for targeted intervention and resource allocation and extraction.
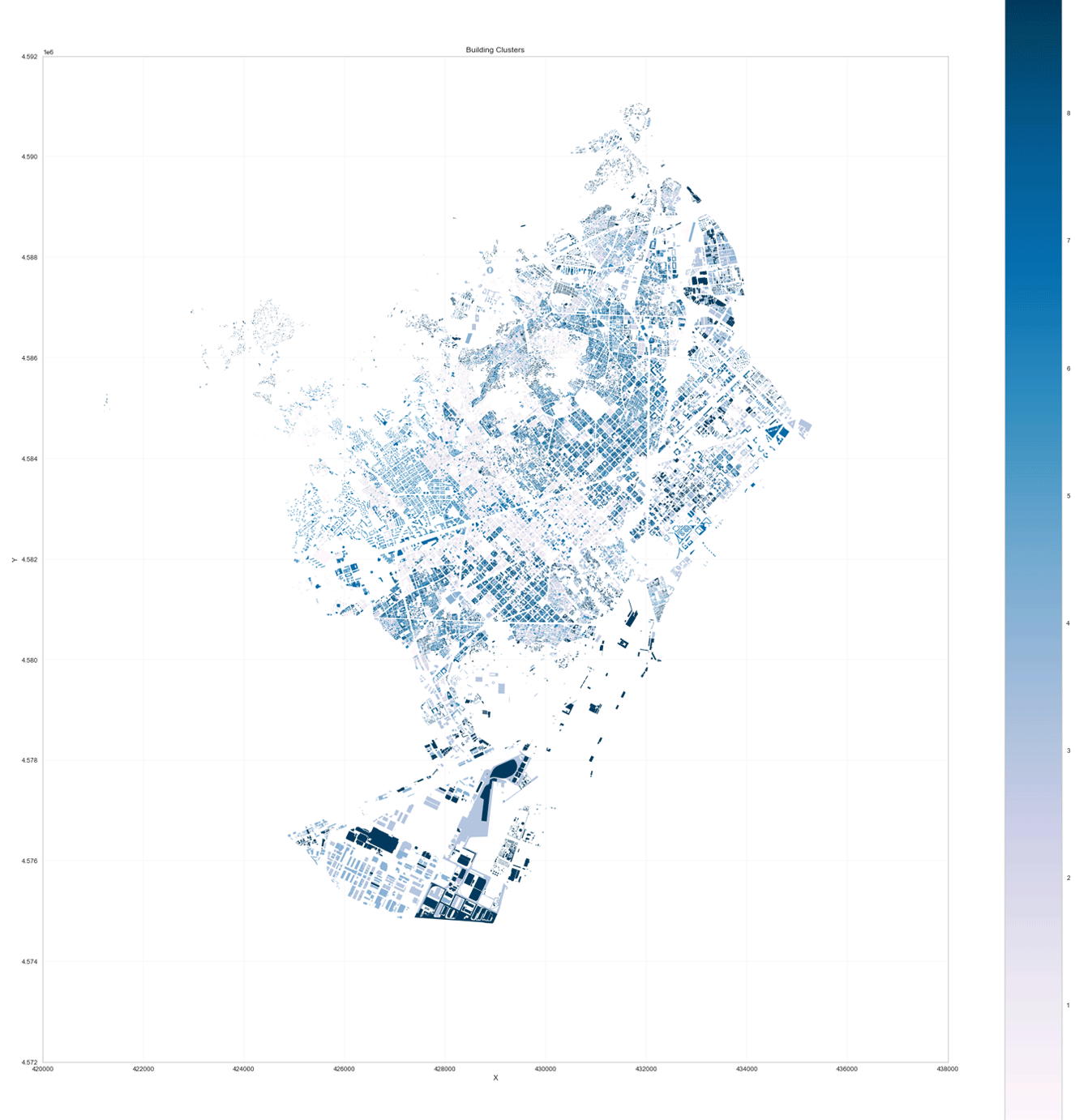
The analysis of the BCN Building Stock dataset provides valuable insights into the potential building stock in Barcelona that requires renovation or demolition. By leveraging machine learning techniques, I was able to accurately classify buildings based on their condition and identify clusters of buildings with similar characteristics. This information can be utilized by urban planners, policymakers, and stakeholders to prioritize renovation efforts, allocate resources efficiently, promote sustainable urban development and circular building practices.
In conclusion, the BCN Building Stock project demonstrates the power of data analysis and machine learning in assessing the condition of the building stock. By identifying buildings needing renovation or demolition, I pave the way for a more sustainable and resilient urban environment in Barcelona. The insights gained from this analysis can guide future decision-making processes and contribute to the overall improvement of the city’s building stock and quality of life for its residents.