Unraveling Footfall Patterns for Enhanced Urban Planning: A Spatial and Temporal Analysis
Introduction
Footfall prediction plays a crucial role in urban planning decision-making processes. Understanding the intricate relationship between footfall and its two dimensions—space and time—is essential. In this project, we delve into the workflow and key findings of a project aimed at comprehending the patterns and interdependencies of footfall, considering both spatial and temporal factors.
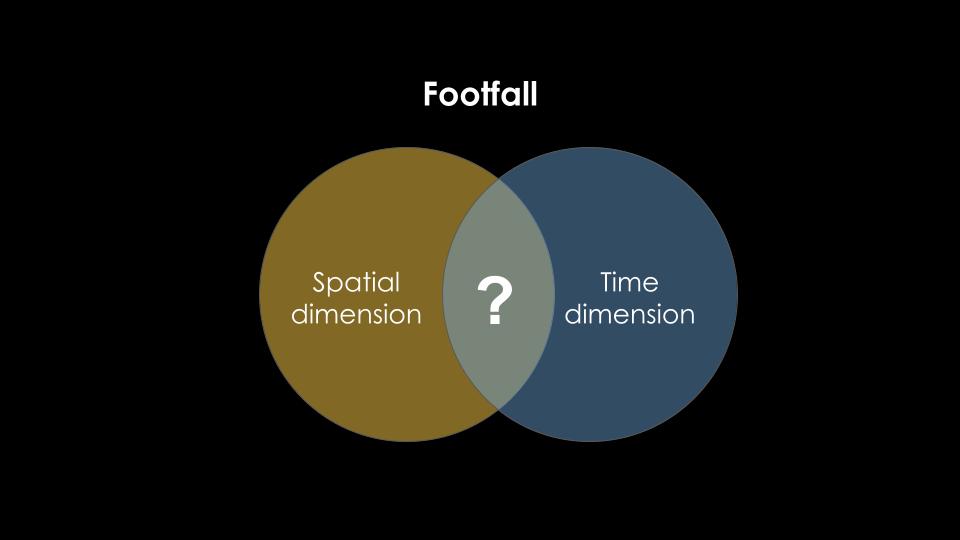
The Workflow Unveiled
The project starts with an extensive data collection effort encompassing temporal and spatial data. The temporal datasets were meticulously analyzed, visualized as histograms, and scrutinized to identify patterns over time. To gain a deeper understanding of the temporal trends, rates of change between different time-based data were calculated and represented in polar plots. Significant intervals of notable change, or peaks in rates of change, were identified for weekdays, Saturdays, and Sundays.
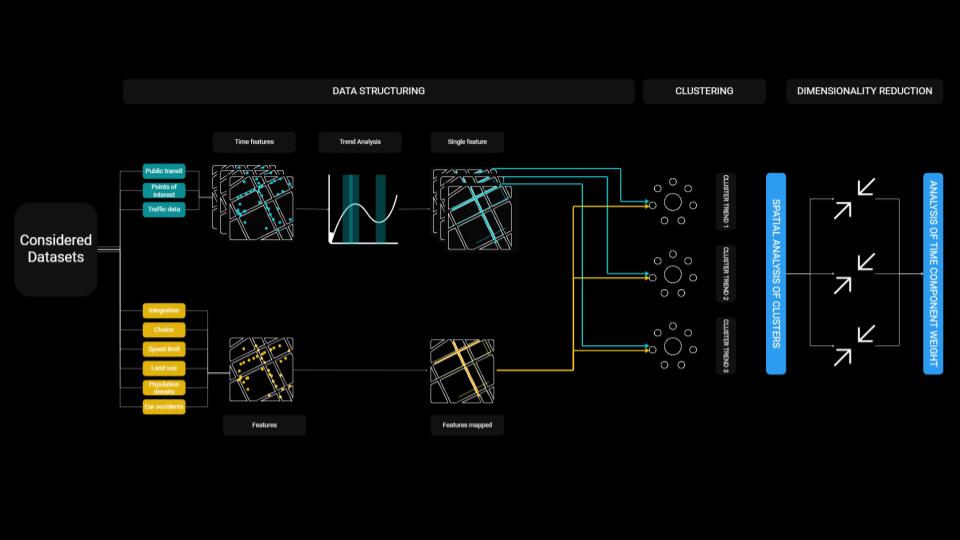
Next, these significant time intervals were separately subjected to a clustering process, one at a time, to compare them with the spatial datasets. This allowed for a comprehensive exploration of the spatial distribution of features and the identification of any disparities in the distribution of time-based features. Three clustering processes were performed for each significant time interval, leading to distinct spatial feature distributions but differing patterns in time-related features.
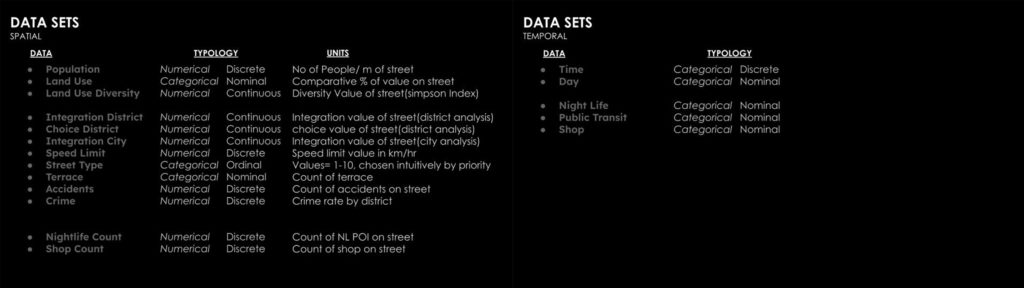
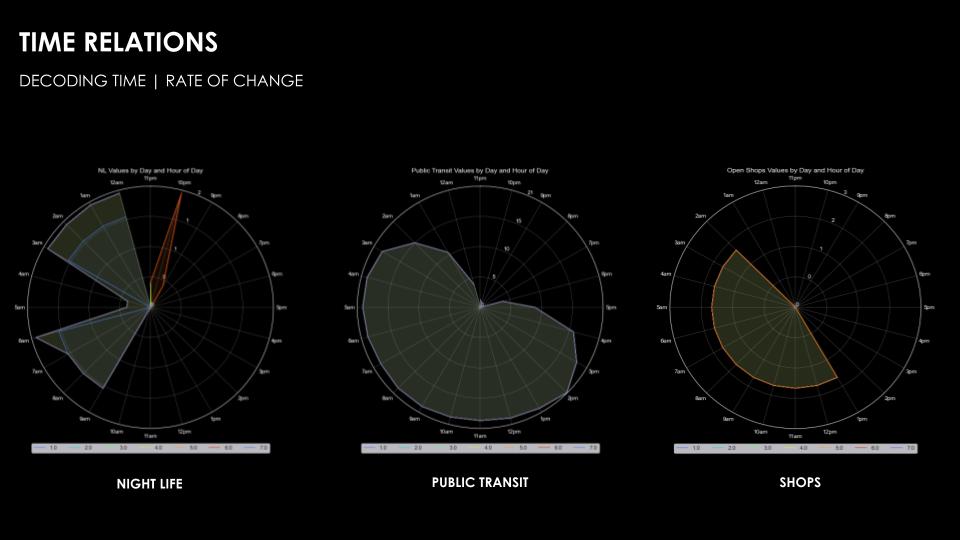
Unveiling the Clusters:
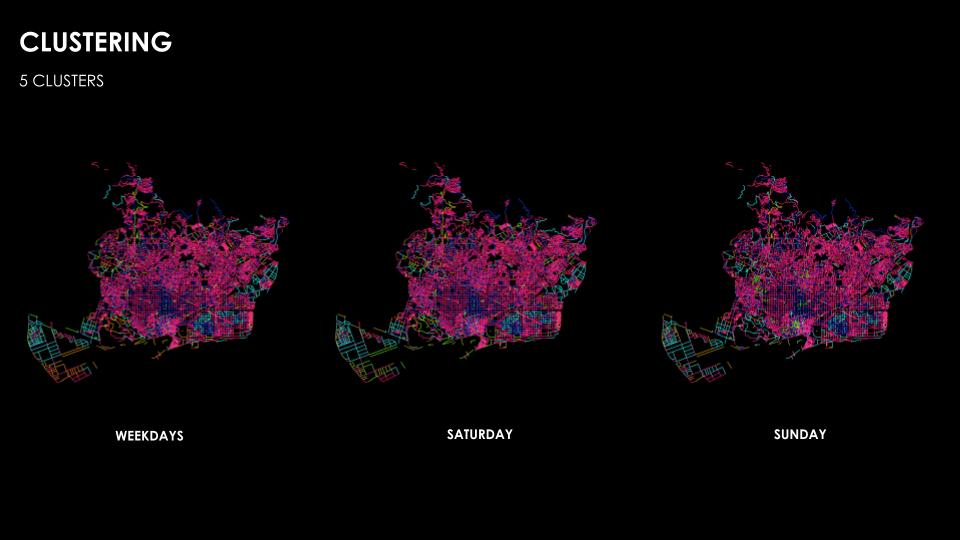
The results of the clustering process revealed intriguing insights. While the spatial distribution of features showed similarities across the three clusters, indicating consistency in footfall patterns in various locations, the distribution of time features varied significantly. This finding underscores the importance of considering both spatial and temporal aspects when predicting footfall accurately. Spatial characteristics alone are insufficient to capture the nuances of footfall dynamics, emphasizing the need for granular and extensive temporal datasets.
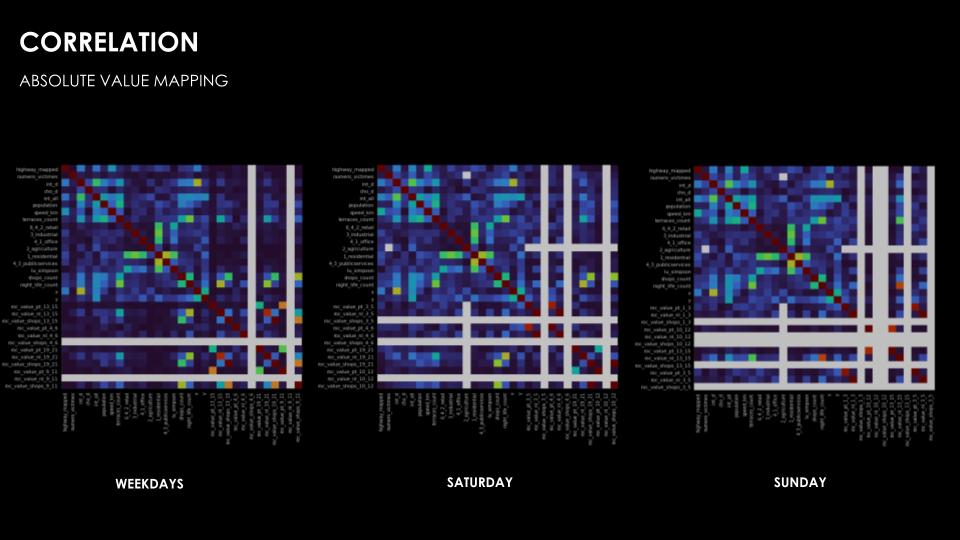
Diminishing Dimensionality:
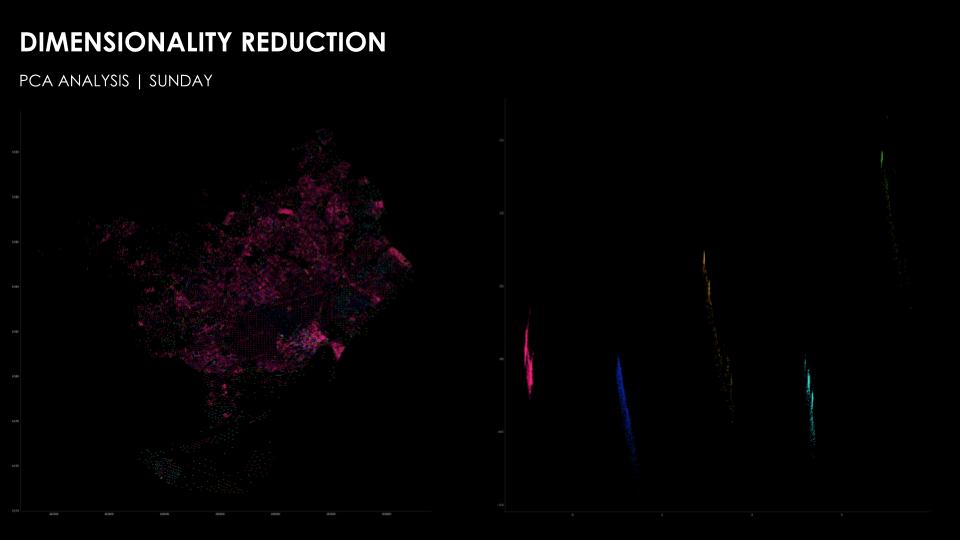
To gain further insights, a dimensionality reduction process was employed, specifically using t-SNE (t-Distributed Stochastic Neighbor Embedding), with different perplexities for each of the three clusters. Surprisingly, the results of all three clusters were strikingly similar, suggesting that the time datasets exerted minimal influence on the footfall prediction process. This observation points to the necessity of augmenting the dataset with more granular and comprehensive time features to achieve more accurate footfall predictions.
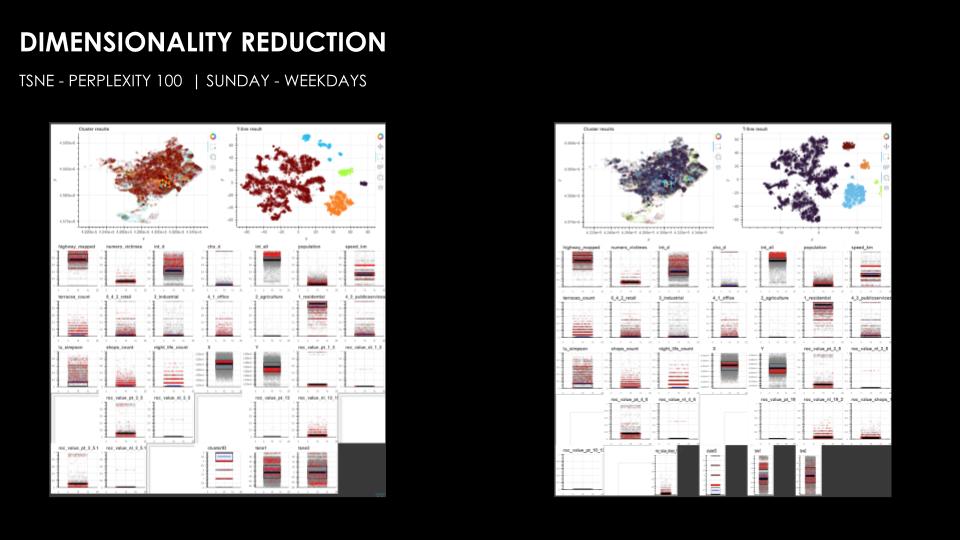
Conclusions and Implications :
This footfall prediction project shows the crucial interplay between space and time in understanding footfall patterns. The findings underscore the significance of incorporating both spatial and temporal dimensions in urban planning decision-making processes. To achieve more reliable footfall predictions, future endeavors must focus on enriching the dataset with more extensive and fine-grained time features. By integrating such insights, urban planners can make informed decisions and shape more effective and optimized urban spaces.
In summary, this project serves as a stepping stone towards a better understanding of footfall dynamics, offering valuable insights into the relationships between space, time, and footfall. By unveiling the intricacies of footfall patterns, we empower urban planners to create vibrant and well-designed urban environments that cater to the needs and behaviors of pedestrians.