Grasshopper’s neural network component is turning data into smart, functional design solutions. The neural network component within this environment presents a new frontier for design intelligence. Here’s a walkthrough of how this component transforms raw data into smart design solutions.
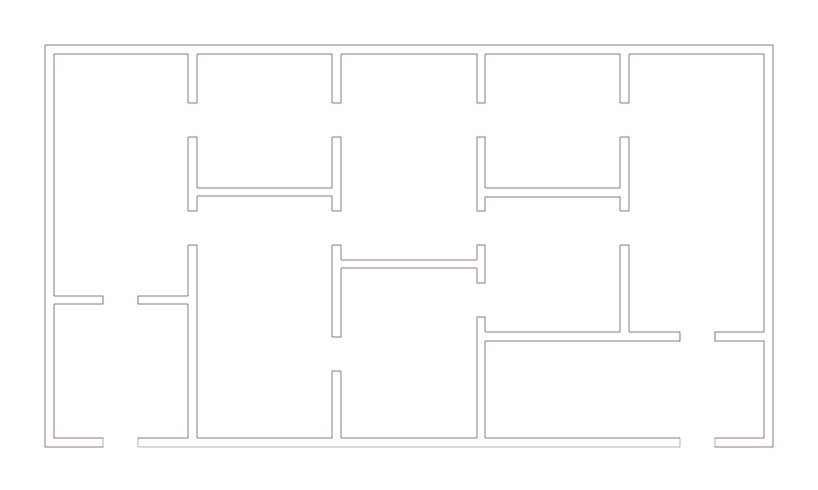
The Training Grounds: Neural Network Trainer
Designers input parameters into the Neural Network Trainer—this is where AI learns to understand design through data. It’s an iterative process, honing the AI’s ability to discern patterns and minimize errors.
At the heart of the operation is the Neural Network Trainer. It’s here that the seeds of intelligence are planted. This component is tasked with feeding data through a neural network, adjusting weights and biases to minimize the error rate. The trainer’s goal is straightforward yet profound: to learn from the data and identify patterns. It iterates repeatedly, each time fine-tuning the network’s parameters to improve the outcome.
Plan Circulation Data:
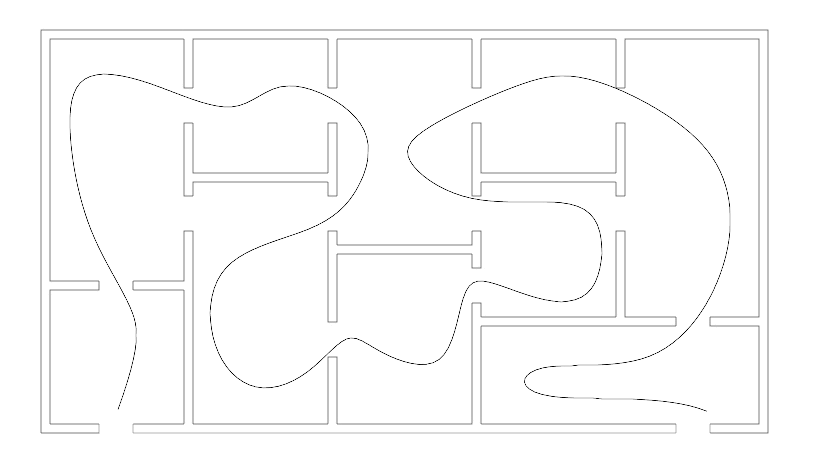
Watching Learning Happen: Quick Graph
Adjacent to the trainer sits a graphical display, modestly titled ‘Quick Graph.’ This visualization is a window into the neural network’s mind, mapping its learning process over time. As the network iterates, the graph updates, showing a curve that represents the error rate. A steep decline in this curve is what designers hope for—an indication that the network is effectively learning and improving its predictions with each iteration.
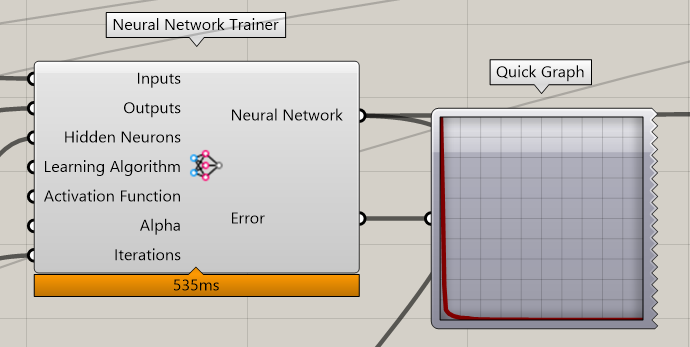
Real-World Testing Post-training, the Neural Network Tester evaluates the AI’s knowledge. It checks if the AI’s output aligns with real-world design expectations, ensuring practicality meets precision.
From Learning to Application: Neural Network Tester
Once trained, the neural network is put to the test. The Neural Network Tester component is the proving ground, where inputs are fed into the trained model to see how it performs. This is where the theoretical meets the practical. If the training is successful, the tester will provide outputs that align with the designer’s expectations. Otherwise, it’s back to the drawing board, tweaking the network structure or training data to refine the result.
Precision Meets Practicality The outputs are fine-tuned with ‘Round To Nearest’—this component ensures the AI’s solutions are practical for real-world application, rounding off to the nearest feasible value.
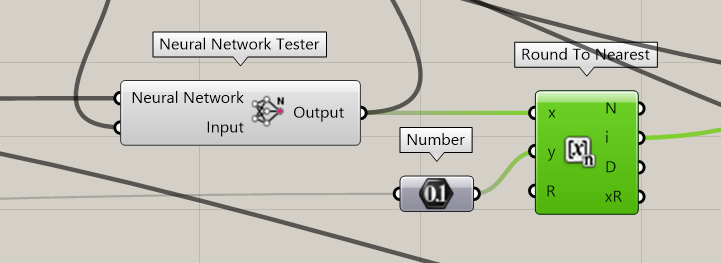
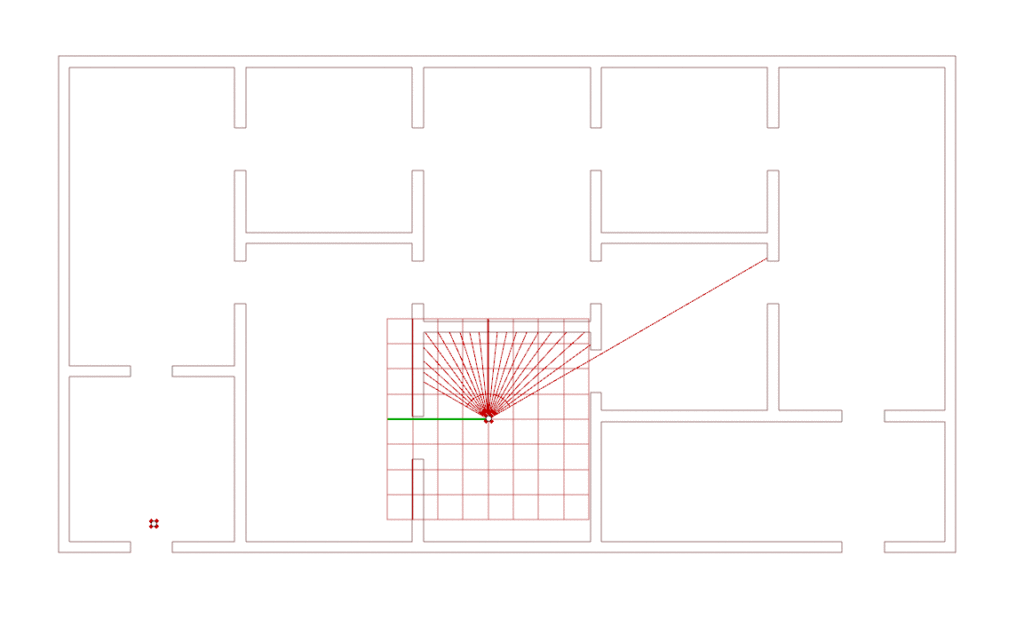
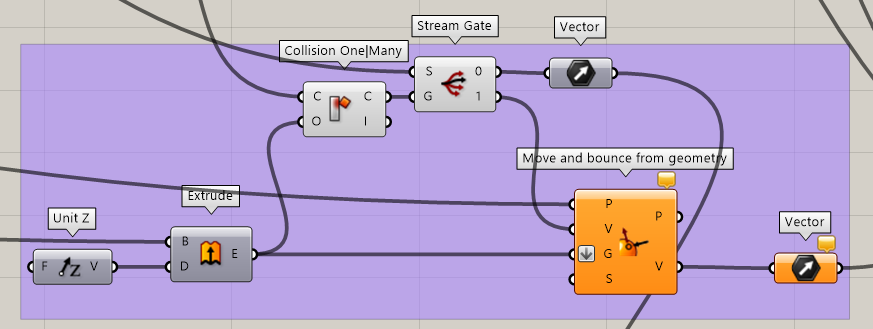
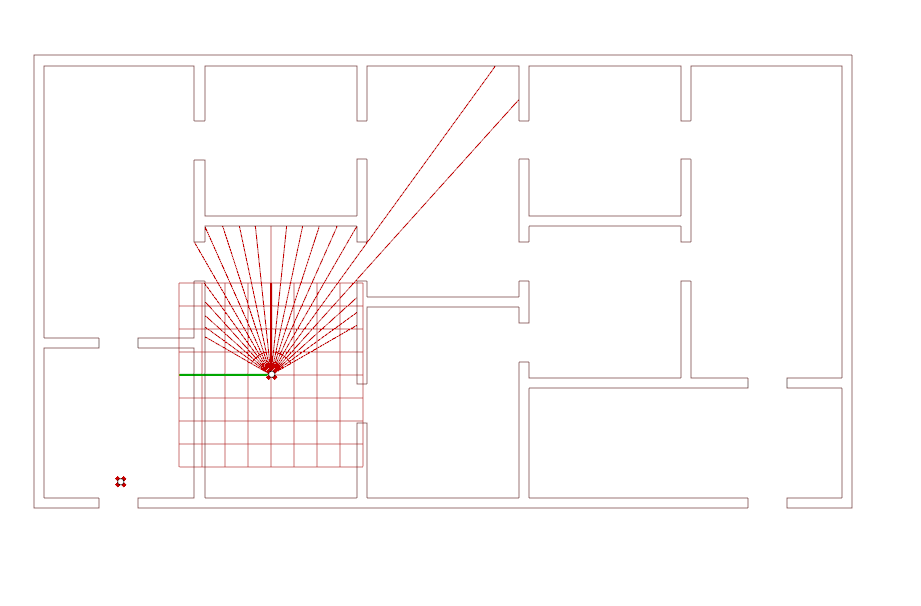
Tolerance Checks: Equality Within Tolerance
Finally, we have a gatekeeper—the ‘Equality Within Tolerance’ component. This function checks whether the results are within an acceptable range. It’s a final quality check, ensuring that the outputs meet the specified criteria and tolerances set by the designer. If the results are too far off, it’s an indicator that the model or the data needs revaluation.
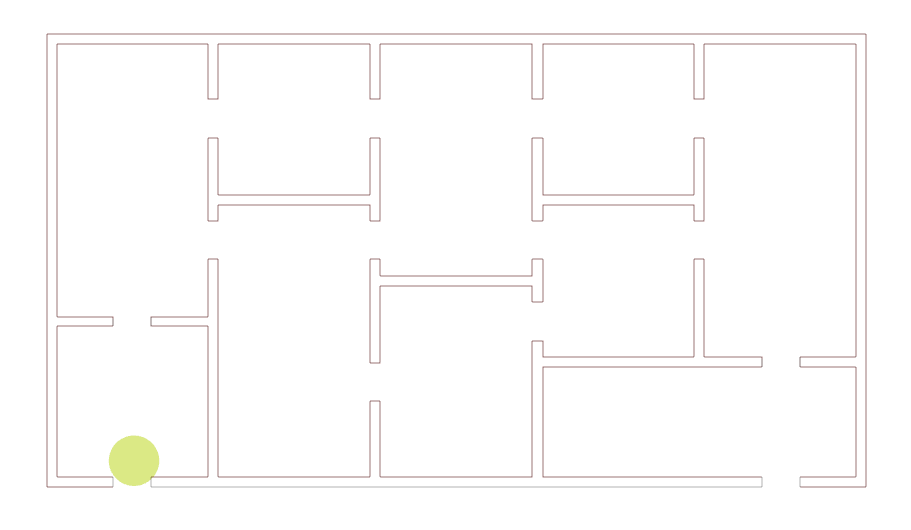
In Conclusion
Grasshopper’s neural network component is more than a tool—it’s a design partner that harnesses the power of machine learning to enhance creativity and precision. As we integrate AI more deeply into our design processes, we unlock the potential to create not just buildings, but environments that learn, adapt, and respond to their inhabitants. The future of design is not just about the structures we create; it’s about the intelligence we embed within them.