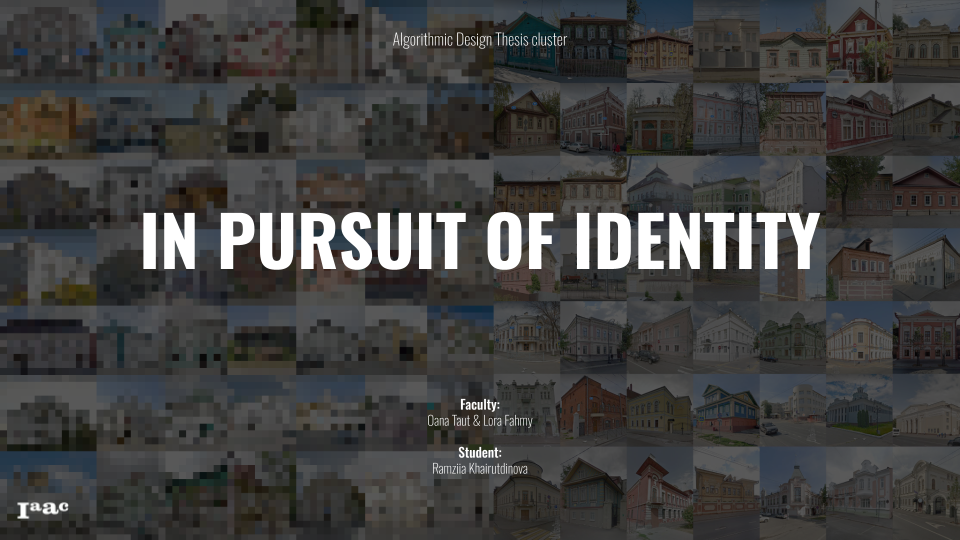
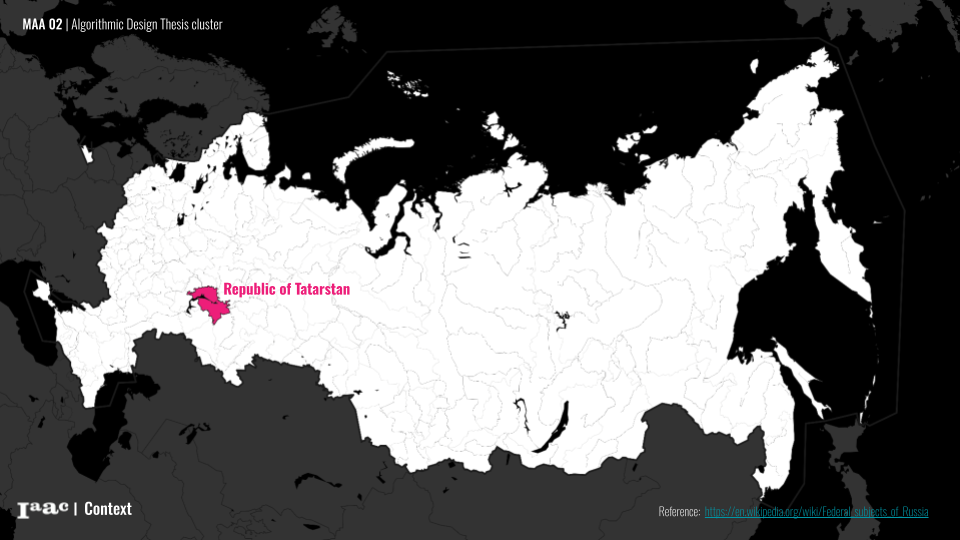
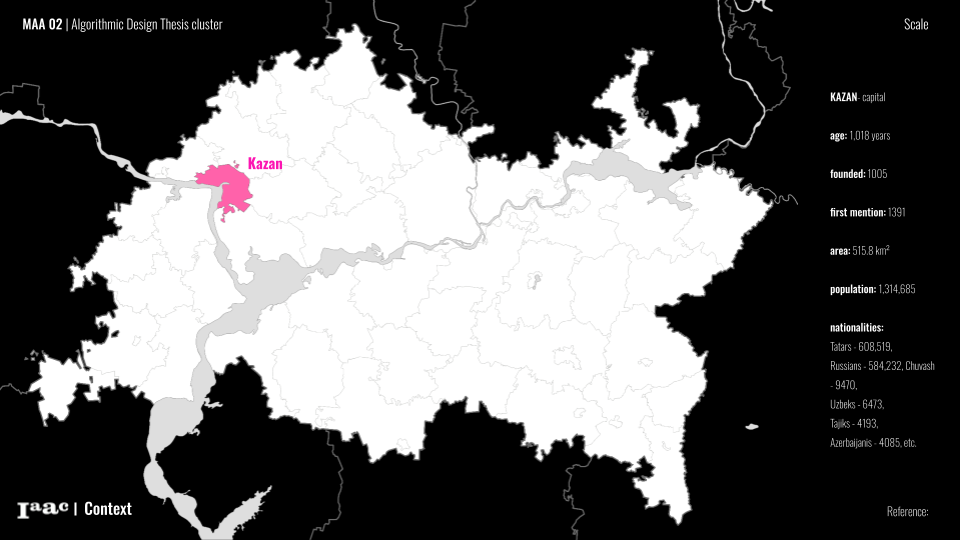
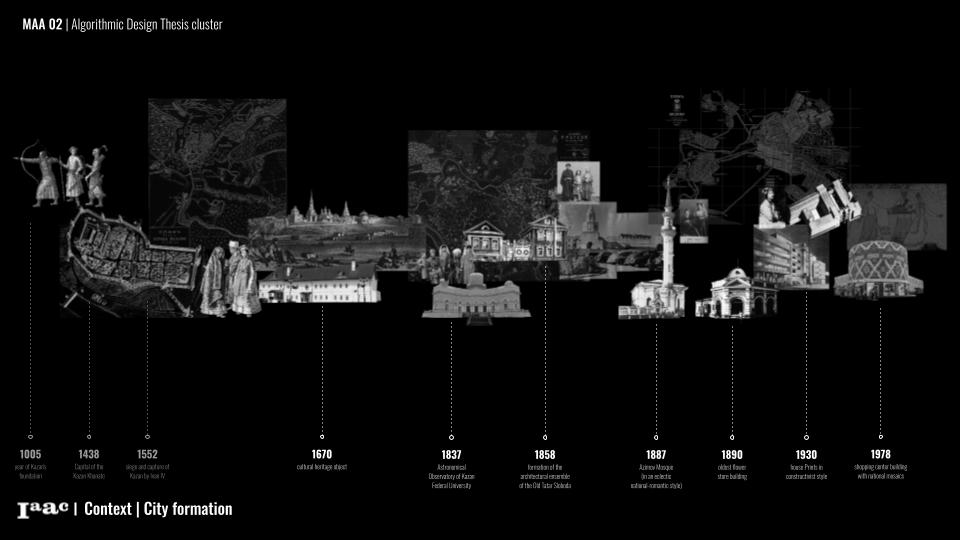
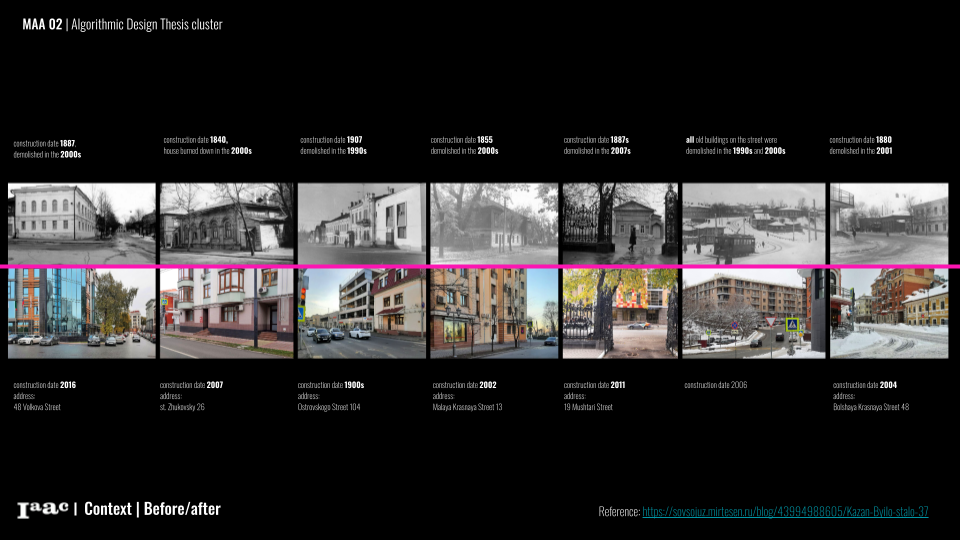
Looking at these before-after photos the first word that comes to mind is fragility: the fragility of its former identity, the frugality of a particular type of life, the fragility of the built environment. Through demolishing and constructing new buildings we are endowing the place with new meanings and new ways of relating to the environment.
There is an opinion that all identities are basically fictional. And if we were to look at things from this perspective, it’s true: an identity can be defined as being an artificial or even random set of values that guide a particular community. Therefore, even if we accept that all identities are manufactured, we also have to accept that they are custom-made for a specific context.
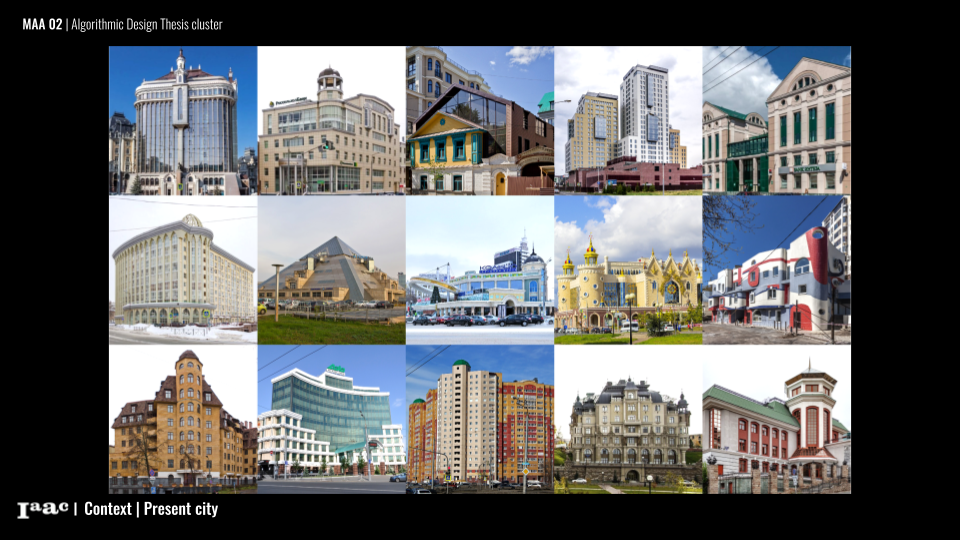
Here we have some examples of modern architecture of Kazan city, which were built in the early 2000-s : For instance, a concert hall in the shape of a pyramid, theater as a sand castle, glass bursting out of ancient ruins and local Gaudi. Globalization blurs the boundaries of identity.
Living in a multicultural environment involves bringing several cultural contexts together in the same space. And instead of designing an identity space for a certain community or culture, the designers all over the world are creating spaces which can become the background for any type of activity or cultural behavior.
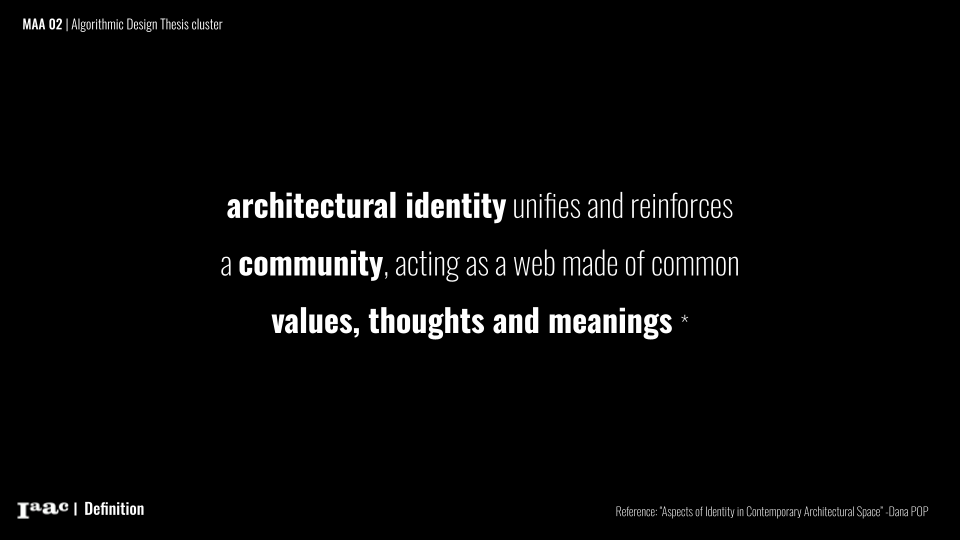
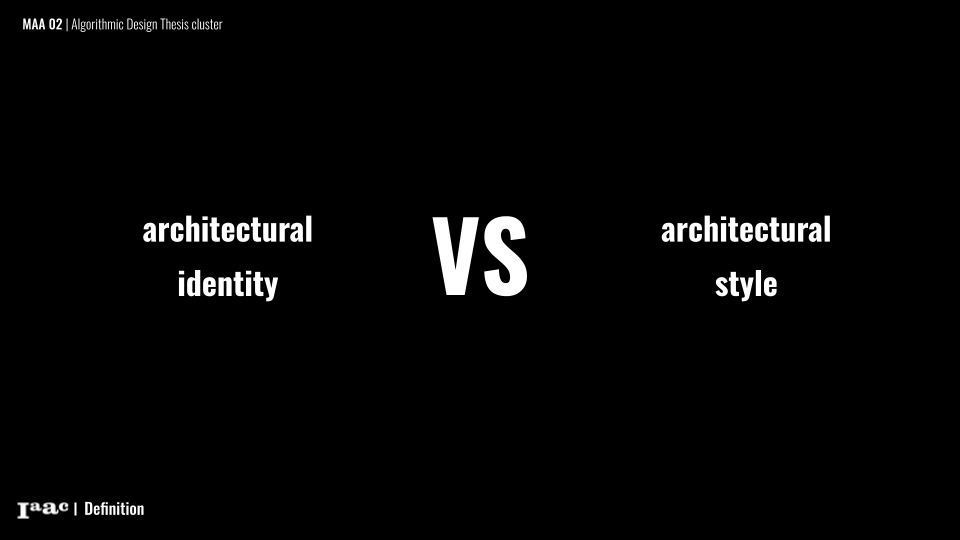
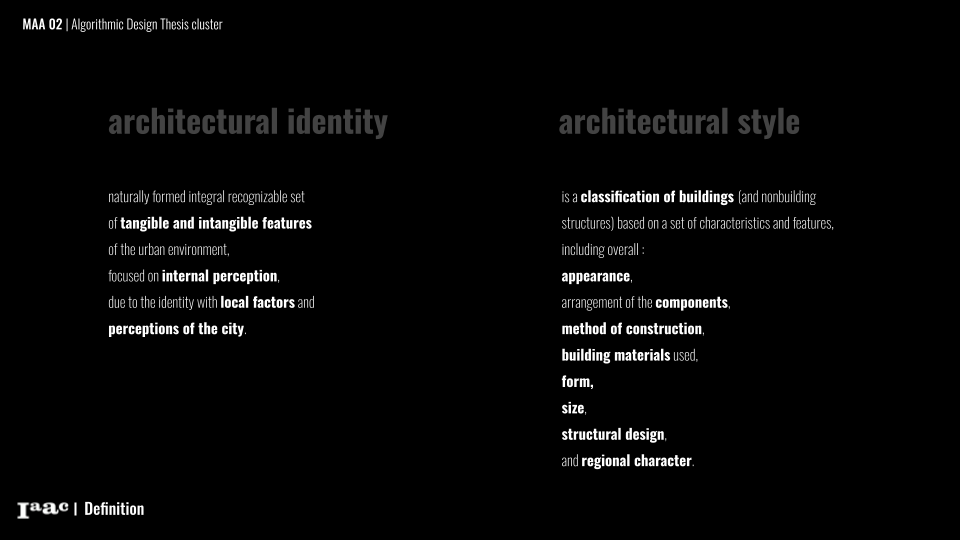
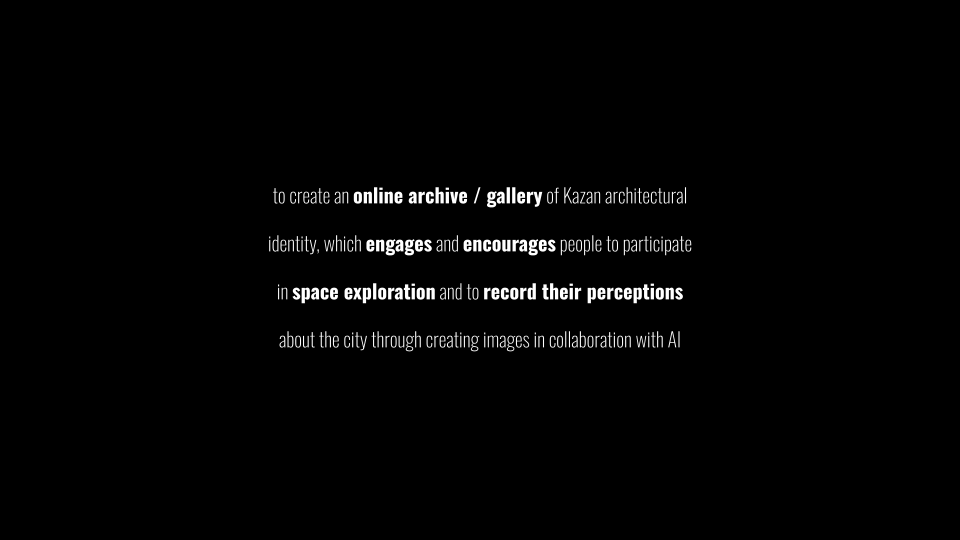
Over the next few slides, I’m going to focus on a few projects with similar ideas to mine, but not exactly.
First one explores and compares outputs of textual and visual information in capturing the place identity of 31 global cities. The findings indicate that generative AI models have the potential to capture the collective image of cities that can make them distinguishable. However, it works only with texts and images, without involving 3d.
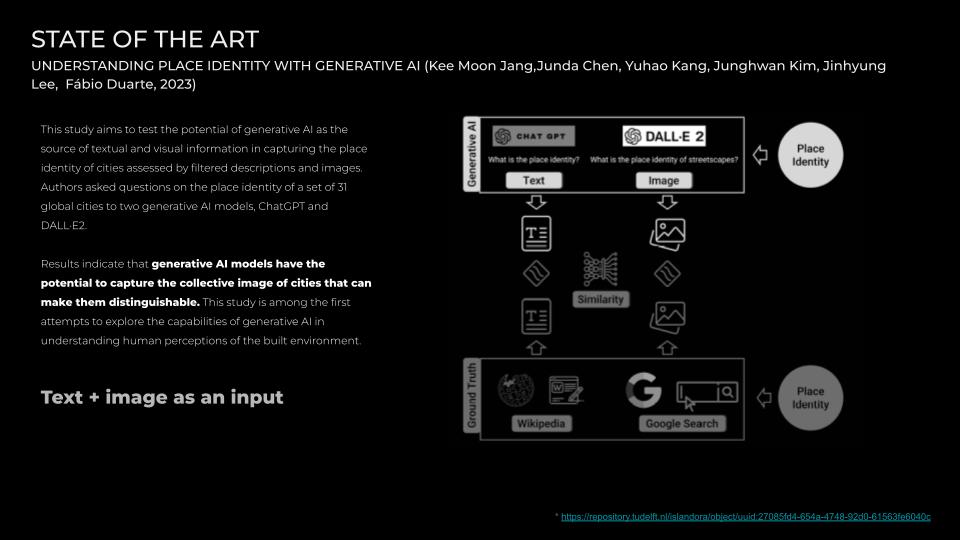
The second project describes a method for generation of building massings and building group layouts, by fusing high accuracy three-dimensional building models with site context derived from cadastral and topographic data, sourced from openly available datasets in the Netherlands. This project is very useful in terms of detailed explanation of workflow and methodology, but it is not aimed to explore city identity and develop this concept, which can bring another level of discussion.
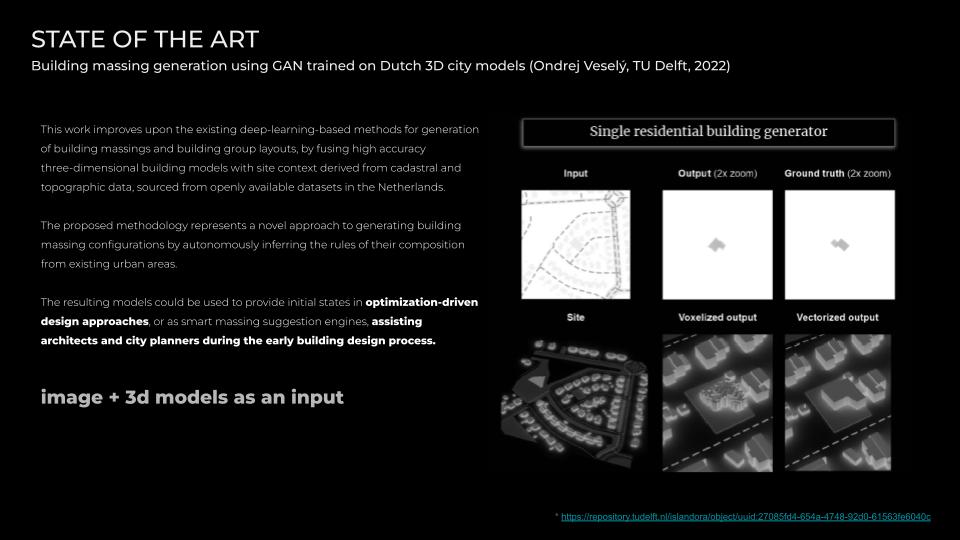
Next project is from last year’s thesis projects, from MaCAD, which initially inspired me to explore 3d generative adversarial networks as an approach to explore architecture and deconstruct the shape itself.
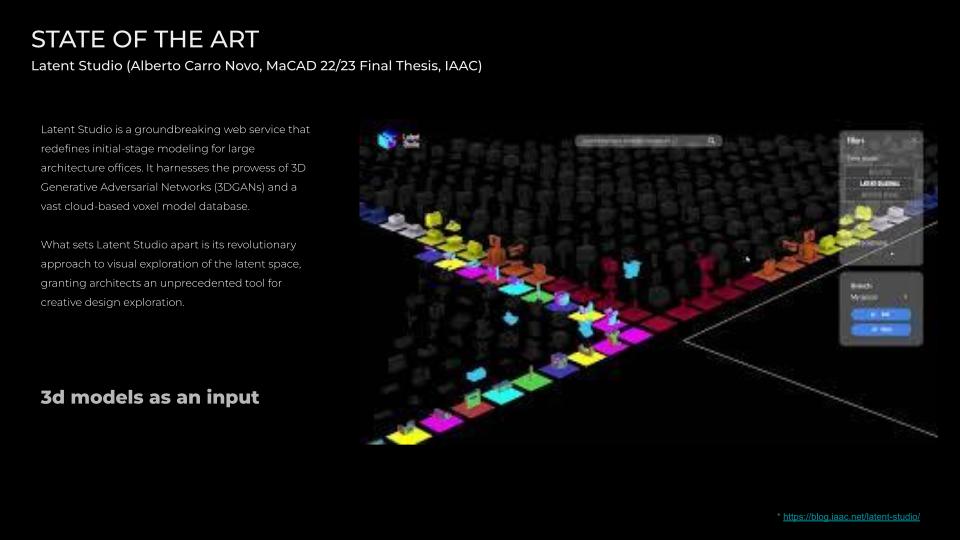
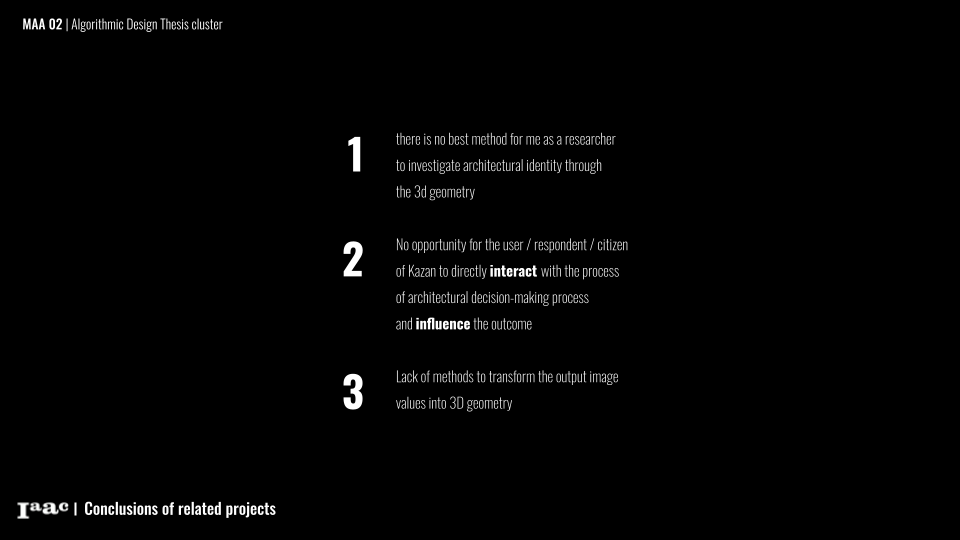
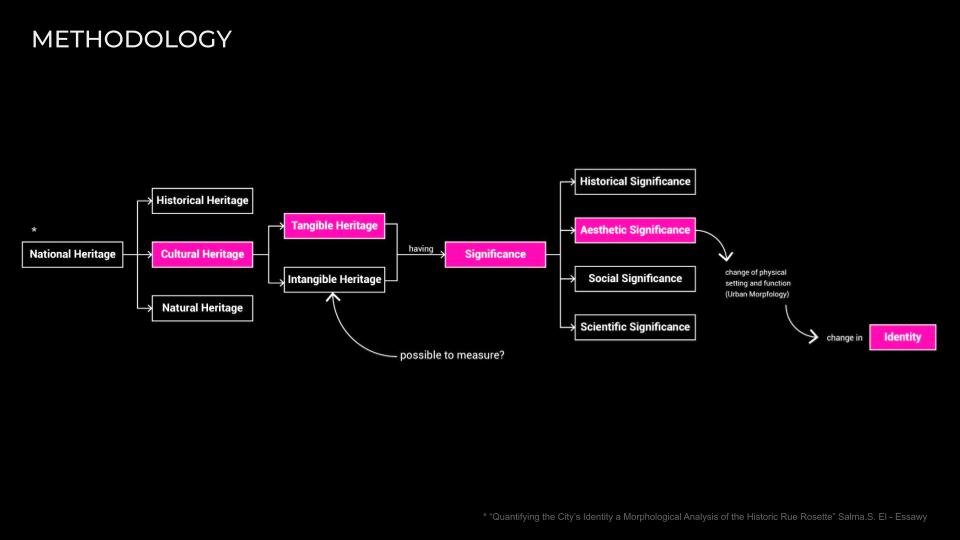
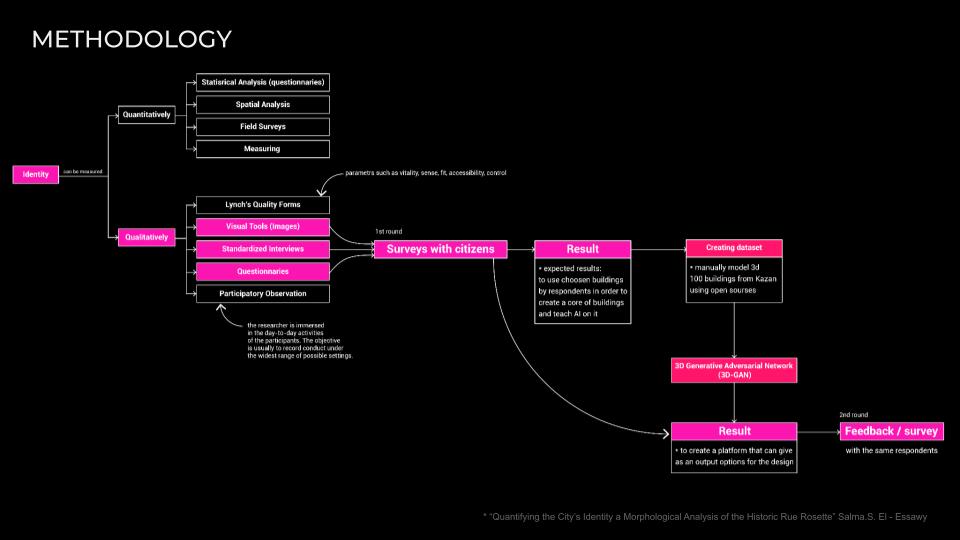
The qualitative method includes questionnaires and interviews with the target group. In the case of research on architectural identity and the efficiency of the product being created. The main purpose of the survey is to validate a problem and to connect with the community in order to understand how they feel about the topic.
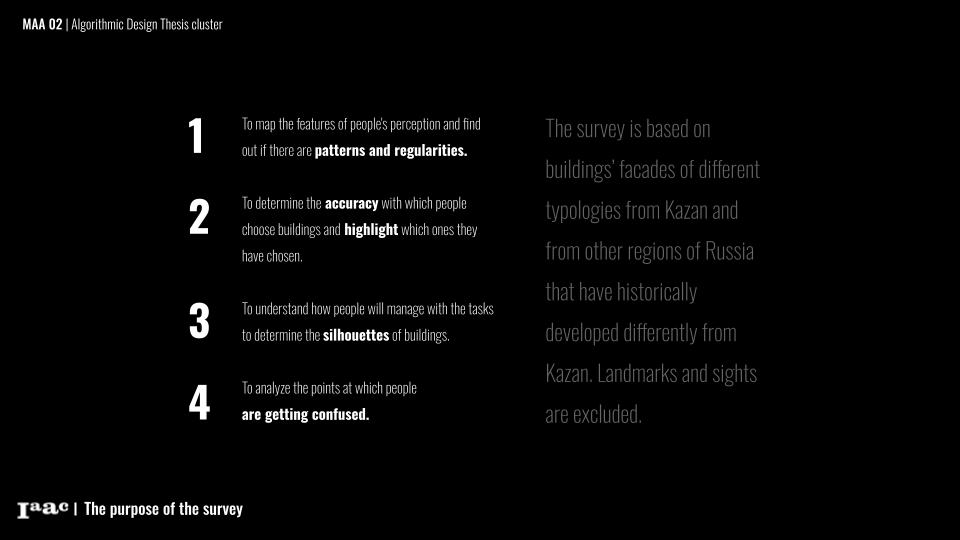
Who are the people who participated in the survey? The majority are from the telegram chat with 6000 subscribers, who are interested in architecture in Kazan, discuss the problems and news. There are architects, professors, researchers, and very curious people. The survey was attended by 250 people. I started from questions about the presence of identity in general and most of the people agree that it exists but expressed not very strong.
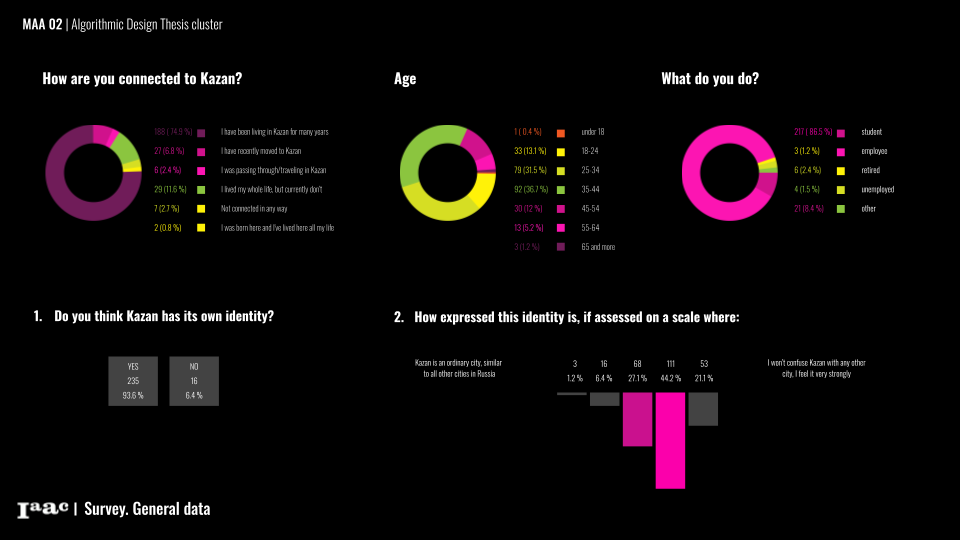
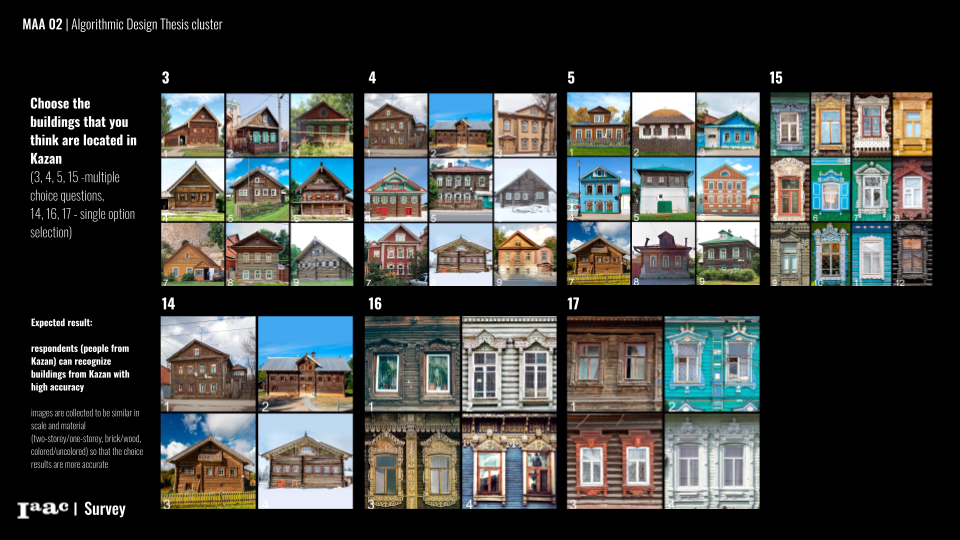
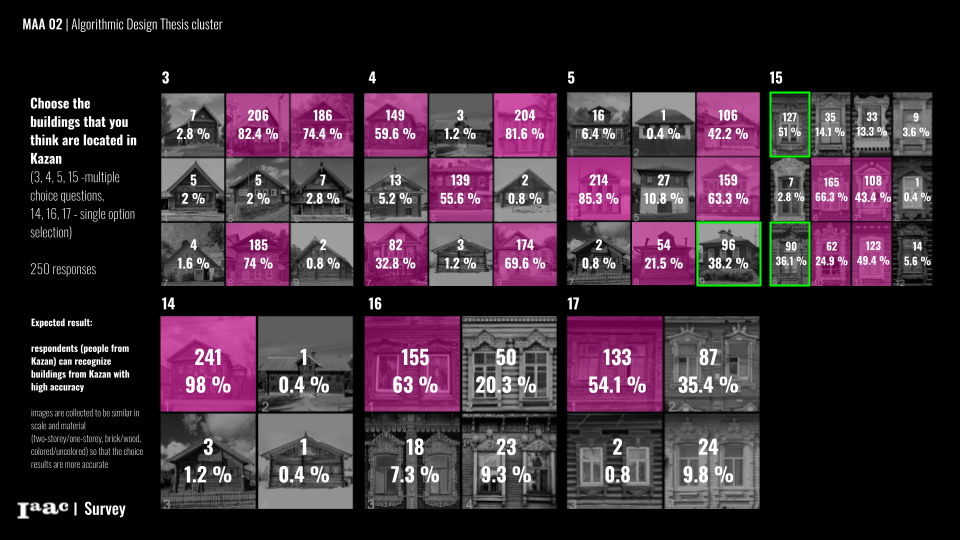
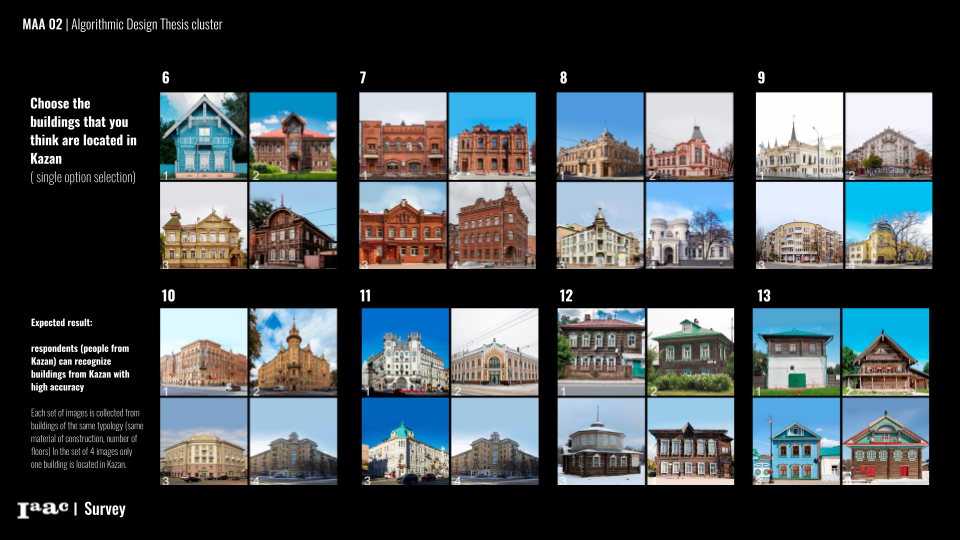
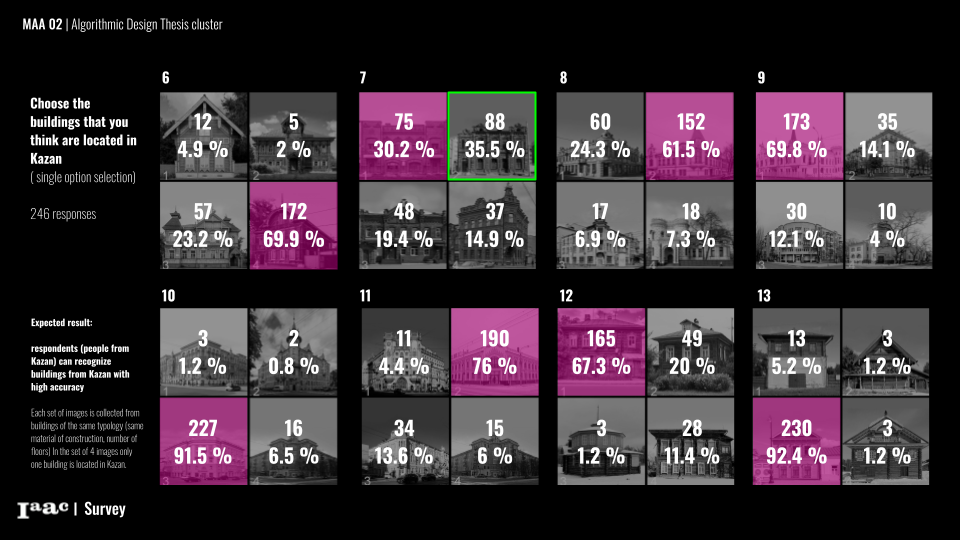
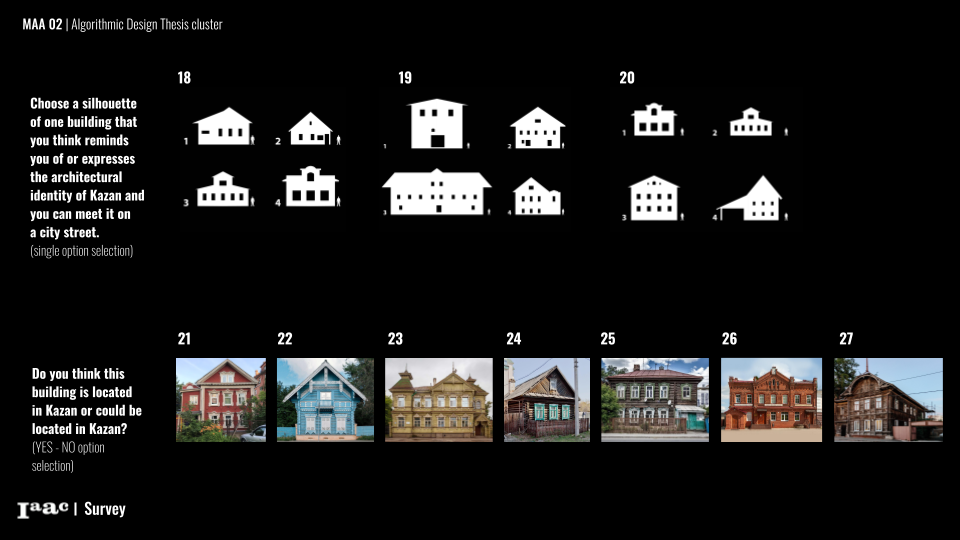
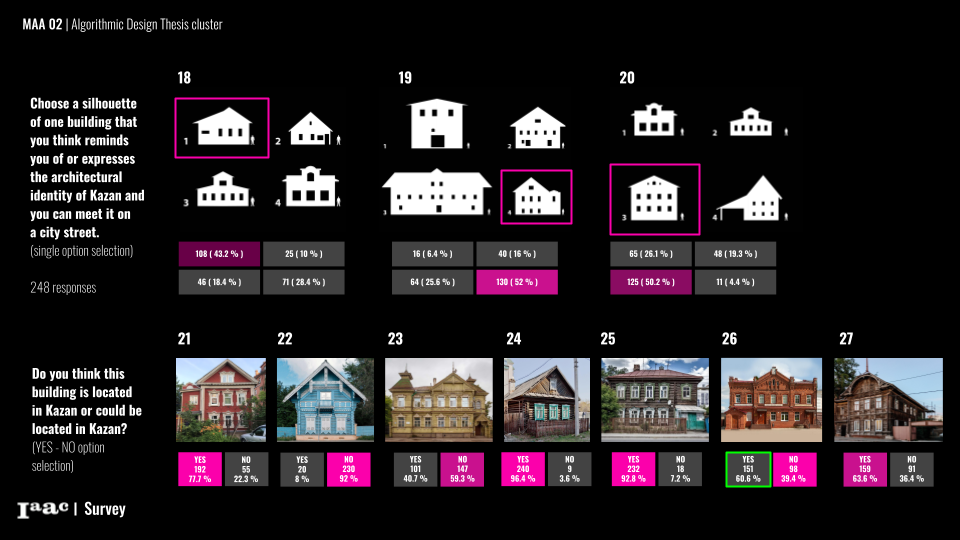
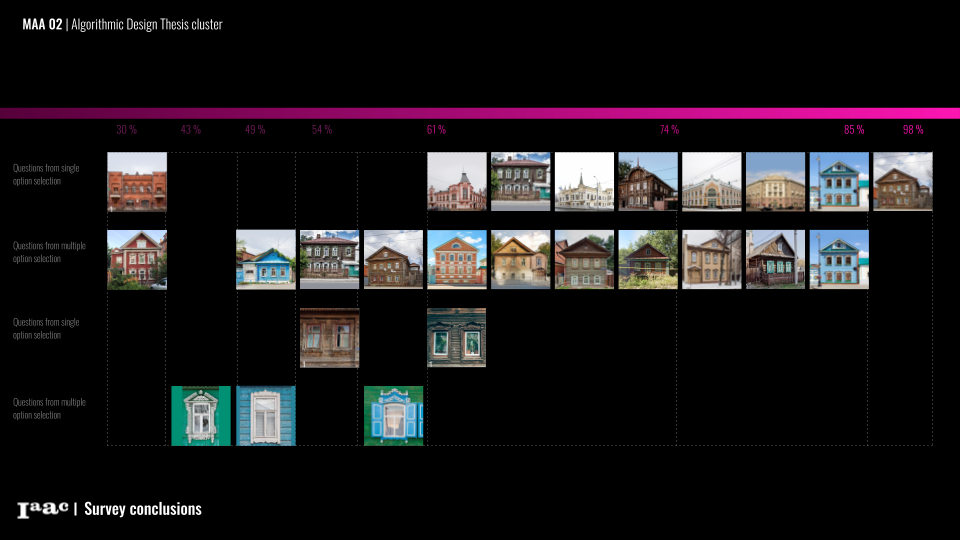
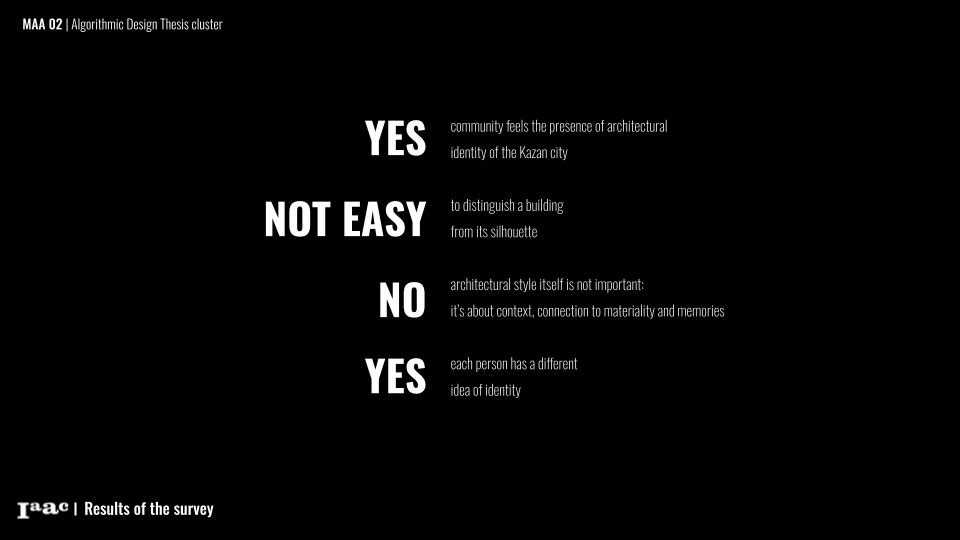
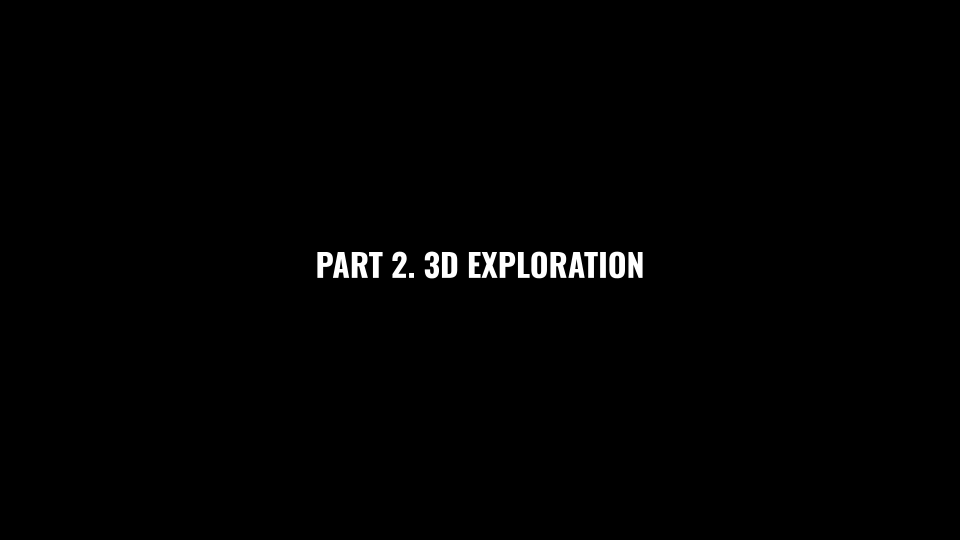
For the tool I chose 3d generative adversarial networks, it is a leading deep generative model that uses deep neural networks (DNNs) to train on a set of training examples to generate new design instances with a degree of flexibility and accuracy that is superior to competing generative approaches.
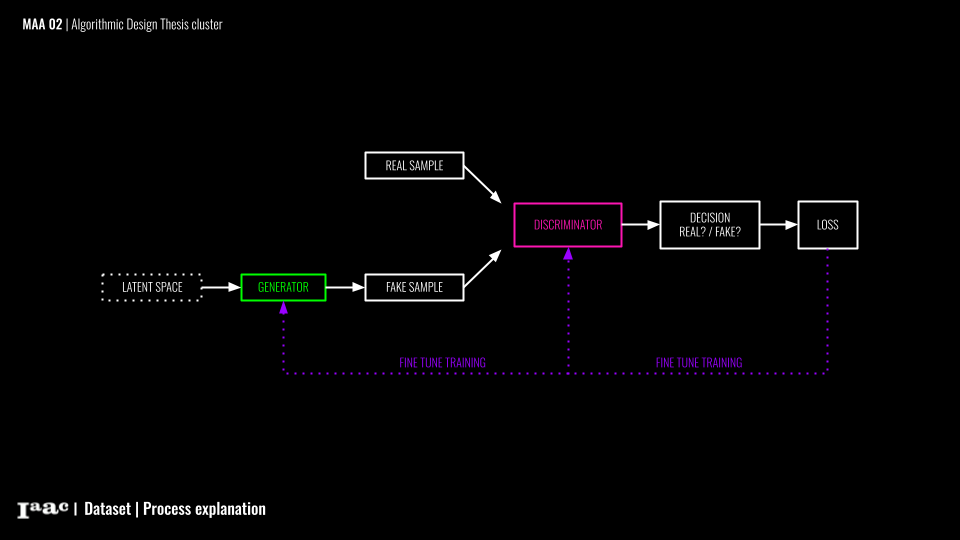
For creating a dataset I have selected a buildings from 2 official documents: “a list with objects of protection of the historical settlement of Kazan”, which includes historically valuable city-forming objects in the amount of 431 buildings and a list of Objects of cultural heritage REPUBLIC OF TATARSTAN which contains 412 buildings. Because there is no open source with 3d models of the buildings in Russia in general and Tatarstan specifically, there’s no opportunity just to connect 3d models into the script. I had to create all 100 of 3d models manually based on maps and different open source data.
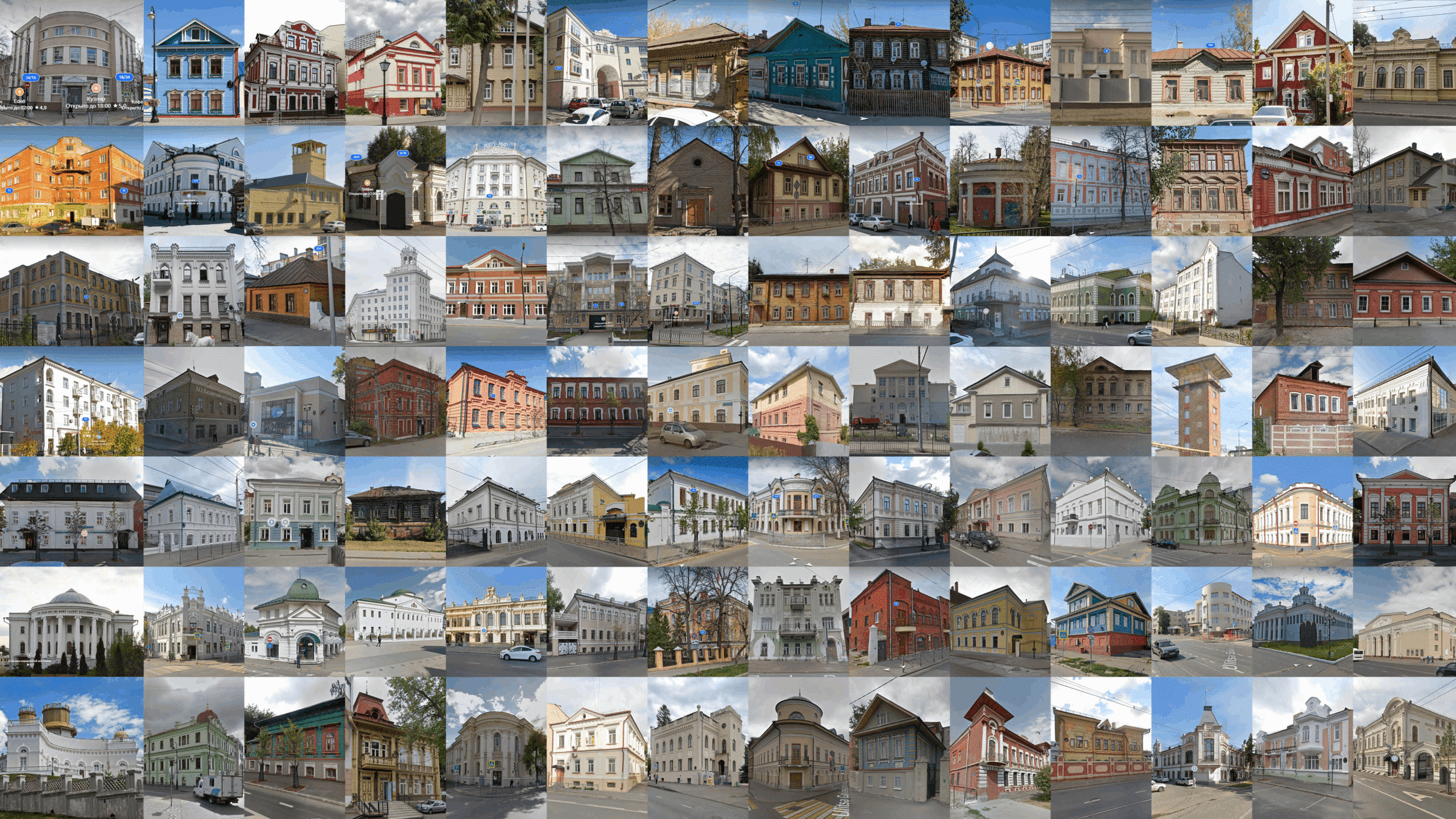
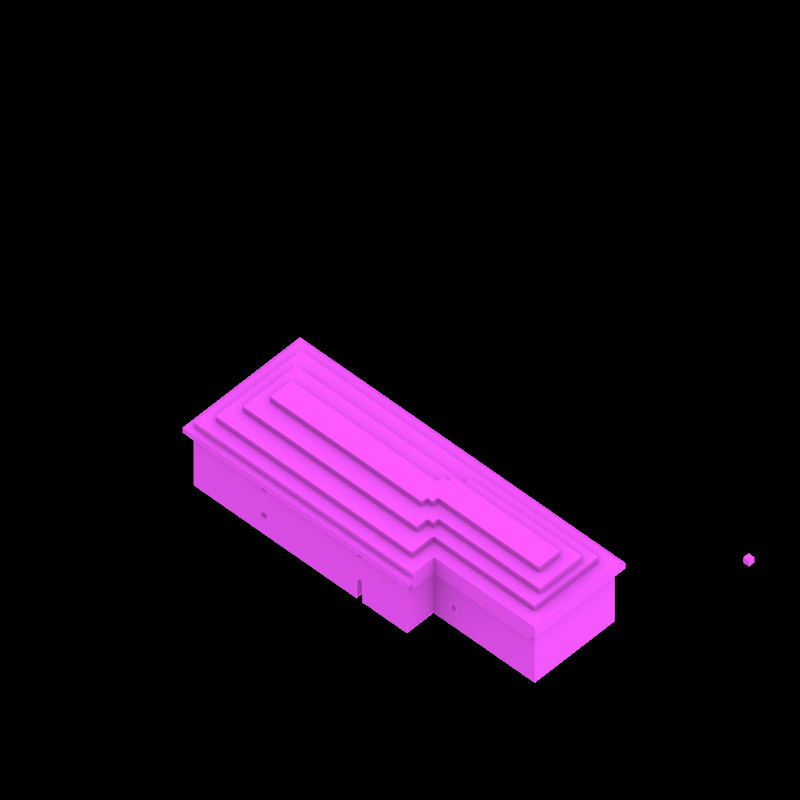
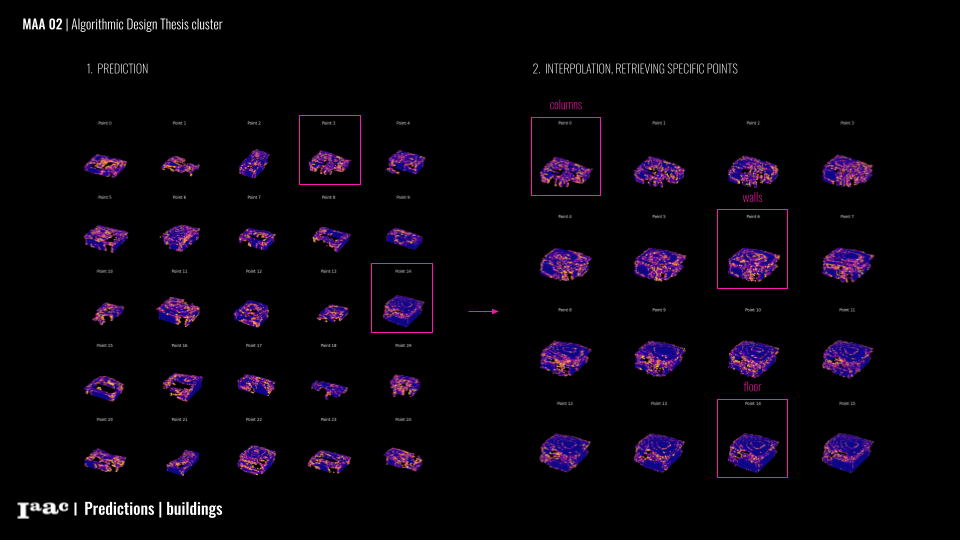
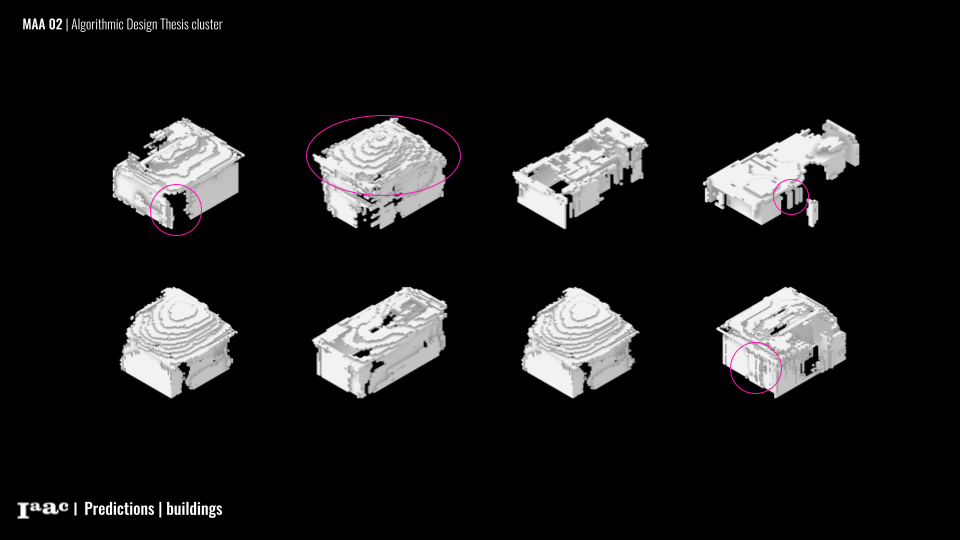
The process of training needs more training and more experimentation with iteration between points, but I was happy to see that I got something that resembles an inclined roof, holes and openings like windows and columns.
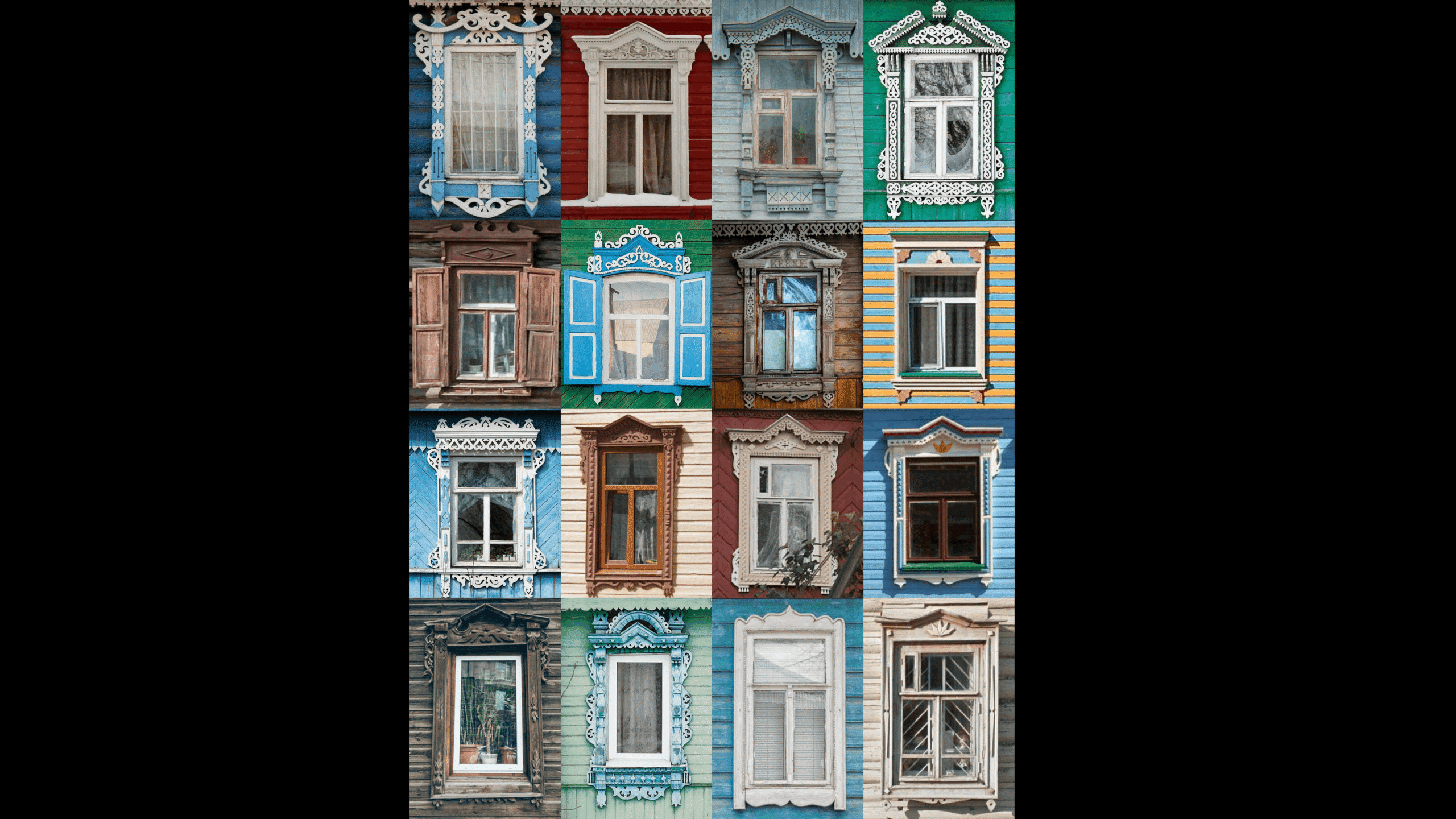
In the survey there were questions about differentiating window frames. Another round experimentation was with wooden window frames, which play a significant role in the formation of the architectural image of the building and distinguish buildings from different regions of Russia through ornamentation.
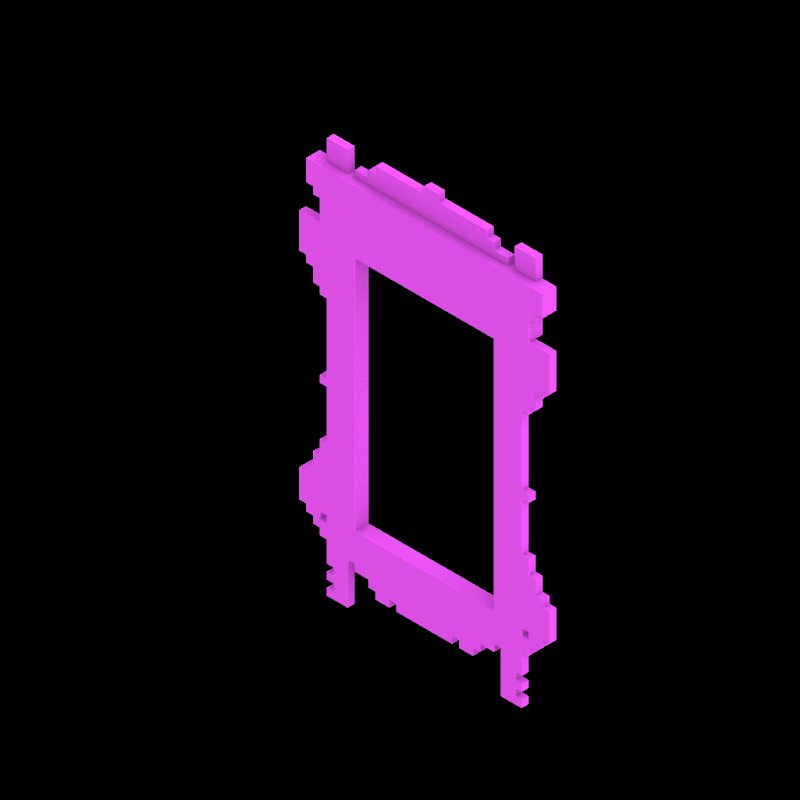
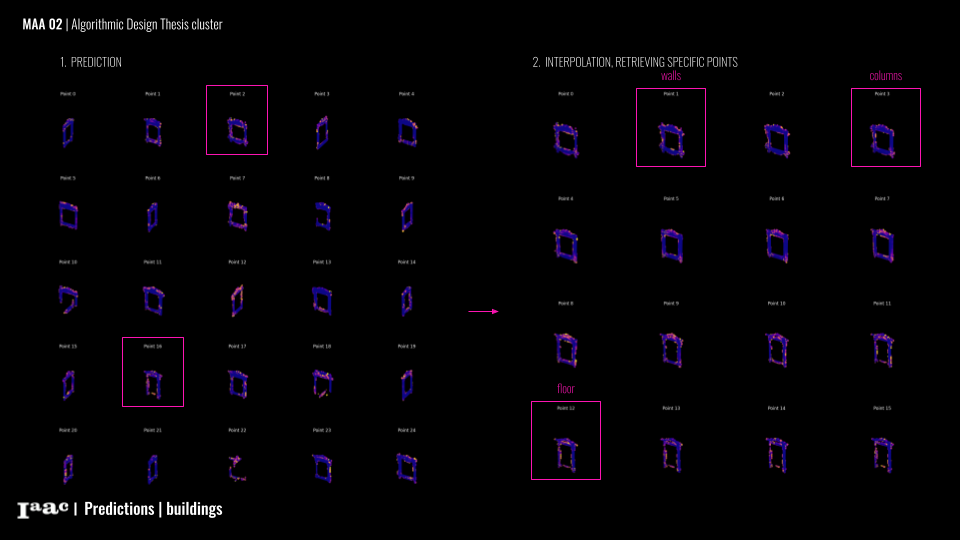
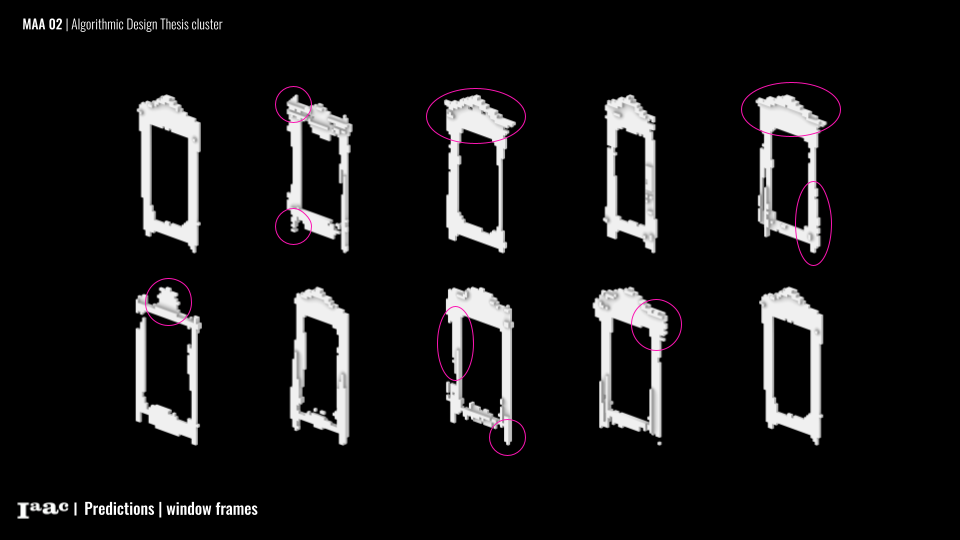
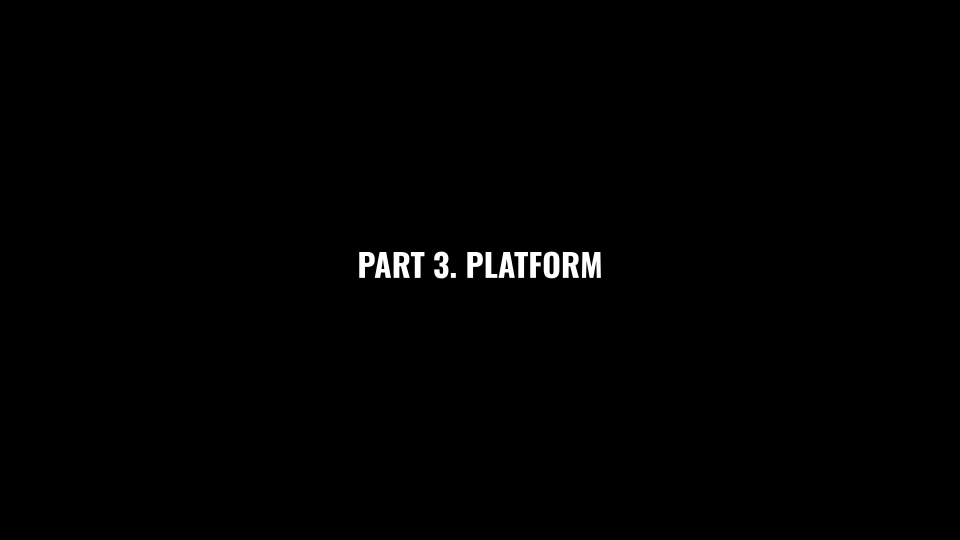
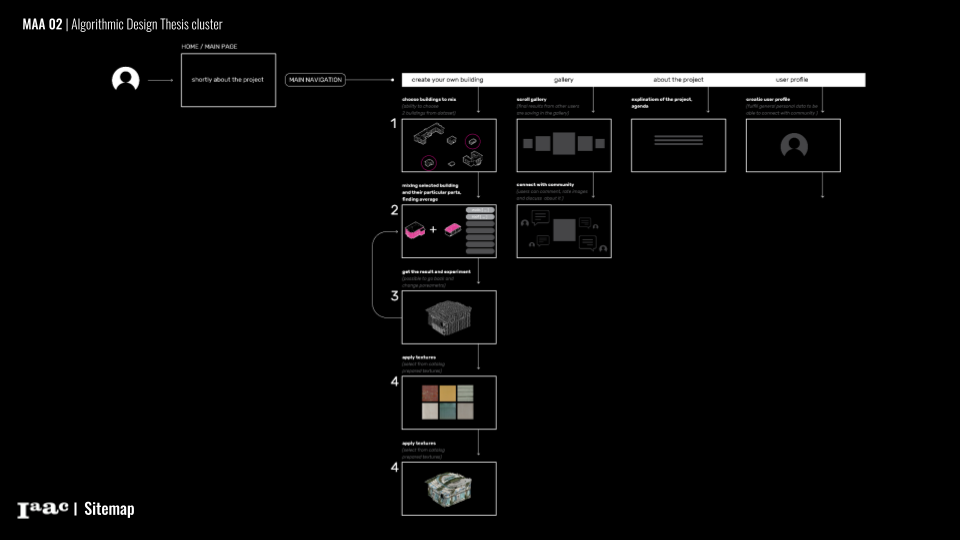