Actually, there is often a significant discrepancy between the designed and the real total energy use in buildings. The reasons of this gap are generally poorly understood and largely have more to do with the role of human behavior than the building design. Human always interact with architecture in their daily life: opening/closing windows, turning on/off or dimming lights’, turning on/off equipment, turning on/off heating, ventilation, air-conditioning (HVAC) systems and etc.
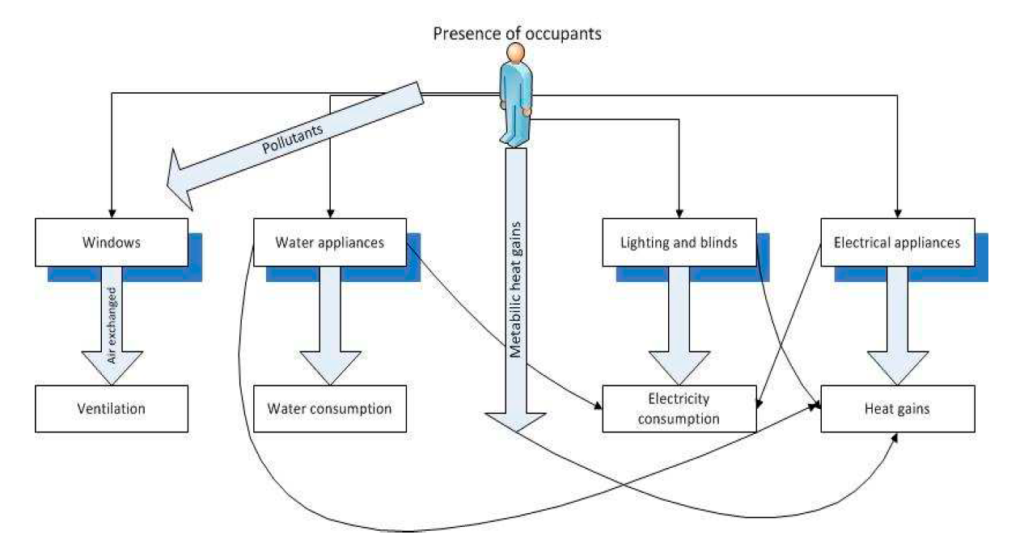
The primary step of occupant behavior research is the collection of behavior data and other relevant variables, together with behavior modeling. In this regard, information related to occupant behavior and environmental or climatic data is collected. The data collection approaches may be performed using the technology-based (e.g., sensor, smart meter, etc.) or survey-based systems. In addition, the selection of data collection approaches should be associated with the purpose of specific behavioral models/approaches. Then, the collected data and the preferred behavioral model will be used to analyze the building energy performance/conservation along with the integration of existing BPS tools (e.g., EnergyPlus, DesignBuilder, etc.) towards further comprehensive analysis.
The whole process is a repeating cycle. When our data is updated, we need to continuously improve the model to obtain higher accuracy.
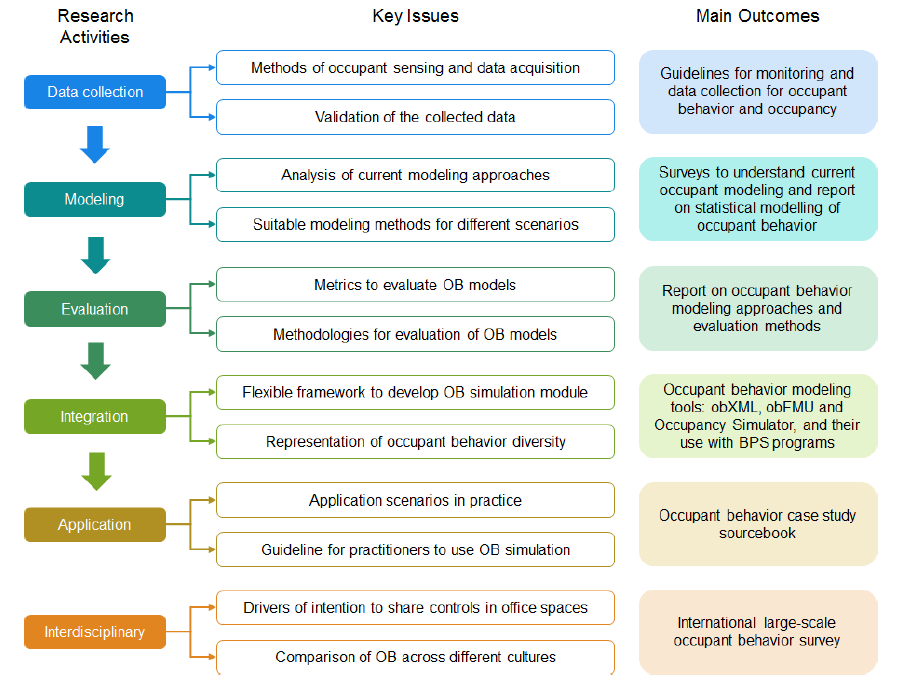
Data collection
An essential part of understanding and modeling occupant behavior is the collection of data. There are various methods of collecting occupant-related data for the purpose of researching building occupants. Sensing occupancy behavior and collecting occupant data in buildings is a non-trivial and comprehensive process. It involves experimental design, sensing and data acquisition, collection protocol and data management. There are four types of experimental approaches: in-situ monitoring, laboratory studies, surveys and mixed method that combines qualitative and quantitative analysis.
Of course, each method has its own pro and cons. We need to choose the appropriate data collection method according to the situation.
Statistical model
The set of mathematical methodologies used in the field of occupant behavior modeling has grown significantly in recent years. Classical statistical models such as general and generalized linear models have been applied extensively. For time-dependent data, Markov and Hidden Markov chains (Dong and Lam 2016, Liisberg etal. 2016, Andersen etal. 2014, Richardsonetal. 2008) have proved to be useful tools. Mixed-effects models have been applied to capture the diversity among occupants, and more recent data mining techniques such as clustering (Panetal., 2017; Renetal., 2015) and decision trees have been used (D’Oca and Hong 2015).
Here are some brief methodological of the modeling approaches used for occupant behavior models.
General linear models(classical GLM)
Generalized linear models (GLMs)
Linear mixed effects models (LMMs)
Hierarchical generalized linear models(HGLMs)
Linear time series models
Markov chains
Hidden Markov chains(HMM)
Bayesian network models (BNs)
…
Model selection is the process of finding the set of predictor variables that build the “best” model. A “good” model is generally considered to be one that explains the observed data well, generalizes to more data, and is as simple as possible (Hastie et al. 2009). In the following list, here are some of the model selection techniques and entities commonly used in the field of occupant behavior modeling.
p-value
maximum likelihood estimation
Akaike’s information criterion (AIC)
Bayesian information criterion (BIC)
k-fold cross-validation
…
The selection and use of specific models need to be analyzed according to specific situations.

Simulation model
We need external tools to help us build models. The aim of these tools is to improve building performance simulation by: (1) providing a standard representation of occupant behavior models, enabling the exchange and use of occupant behavior models between BPS programs, applications, and users to improve the consistency and comparability of simulation results, and (2) generating realistic occupancy schedules. These tools capture the diversity, stochastics, and complexity of occupant behavior in buildings to improve the simulation and evaluation of behavioral measures, as well as of the impact of occupant behavior on technology performance and energy use in buildings.
Here are some Tools:
EnergyPlus: EnergyPlus is a powerful simulation tool to model the heating, cooling, lighting, and ventilation systems.
obFMU: Occupant behavior model, provides the capability to model occupant-based control strategies.
Occupancy Simulator: Occupant movement models using Occupancy Simulator.
Anylogic: Visualization models using Anylogic.
ESP-r: Integration of occupant behavior modeling tools, Linking obFMU with ESP-r enables fully automated co-simulation.

Usually we have the following steps: pre-work(data collection and statistical analysis), define agents, environment Initialization, time Coupling, result validation. And here is a more detail framework from one case study(Simulation and visualization of energy-related occupant behavior in office buildings)
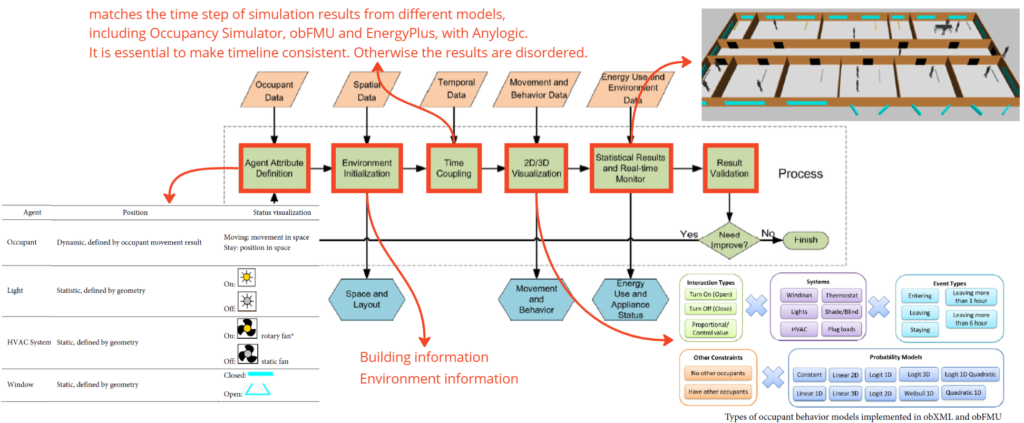
Deficiencies and challenges of current simulation methods
1, Most of the existing research focuses on occupancy or occupants from high-income or developed economies, while information on occupants from low-income or developing economies still remains unclear, complex and conflicting.
2, Most of work are mainly focus on the offices and residential buildings. However, very few articles have examined educational or laboratory buildings. In addition, some other building categories, such as recreational, exhibitions, hotel, clinic, or hospital buildings, have been given sparse attention and require further study.
3, Lack of Qualitative Behavior Research Compared to Quantitative: most of the current research used quantitative research techniques. In the earlier study, the researcher concentrated more on “what” occupant behavior is instead of “how” and “why” occupant behavior is created.
4, Exploitation of Survey or Secondary Data and Lack of Real Data Involvement for ABM Validation: the significant approaches within the preceding research studies used national surveys or secondary data and built ABM approaches without real data involvement.
5, BIM Integration with the Existing Occupant Behavior Modeling/Simulation Approach: BIM-incorporated occupant behavior simulation in buildings helps researchers and engineers to identify the design deficiencies, and improve the overall building performance as well as the automation capability.
References:
[1]. Yixing Chen, Xin Liang, Tianzhen Hong, Xuan Luo(2017). Simulation and visualization of energy-related occupant behavior in office buildings. Building Simulation volume 10, pages785–798 (2017). https://doi.org/10.1007/s12273-017-0355-2
[2]. Da Yan, Tianzhen Hong(2018). EBC Annex 66 Final Report – Definition and simulation of occupant behavior in buildings. ISBN 978-0-9996964-7-7.
[3]. Mr. Mohammad Nyme Uddin, Dr. Hsi-Hsien Wei, Dr. Hung-Lin Chi, Prof. Meng Ni(2021). Influence of Occupant Behavior for Building Energy Conservation: A Systematic Review Study of Diverse Modeling and Simulation Approach. Buildings 2021, 11(2), 41. https://doi.org/10.3390/buildings11020041
[4]. Max Marschall(2020). Humans in the Loop: incorporating Occupant Behaviour in Building Performance Simulations. Ph.D. Thesis. RMIT University. https://doi.org/10.13140/RG.2.2.11677.54249
[5]. Page, Jessen(2007). Simulating Occupant Presence and Behaviour in Buildings. Ph.D. Thesis. Lausanne, EPFL. https://doi.org/10.5075/epfl-thesis-3900
[6]. Salvatore Carlucci, Marilena De Simone, Steven K. Firth(2020). Modeling occupant behavior in buildings. Building and Environment 174:106768. https://doi.org/10.1016/j.buildenv.2020.106768