Methods for Encoding and AI Generation of Architectural Geometry
MaCAD Digital Tools for GENERATIVE PLANNING SEMINAR
“The promise of deep learning is to discover rich, hierarchical models that represent probability distributions [and] map a high-dimensional, rich sensory input to a class label” (Ian Goodfellow et all – GANs paper)
While in the previous decades, predictive and discriminative AI became evermore embedded in our daily lives – computer vision is used in most consumer and industrial devices, predictive analysis guides your daily choices, etc. – it is clear that generative AI is the big bet for the upcoming years. As this branch of machine learning is finally reaching the wider public with its hyper accessible interfaces and emotionally triggering results, it’s important to address its potential impact in Architecture, and more generally in the AEC. Even with the limited applicability (2d images), stable diffusion models were responsible for a considerable portion of the visual content posted under the #architecture in 2022.
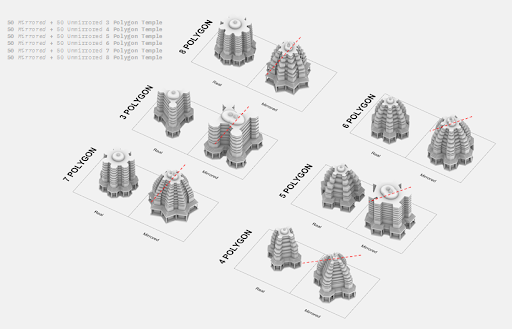
Source: Post Digital Temples – Mahmoud Ramdane | Naitik Sharma | Daniyal Tariq – Macad 2022
With equally passionate supporters as critics, these images drew attention to the need for AI methods that solve AEC problems, as well as to the complexity that future AI tools for AEC need to address.
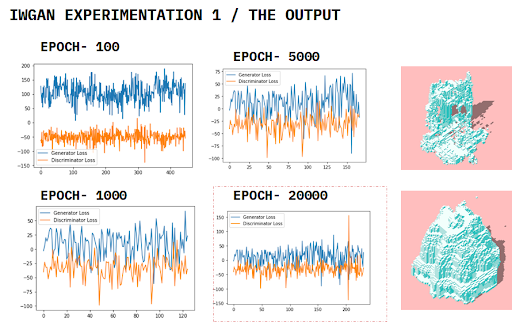
Source: Post Digital Temples – Mahmoud Ramdane | Naitik Sharma | Daniyal Tariq – Macad 2022
In this course, we will go from studying the fundamentals of the AI paradigm, to learning and applying key generative AI models, to a more in depth study of the concepts pertinent to the application of AI for the generation of sparse 3d objects.
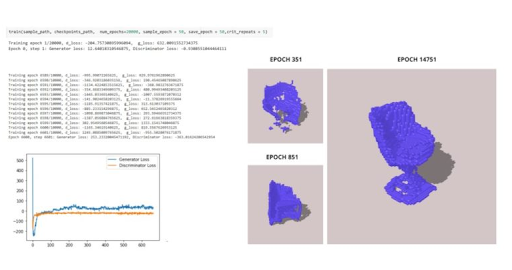
Source: Ch-AI-r convergence – Maria Papadimitraki | Michal Gryko – Macad 2022
The overarching goal of this course is to brainstorm and explore potential applications that enable AI generation of meaningful geometry for Architecture and Engineering. For this we will investigate topics of data representation, synthetic data generation, and conditional and unconditional models, in an intent to understand the end to end pipeline and parameters that come into play in constructing the optimal model for such problems.
A more expanded discussion on the topic of AI in architecture can be found here.
Learning Objectives
- Get introduced to fundamental concepts of AI and its applications in Architecture.
- Understand Generative AI concepts and Models: methods, layer types, hyperparameters
- Construct and manipulate Generative Adversarial Neural Networks models
- Learn to explore and manipulate the latent space
- Set up a synthetic data generation algorithm and run experiments generate and recreate new data