Our project derives from our observation that in Singapore, most of hotels are located along the east and southeast areas near Changi Airport. There are very few hotels on the west side of Singapore. We also take into account on factors that tourists and visitors consider when making a reservation. These factors include public transportation, nearby amenities, parks and gardens, tourist attractions, and Singapore street food (Hawker Centers).
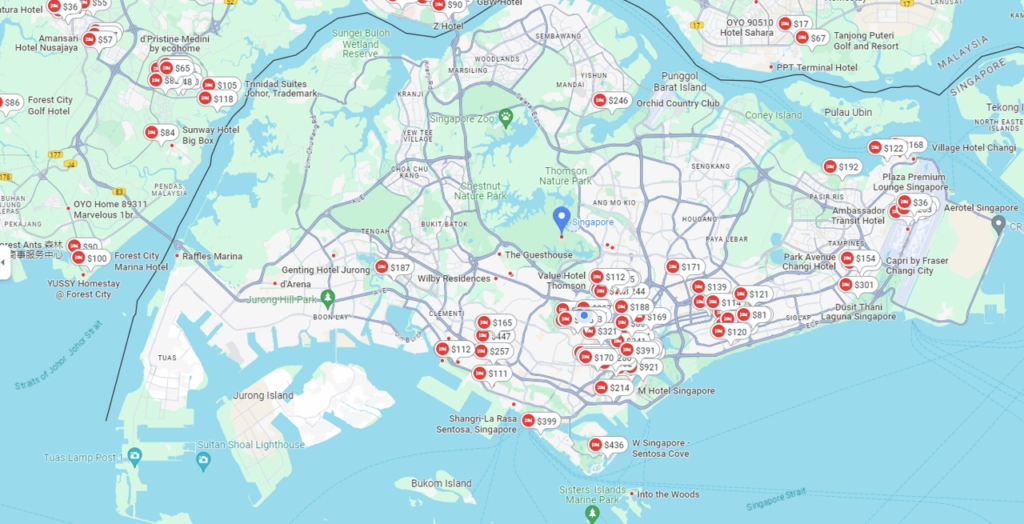
We start to plot our graph with open street map background with Hawker Centers, restaurants, MRT (public transport) stations, and existing hotels as shown below.
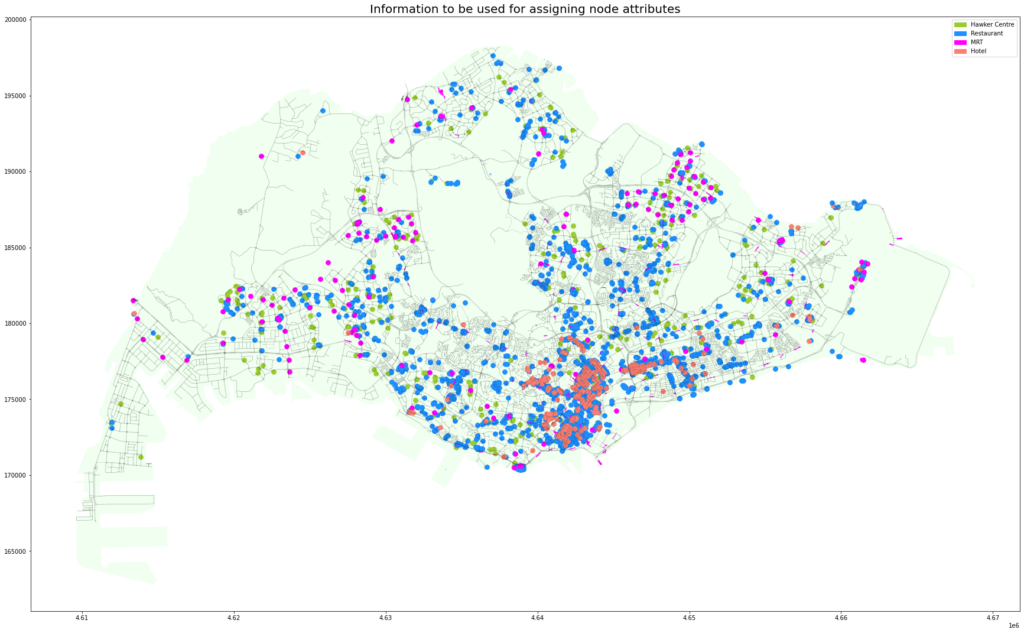
Then, we develop a bounding box for Hawker Centers, restaurants, MRT (public transport) stations as well as defining 7 clusters of proximity clusters.
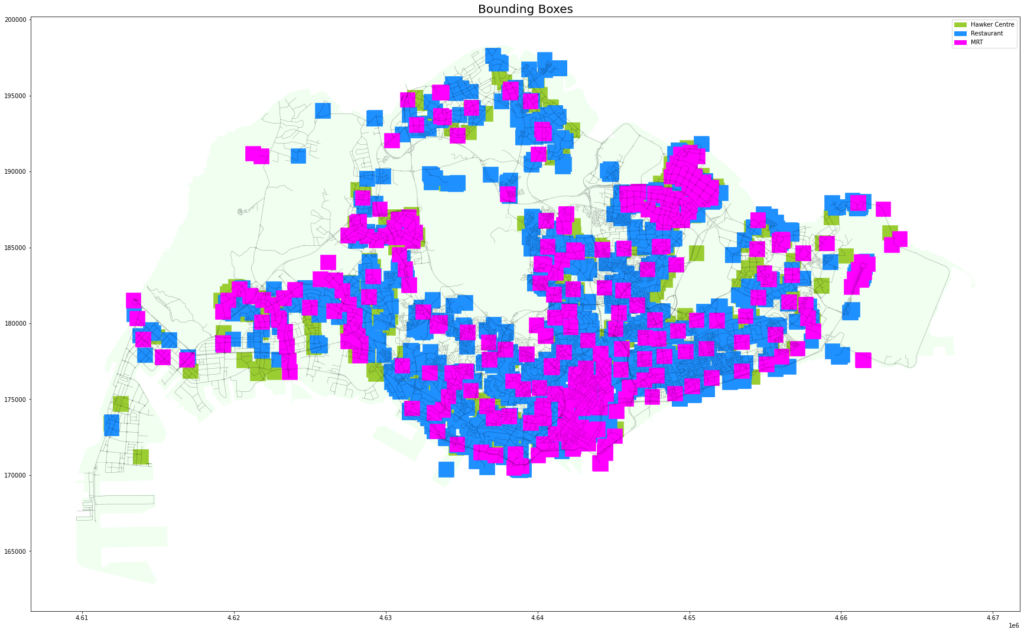
We plot the heat map for all attractions.
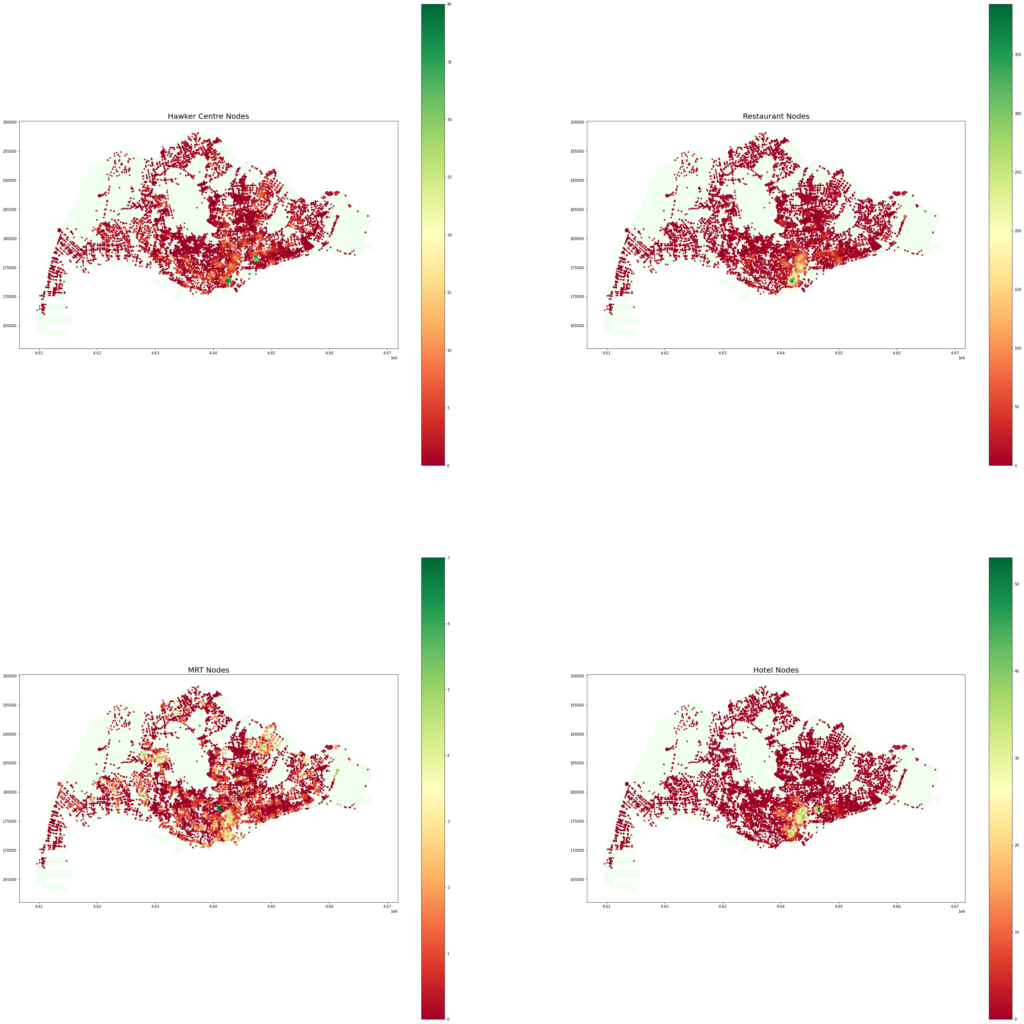
Thereafter, we define our classification to 3 classes: No Hotel, 1 hotel and more than 1 hotel.
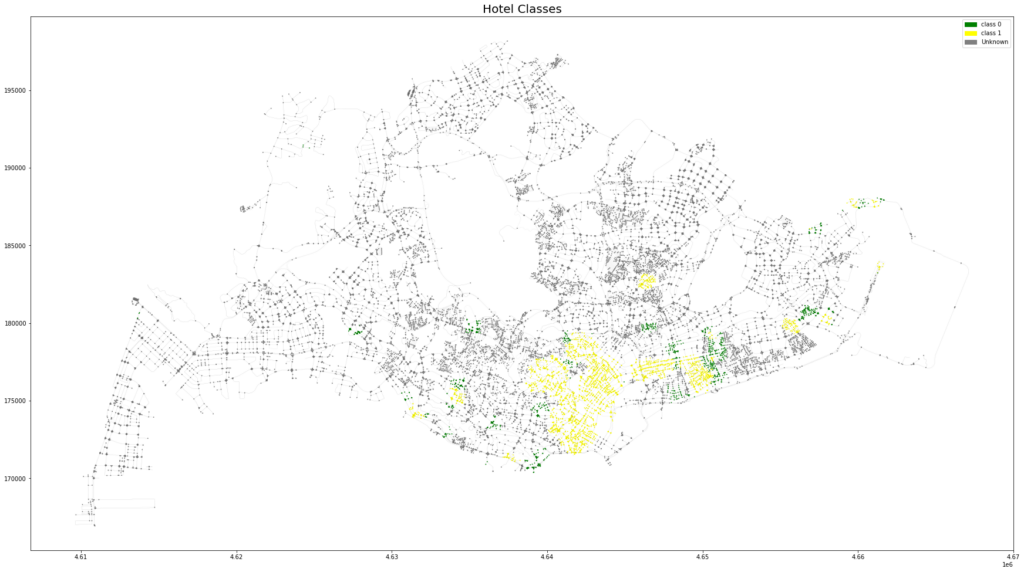
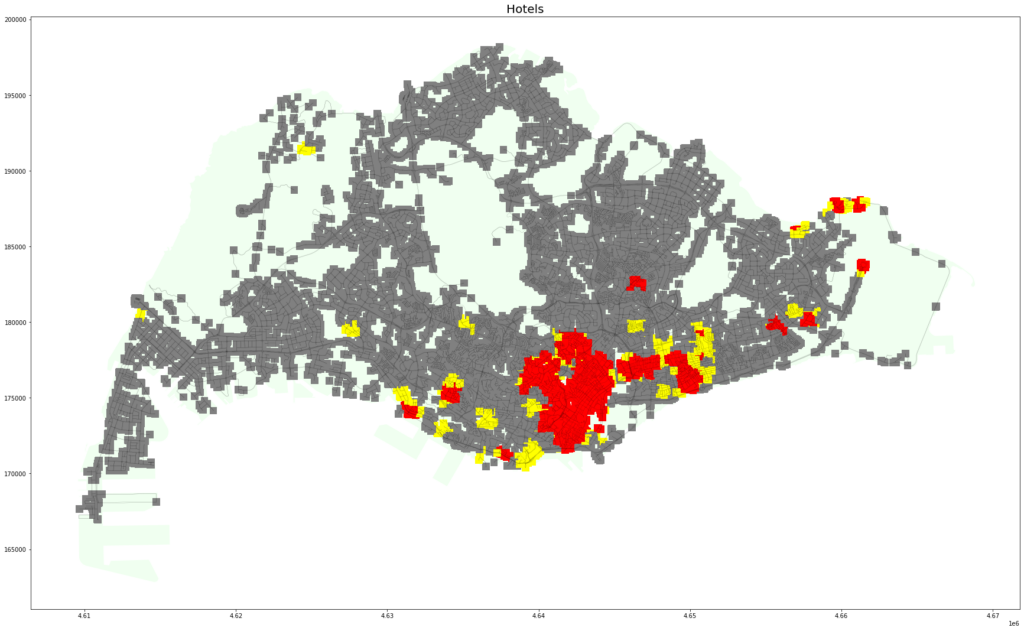
As expected, there are a lot of grey bounding box outside of east and southeast areas of Singapore. We train the graph ML with our data set.
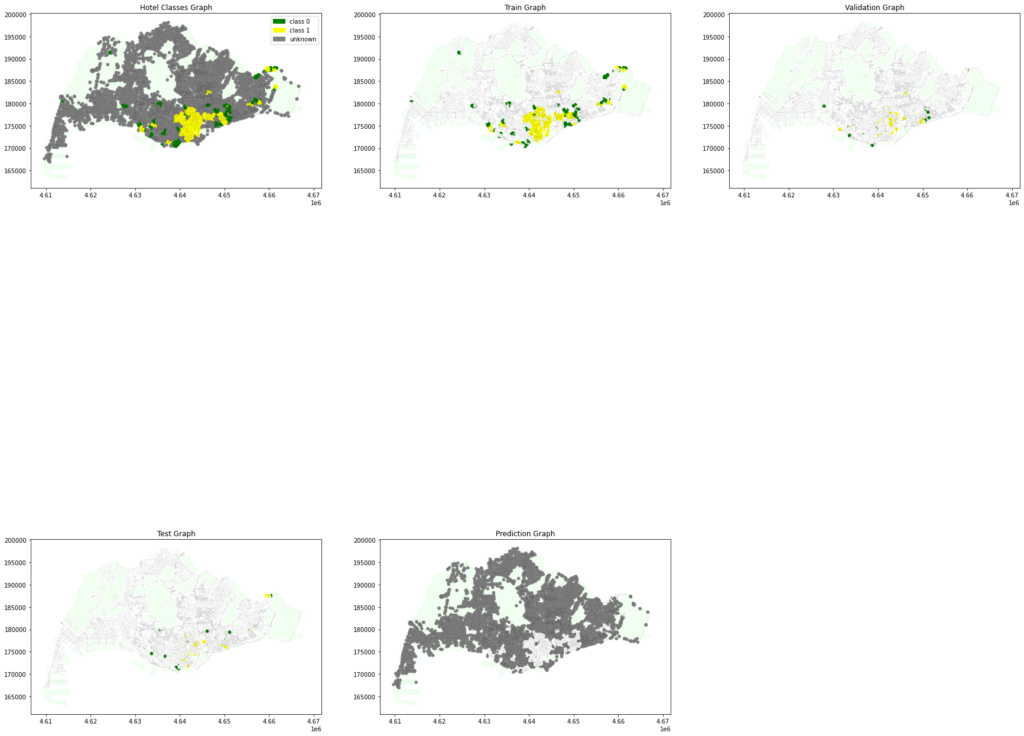
At the end, we are able to projected other areas in Singapore to have more hotels.
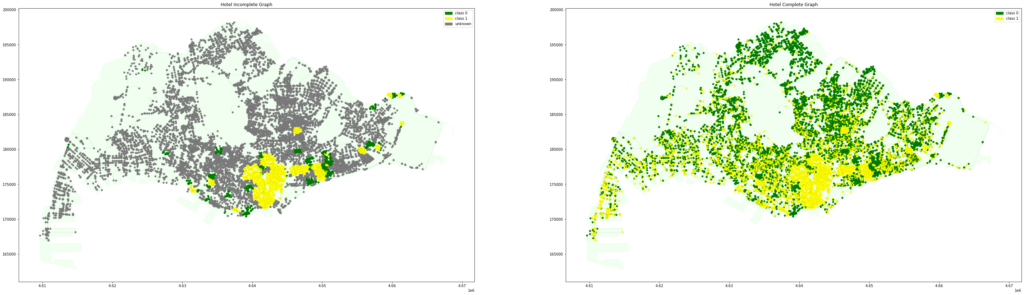
The journey of our graph ML final project was not that simple. We got stuck along the way as at the beginning we never define our no hotel zone, this results in a failure to train our model to populate more hotels near by the amenities. It took our efforts to flip the equation and asked our questions in a backward way. This approach results in a more successful attempt for our final project.
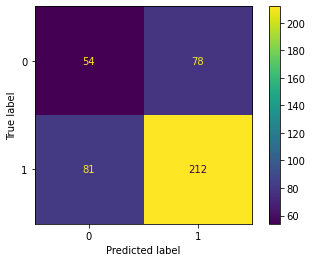