Introduction
In 1950, Alan Turing posed a fundamental question, “Can machines think?”. Our exploration aims to extend this quest by considering additional questions: can machines develop the higher-order thinking described, as described in Bloom’s taxonomy? Can they create, dream, or imagine?
To understand imagination and machine creation, we first examine perception, i.e., the processes of information gathering and interpretation. We focused on the concept of human pareidolia and its reflection in machine perception and imagination.
Pareidolia refers to the psychological phenomenon in which humans perceive familiar patterns, such as faces or objects, in random stimuli such as clouds or rock formations. Essentially, it is the mind’s attempt to make sense of ambiguous or random inputs by identifying familiar patterns.
The concept has historical roots, such as Carl Jaspers’ 1958 publication on the early stages of schizophrenia, in which he observed abnormal meanings and overinterpretations of sensory perceptions. He did not classify them as hallucinations, but as indicators of the early phase of mental illness. This phenomenon is closely related to apophenia, the tendency to perceive connections between unrelated things.
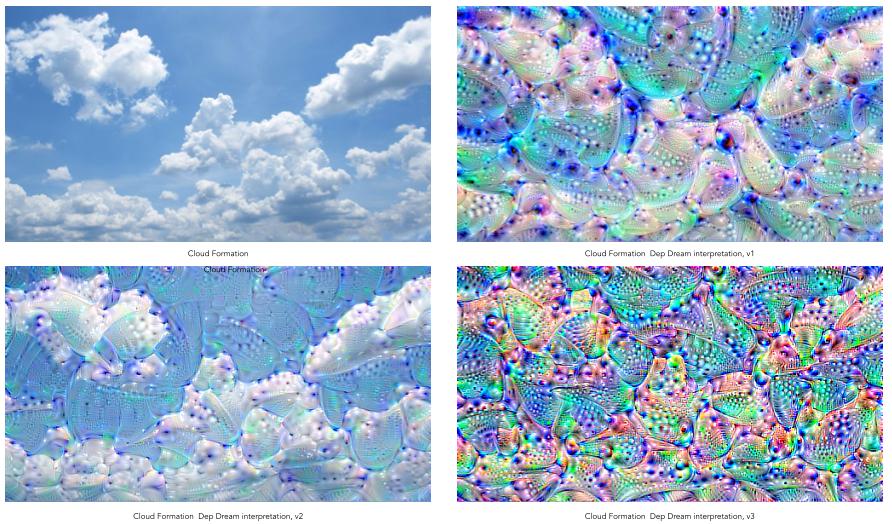
Machine Perception and Pareidolia
Artificial intelligence shows similar tendencies. When machines attempt to make sense of patterns, they may incorrectly identify familiar shapes or structures in ambiguous images, which is a case of automatic pareidolia. This reflects biases present in the training data, analogous to human biases in interpretation. Although often considered a limitation, pareidolia can also be an advantage.
AI systems can potentially benefit from pareidolia to contribute to creativity, innovation and discovery through serendipity. By identifying unexpected patterns that humans do not see, AI can produce surprising results, thus opening up possibilities for machine “creativity.”
These perceptual effects can facilitate artificial creativity by enabling creative interpretations of data, similar to abstract art. This process provokes multiple interpretations from viewers, encouraging exploration of new ideas, styles, and methodologies that may be beyond the reach of humans or machines alone.
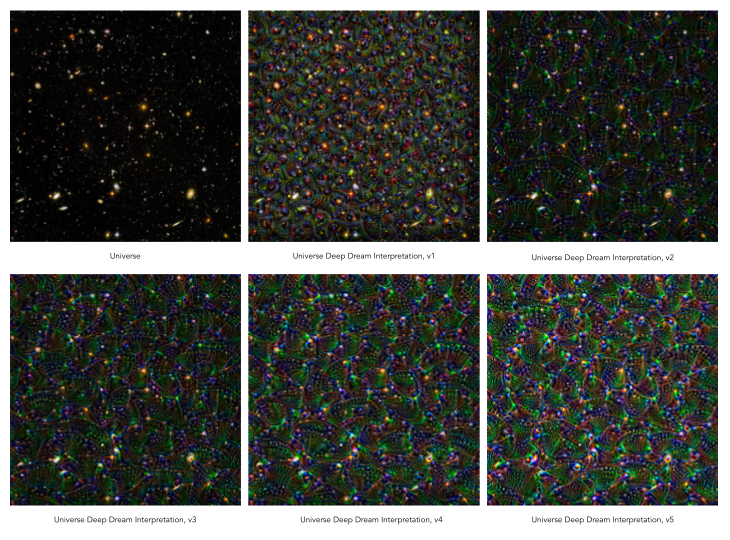
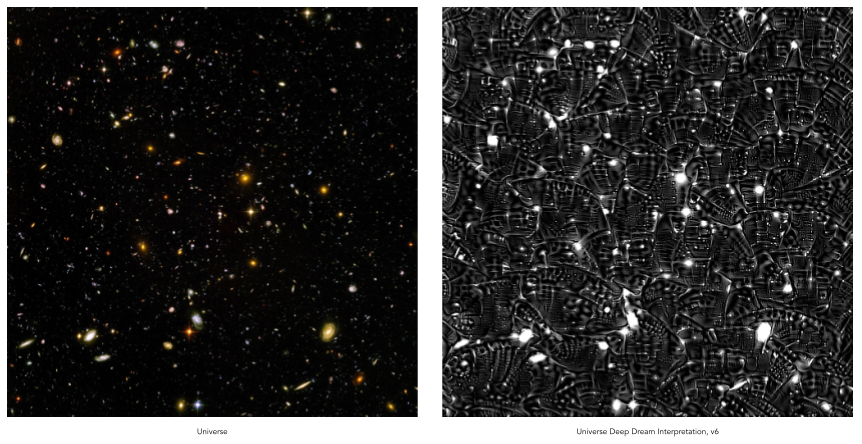
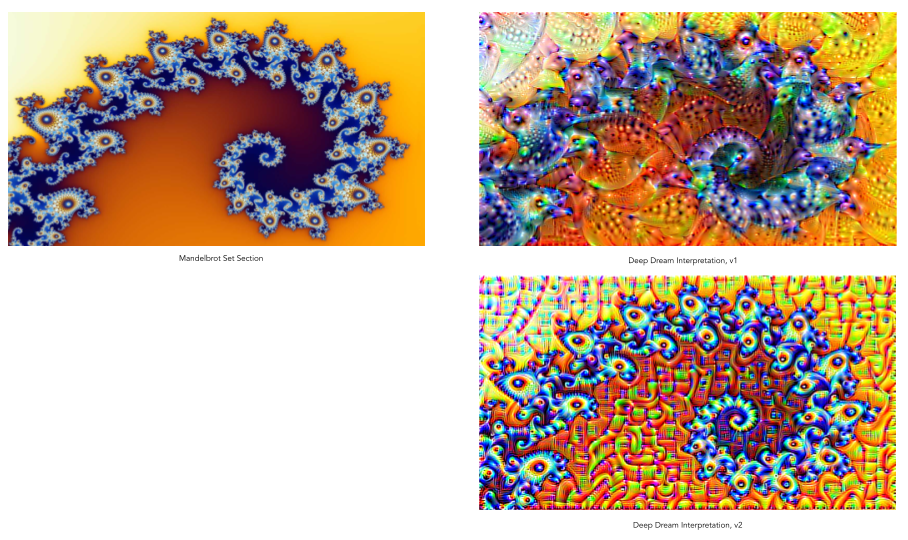
Rorschach Test for Machines
Our exploration adopted a method similar to a Rorschach test for machines. Traditionally, the Rorschach test consists of asking individuals to describe what they see in ink stains, revealing aspects of their personality. We use Google’s Deep Dream, a convolutional neural network that modifies the patterns it “sees” in images. By providing an image and allowing the machine to interpret it, we tried to discover patterns that humans might not perceive.
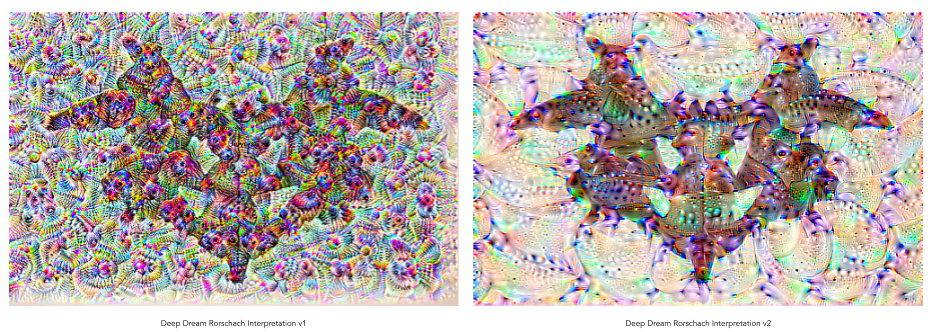
For our test images, we used a variety of sources, from identifiable to abstract patterns, to see what the machine could distinguish and create from near-random inputs. To take the experimentation a step further, we employed different types of visual noise, such as white noise, color noise, cellular noise, Worley noise, and Perlin noise.
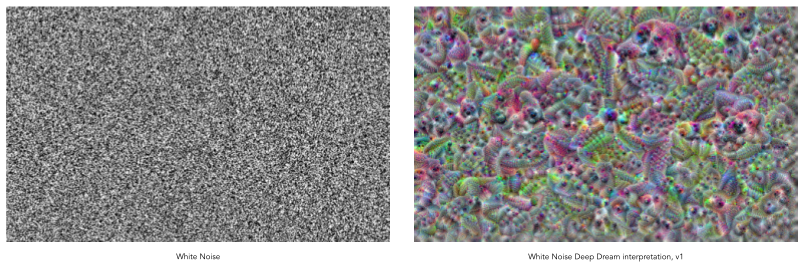
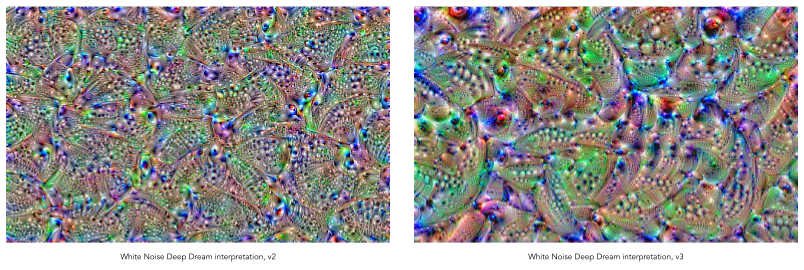
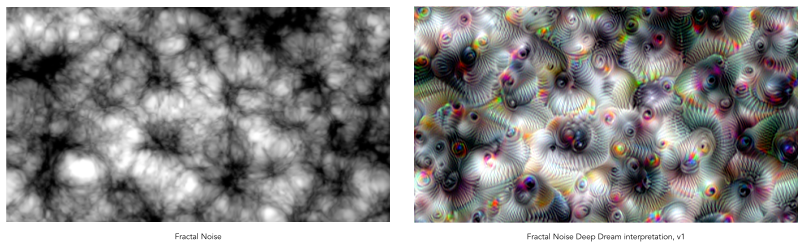
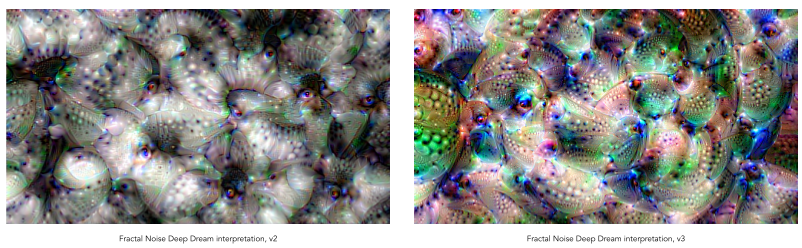
Conclusions
Rather than definitive conclusions, this experiment raised other questions. One of them concerns the importance of interpretation in both human and machine perception. The results invite multiple interpretations, as does human art, highlighting the role of the observer in the creation of meaning. This interaction suggests that meaning is constructed through the interaction between the observer and the observed.
This exploration also raises the question of whether AI can truly “create” or whether it merely simulates creativity based on programmed algorithms. Our findings indicate that AI has the potential to offer creative ideas and interpretations, although in ways that challenge traditional notions of creativity and imagination.
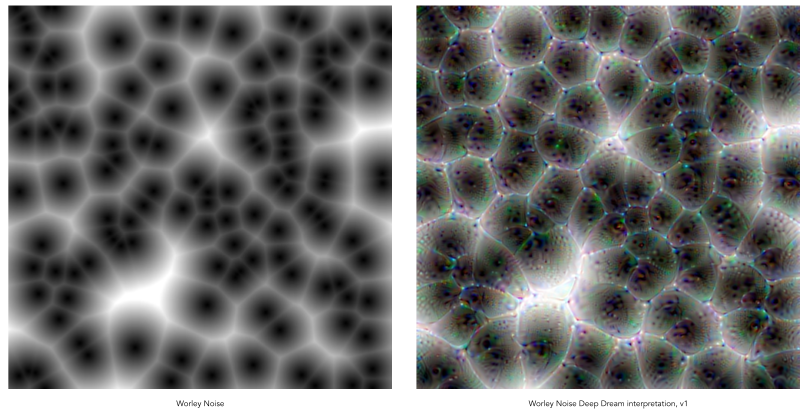
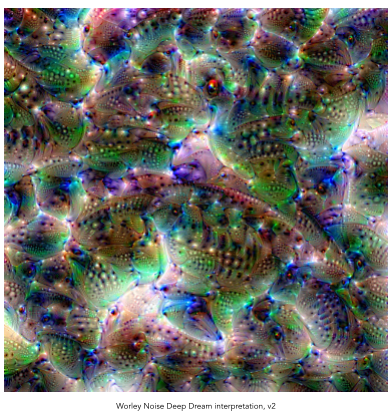