Introduction
Human behavior has a significant impact on building energy performance and is often overlooked in favor of upgrading systems or technology. Building simulations often rely on deterministic models, but these models can be inaccurate due to the variability of human behavior. Stochastic models allow for more flexibility in assumptions and predict a range of possible scenarios and the likelihood of each occurring. They can be more accurate in predicting energy use in buildings as they include variability for human behavior (Chen et al, 2017).
In the future, it is likely that buildings will become more intelligent through the use of data collection, machine learning, and artificial intelligence. Cameras and sensors can be used to collect data on the movements and activities of building occupants, as well as environmental factors such as temperature, water-use, energy-use, and light levels. This data can be used to improve building simulations and optimize building performance.
Personas can help designers and developers move away from designing for themselves or for a generalized average user, and instead design for specific individuals with specific needs. This approach can help create more targeted and effective simulations and predictions for buildings. Personas can also be helpful in understanding the diversity of user needs and preferences within a given context, making design more inclusive and accessible to a wider range of users.
Collecting Better Data
Cameras & Sensors
Cameras and sensors are useful tools for collecting data about buildings. For example, building management systems can collect data from smart thermostats that gather information about temperature preferences and scheduling. However, it is important to be aware of the potential for corruption of the accuracy of the data. People change their behavior when they are aware that they are being observed, such as drivers who know the locations of onlooking speed cameras.
Low-energy sensors can be used in buildings to collect data on various aspects of the building’s environment and usage. MIT Media Lab’s City Science group has been working with environmental sensors called MITes and TerMITes. These sensors are used to track when cabinets are opened and closed or when appliances are used (kllmit, 2017). This information can be used by building managers to understand how their building is being used and how energy is being consumed. By analyzing this data, patterns can be identified to make predictions about energy consumption, maintenance needs, and occupant behavior. If a building has data on how often its occupants use the stove or oven, it can make more accurate predictions about energy consumption and plan for maintenance or upgrades as needed. Additionally, understanding the usage patterns of appliances can help building managers identify opportunities for energy conservation, such as encouraging occupants to use appliances during off-peak hours or promoting the use of energy-efficient appliances and practices.
Emotional State
Jonathan Harris and Sep Kamvar launched We Feel Fine on the web in 2005, scraping blog posts for any sentence that contained a feeling. 600,000 feelings were collected over three years. Dominant emotions can be examined by region throughout the world at any given time. Maps showed the level of happiness, loneliness, sickness, religiosity, and those feeling overweight across the USA (Kamvar and Harris, 2009). Other views could segment the data by age, gender, or even weather. How do people feel on hot days versus cold days in different parts of the world?
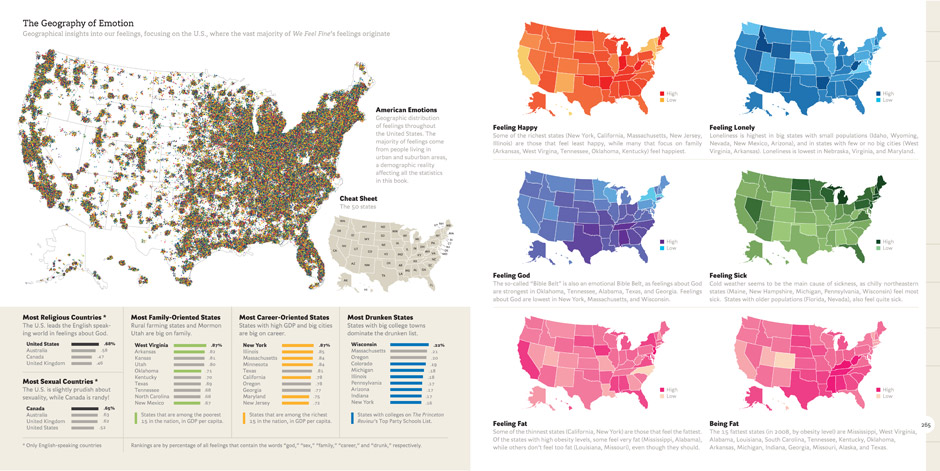
Early versions of facial expression databases included the position of all facial muscles that expressed the emotions of anger, fear, disgust, surprise, joy, and sadness. They were used extensively by filmmakers and psychologists. The most well known is the Facial Action Coding System (Eckman and Friesen, 1978). Newer databases contain much more detail differentiating between various types of smile such as the Pan-Am Smile and the Duchenne Smile. Researcher Javier Hernandez applied this research while working with the Affecting Computing group at MIT’s Media Lab. His project, the Mood Meter, recognized the strength of every passerby’s smile. The system was deployed across the MIT campus, producing a heatmap of the most happy and least happy areas on campus. Segmenting the data over time showed that pedestrians on campus were most happy on weekends and least happy on Tuesdays. (Hernandez, Hoque, and Picard, 2012).
Capturing the emotional state of building occupants may be useful in predicting water-usage. Research from John Bargh and Idit Shalev found that people who feel lonely take longer showers at higher temperatures (Shalev and Bargh, 2012). Understanding the emotional states of occupants can better predict future building energy and water usage.
Predictive Analytics & Habits
Predictive analytics are used to identify patterns and predict future behavior in people. Corporate marketing teams have used this technology to design more effective products and services. Charles Duhigg wrote an article for the New York Times titled “How Companies Learn Your Secrets” (Duhigg, 2013). The article centers around statistician Andrew Pole, who dived into the lives of Target’s consumers. A basic use of the technology was to identify the age and gender of each child in a family to market that family the best toys. Smarter predictions looked at products of association. If a customer bought a swimsuit in April, target them with coupons for sunscreen in June. If a customer bought cereal but not milk, send that customer coupons for milk, assuming milk was purchased from a competitor.
Marketers also knew that a consumer’s purchasing pattern was most likely to change during a period of flux in that shopper’s personal life. The gold standard became predictions of pregnancy and marriage. After many tests, Pole found a list of 25 products that when purchased together, having not been purchased previously, would assign a pregnancy prediction to that shopper. One year later, a furious father walked into a Target in Minneapolis, MN. “My daughter got this in the mail! She’s still in high school, and you’re sending her coupons for baby clothes and cribs? Are you trying to encourage her to get pregnant?” The manager apologized and called a few days later to apologize again. But the father had learned something new in the intermittent few days. He explained, “She’s due in August, I owe you an apology.” Not only did Pole’s pregnancy predictor work; it learned such sensitive news earlier than everyone else.
Overall, our goal is to use data to create more efficient and comfortable buildings that are responsive to the needs of their occupants. But if purchasing patterns are most in flux during a period of change in consumer’s personal lives, then are energy-use patterns also most in flux during the same time? The best opportunity for a building to change an occupant’s behavior from always using the air-conditioner to opening a window once a while may lie in this story of predictive analytics and pregnancy.
The article concludes with a segway to Duhigg’s book The Power of Habit released in the same year: “Duke University estimated that habits, rather than conscious decision-making, shape 45 percent of the choices we make every day.” The habits of specific occupants are what buildings should aim to better understand (Duhigg, 2014).
Overconfidence
UX designer Josh Clark of Big Medium is skeptical about the confidence our current technology claims to have. In his talk titled “Design in the Era of the Algorithm,” he discusses AI image recognition (Clark, 2022). The AI sees a dinosaur on top of a surfboard. It obviously got the dinosaur correct as well as its position. But is that a surfboard underneath? It’s a scale allowing viewers to understand how big this dinosaur was. Clark’s frustration was the level of confidence the technology claimed in the statement “a dinosaur on top of a surfboard.” Digging into the meta-information, he discovered that the AI was 97% confident in its dinosaur claim, while only being 26 % confident in its surfboard claim. So why the absolute confidence? Clark proposed an alternative user-interface that better explains the level of confidence an AI has about its claim. He detailed a number of examples where technology companies overstate the confidence of their algorithms. We trust our lives with technology, and we make big decisions based on the analysis made by technology. We really need to know when technology is 97% sure of its claim or just 26% sure. (Clark, 2022)
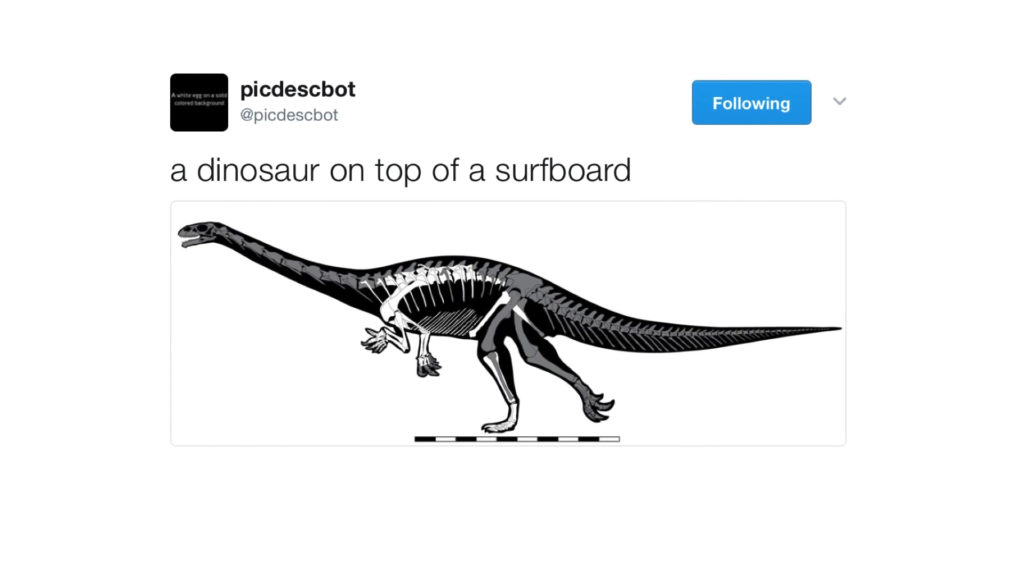
Ethical Questions
The use of data collection and analysis tools in the built environment raises important questions about privacy and trust. Different individuals and communities may have different levels of trust in different organizations or institutions to handle sensitive data. Google Health was being discussed in a class in London that included Scandinavian students alongside American students. The Scandinavians found the idea that a corporation would hold their private medical information highly objectionable. They would only trust their government with such sensitive data. The American students rebounded in collective agreement that they would never trust their government with their private medical information but had no problem trusting a corporation. The world may differ in who it trusts with its private information, but this story highlights that whoever holds that data has an enormous responsibility to keep that data safe.
Some organizations, such as Numina, take additional measures to protect personal information by deleting it before uploading data to the cloud and using techniques such as blurring faces and license plates in order to protect the privacy of individuals. Their slogan “intelligence without surveillance” accurately describes their mission. (Pham, 2021)
Numina tracks street movements but deletes private information before uploading to the cloud.
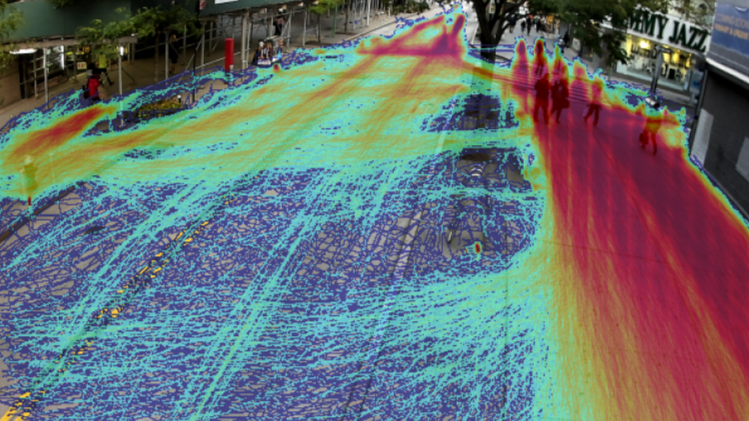
Creating Personas
Malcolm Gladwell discussed the story of Howard Moskowitz at length in his TED talk “On Spaghetti Sauce” (Gladwell, 2022). The prevailing wisdom at the time was the need to find the product that most people preferred. Le Corbusier’s Modulor (1943), based on a man of average height who liked thermal comfort at an average temperature, would have liked the average pasta sauce. Moskowitz had a eureka moment while working with Pepsi, finding that there was “no perfect product only perfect products.” This became his favorite saying that he would repeat to all potential clients. He found clusters of people with similar preferences, but concluded that averaging everyone’s preferences resulted in something that nobody liked.
Prego asked Moskowitz to research what was the favorite pasta sauce of American consumers. Testing 45 different varieties of sauce on thousands of people, he found three distinct groups: plain, spicy, and extra chunky. Extra chunky was the most remarkable, as nobody in the United States was selling extra-chunky sauce. Moskowitz claimed companies weren’t aware that one third of Americans preferred extra-chunky pasta sauce, as the data had always been averaged. Prego’s extra chunky pasta sauce was a hit, resulting in market dominance for years to follow (Gladwell, 2022). Moskowitz’s contributions to the field of marketing are known as horizontal segmentation.
The idea of “personas” in user-experience design is quite similar to Moskowitz’s idea. They first appeared in the book The Inmates Are Running the Asylum by Alan Cooper, who had a degree in architecture (Cooper, 2015). Personas are specific individuals with very specific needs. A persona is often a fictional character that is given a name by the design team and used by designers to move away from designing a product for themselves. The idea is that designers design for multiple personas, such as those who like plain, spicy, and extra-chunky pasta sauce.
Many products, especially those in fields of technology, suffer from self-referential design. New Zealander Richard Lee discovered this first-hand when attempting to renew his passport. The system claimed that his eyes were closed and could not proceed with his passport renewal (Reuters Staff, 2016). The real problem was that the designers of European descent had only tested the system on themselves before launching it nationwide. People of Asian descent were all told that their eyes were closed, as the system tried to eliminate passport photos with closed eyes.
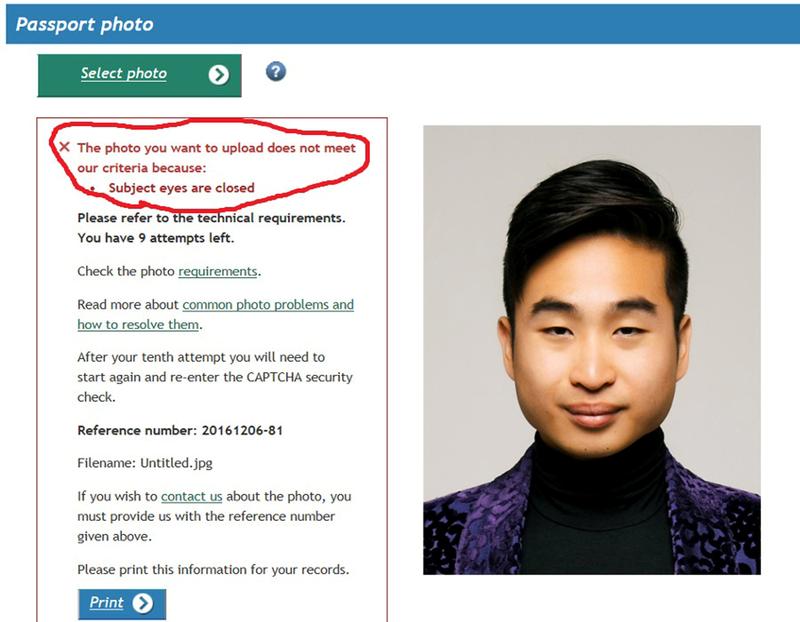
Marathons are another great example with multiple persona types, where each persona has very little in common with the others. Few are trying to win the marathon with a sub-2 hour and 30 minute time; some want to run a sub-4 hour marathon, many just want to complete a marathon before the roads reopen, others raise money for charity, people with disabilities are there to complete a goal, sponsors want to gain attention, and the people on the sidelines want to make people happy cheering everyone along. These personas have very different goals, different training schedules, and wear completely different shoes. The first two groups will likely pay enormous amounts for carbon plate shoes, whereas the other groups would certainly find the price of those shoes ludicrous! Separating the data in persona types sorts chaos into useful and actionable information.
Current simulations, such as this evacuation simulation by Arup / Oasys, treat all agents as identical. The simulation therefore shows the agents exiting the building like robots (TheOasysSoftware, 2022). Even in a stochastic model where agents might vary their speed with a maximum and minimum, this would not closely reflect reality. A persona-based simulation would identify different groups using some of the data collection techniques mentioned above. For example, how many cat owners are in this building is a question that should be answered. Cat owners spend a number of minutes searching for their cats when alarms go off, as the cats are terrified from the loud sound. My experience (as an owner of two cats) is that cat owners depart the building eight to ten minutes later than the majority of people. Another question to ask is how long does it take people with mobility disabilities to leave the building? How about parents with young children? Simulations going forward need to be more intelligent both in the data they collect and results they deliver.
Synthetic Populations
“What are synergies between synthetic populations (SP) and persona-based approaches, and how can they strengthen and complement each other?”
An overview of Personas and Synthetic Populations
Personas are fictional characters that are created to represent different types of users or customers in a product or service. They are often used in user-centered design and marketing to help designers and marketers better understand the needs, goals, and motivations of their users.
Personas are typically developed based on research about the target audience, such as user interviews, surveys, or focus groups. They are designed to be representative of a particular segment of the user base and are used to help designers and marketers make informed decisions about the product or service they are developing.
Personas are often given a name, age, occupation, and other personal characteristics to make them more relatable and easier to understand. They may also be given a backstory and specific goals and needs to help designers and marketers understand how they might use the product or service.
By using personas in the design and marketing process, it is possible to create products and services that are better tailored to the needs and goals of the target audience.
Synthetic populations are computer-generated groups of individuals that are designed to represent a real population in terms of characteristics such as age, gender, income, education, and occupation. Synthetic populations are often used in urban planning, transportation modeling, and other applications where it is necessary to simulate the behavior and interactions of a large group of people.
Synthetic populations are created using data from real populations, such as census data or survey data. The data is used to create a statistical model that represents the characteristics of the real population. This model is then used to generate a synthetic population that is statistically similar to the real population.
Synthetic populations have several advantages over real populations in certain situations. They can be generated quickly and inexpensively, and they can be used to test scenarios or policies without the need for real people to participate. They can also be used to protect the privacy of individuals by allowing researchers to work with data that has been aggregated and anonymized.
Existing approaches combining big data and personas
It is true that recent studies have attempted to use more quantitatively informed approaches, such as surveys and big data, to create more detailed and accurate personas. Similarly, some studies have attempted to enrich the characteristics of synthetic population agents by incorporating persona model attributes using semantic technology. These approaches can provide valuable insights and can be useful in certain contexts, but they may not fully consider the bidirectional communication between personas and synthetic populations or be able to adapt to future situations.
One way to address this challenge may be to use a more dynamic approach to creating and linking personas and synthetic populations. For example, rather than creating a static set of personas and synthetic population agents, the personas and agents could be continually updated and refined based on real-time data and feedback. This could involve incorporating data from surveys, big data, and other sources to continually refine the characteristics and behaviors of the personas and agents, and using machine learning or other advanced techniques to enable the personas and agents to adapt and evolve over time.
Another approach might be to use a more integrative approach to linking personas and synthetic populations, where the personas and agents are created and analyzed together as part of a single system. This could involve using both real data and simulated data to create and refine the personas and agents, and using tools and techniques from both fields to analyze and understand the dynamics and patterns within the system.
Overall, there are many potential ways to strengthen and complement the synergies between synthetic populations and persona-based approaches, and more research and experimentation will likely be needed to identify the most effective and practical approaches.
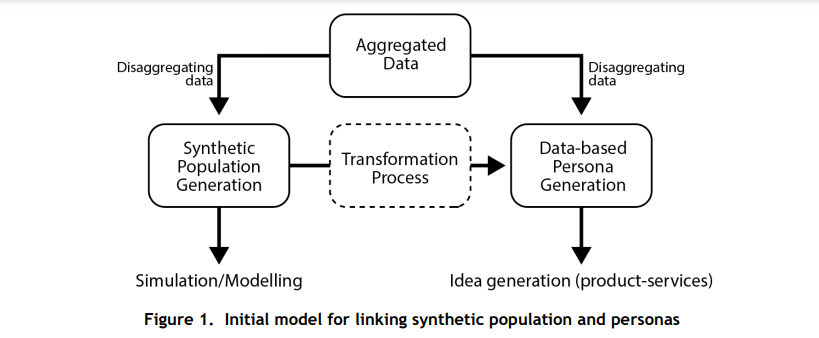
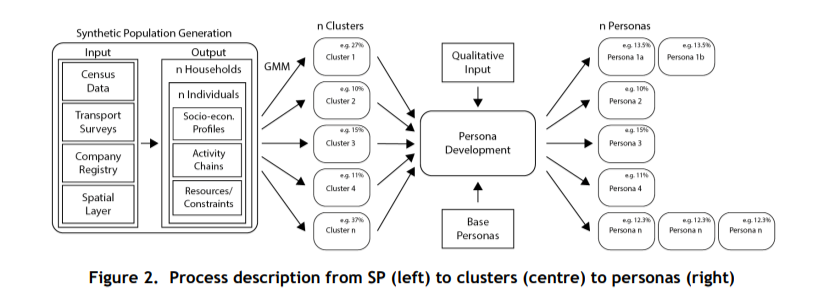
Simulating Personas
In order to define personas, some of the researchers have conducted target group interviews of potential subjects. Using these interviews, a set of archetypes can be constructed that personify certain predominant characteristics and significant behavioral patterns. This information can then be divided based on the unique characteristics and goals of these studied subjects.
In the paper authored by Taro Kanno, Tomohiko Ooyabu and Kazuo Furuta, a method was proposed to integrate human modeling and simulation using the persona method to predict residents’ behavior in an emergency situation and design emergency announcement strategies (Kanno et al., 2011). Based on the target group interviews, they arrived at five key characteristics which can significantly affect the way the occupant would evacuate in an emergency situation. See an example from the study below that shows key characteristics of the target groups.
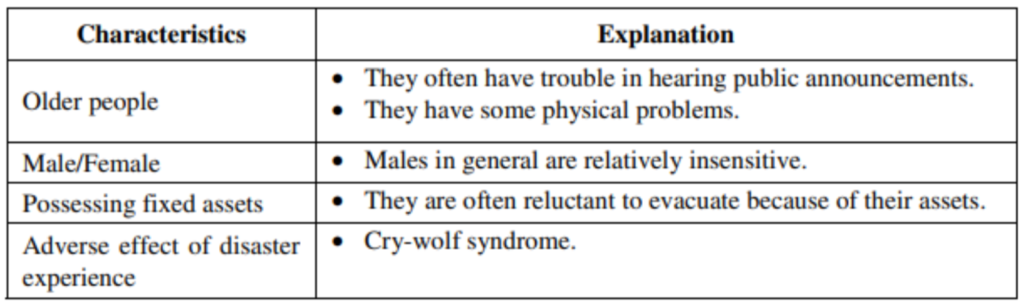
Based on this, various fictional personas can be created similar to one shown in seen below.
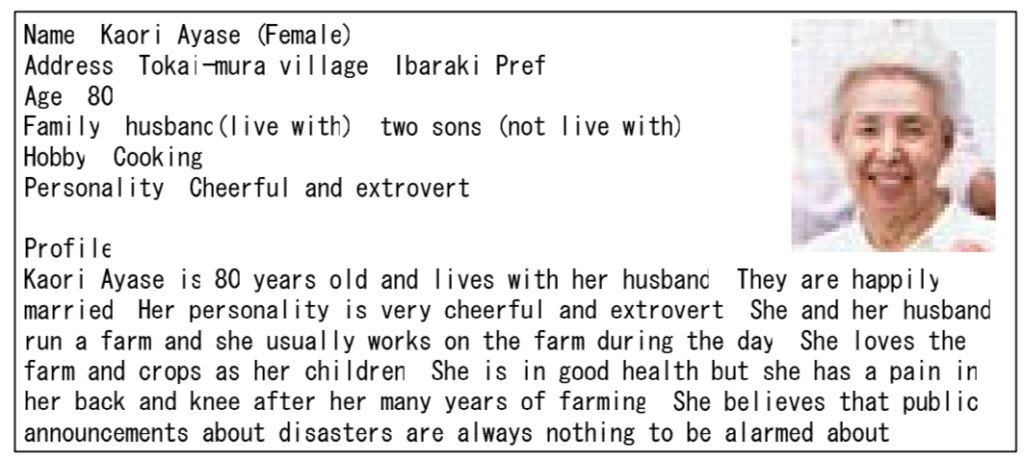
Studies using these methods can provide valuable data regarding different situations and events happening in the simulation and also be used to analyze various characteristics of different personas.
In conclusion, the Persona method seems to be a promising way to study human behavior. However, it is very difficult to include all possible permutations and combinations, especially when simulating a large public facility. Thus, one needs to come up with a shortlisted set of personas which will effectively represent the larger data set.
From Personas to Big Data
One other way to collect data specific to each individual is by using tracking devices. This also seems to be the most reliable way to capture different personas when compared with the interviewing method used in the previous study (Kanno et al., 2011). We have investigated some of these methods below in this post.
A lot of human interaction is visually driven as compared to other senses. They say the eyes don’t lie. Eye movement tracking can reveal unbiased data about how people perceive and move through space. Thus, eye tracking can provide a useful insight into human behavioral patterns. There has been extensive work done with this, especially in retail merchandising spaces, where predicting the shoppers’ behavior has proven to increase retail revenue.
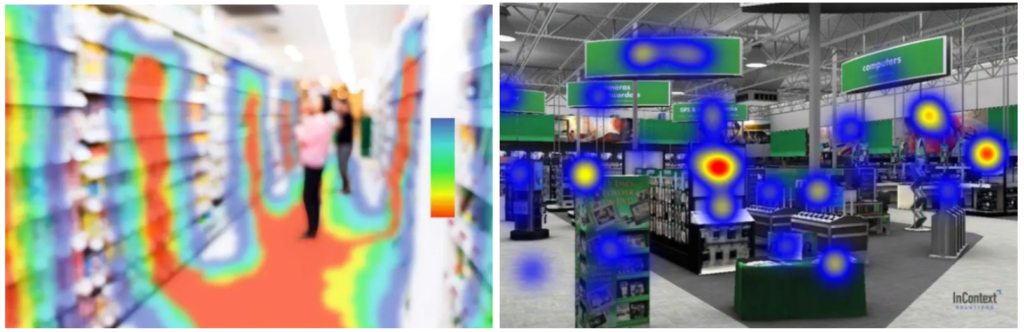
In the left image, customers were tracked using an in-depth sensing camera to generate heat maps. These heat maps reveal information about how long, how many times, and how often a particular product is looked at. This lets the store track how decided/undecided shoppers behave, which kind of packaging or signages are more effective, and if they tend to ignore or focus more on a particular shape, size, color, or even information. The image on the right shows the attention heatmap of where customers are looking when they enter the store. Products placed at the left of center seem to get most of the attention.
In the above case there are two distinct data sets. One is the Behavior Data containing human connectivity and social interactions, while the other is the Big Data containing multiple records and information at micro level, such as pricing, packaging, labels, description, etc. Behavioral Data is not a new concept. Traditionally all companies have been collecting this to forecast patterns. For example, doctor’s offices will collect the family history, while the insurance companies will collect the same to predict future risks. A data set of Behavioral Big Data can be formed by combining these two data sets—Behavior Data and Big Data—which contains rich and integrated information at micro level.
For example, Link Retail* tracks shoppers to create heat maps by employing their AI analytics to inform store layout and utilization. Based on their behavior the shoppers can be further divided into various meaningful personas, including Passerby, Impression, and Dwell categories, for better analysis.
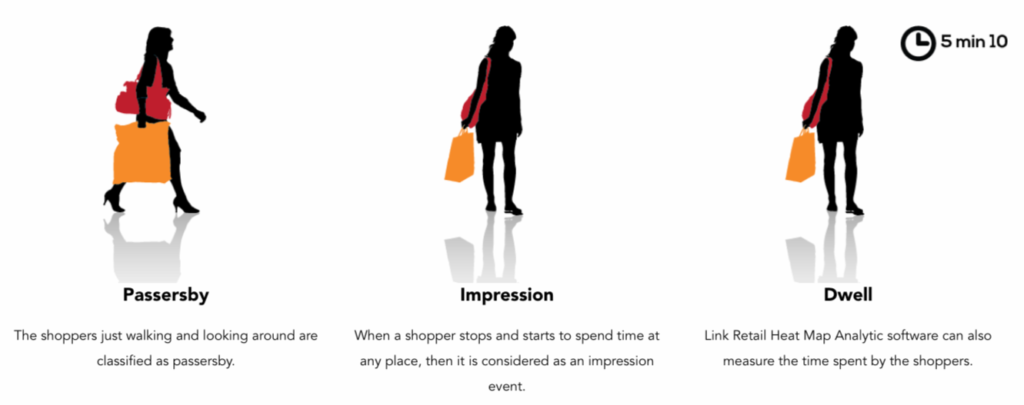
Learning from Product Design
Data collected from the above methods helps designers understand the user needs, experiences, and requirements on an individual level. Similarly to the product design workflow outlined by Lene Nielsen, this data can be used to form a meaningful hypothesis for a given design scenario. In their research, Nielsen et al have broken the personas for UX design objectives into four distinct categories: Goal-directed, Role-based, Engaging, and Fictional personas (Nielsen et al., 2015). And then they have prescribed the following process during product design. A similar process could be adopted for studying human behaviour in building simulations.
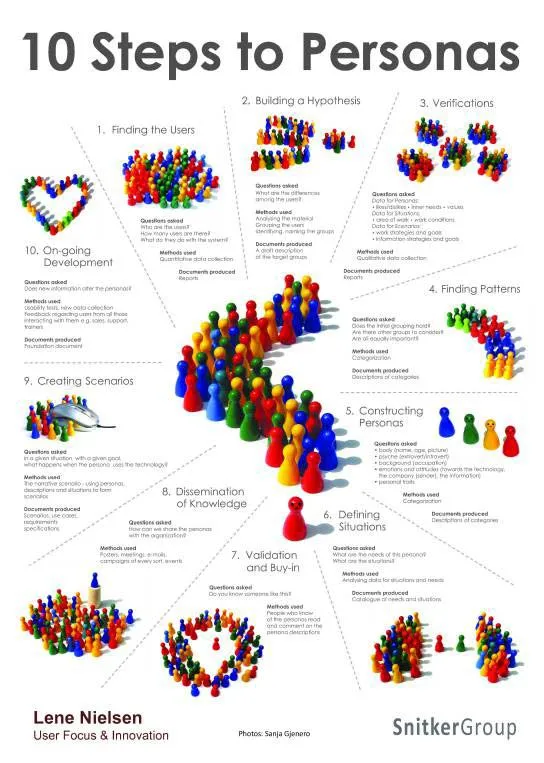
Conclusion
A well-thought out persona is able to paint a picture of a real person in the mind of the designer, making them better able to understand individual behaviors and goals, and thus help simulate actions. These days, Behavioral Big Data is used in predicting future human behavior on several internet and retail platforms and social media apps. Similarly, Behavioral Big Data can also be associated with architecture to predict occupant behavior. For example, the “robot” in our homes that cleans the house knows about the floor plans, and the thermostat and sensors know information about personal behavioral preferences of its occupants. Architects and designers can use these data sets to find patterns and construct personas to personalize their design outcomes and develop a more informed and inclusive building program to suit varied personas occupying the space.
References
Barthet, Matthew, Ahmed Khalifa, Antonios Liapis, and Georgios Yannakakis. “Generative Personas That Behave and Experience like Humans.” FDG ’22: Proceedings of the 17th International Conference on the Foundations of Digital Games, 2022. https://doi.org/10.1145/3555858.3555879.
Chen, Yixing, Xin Liang, Tianzhen Hong, and Xuan Luo. “Simulation and Visualization of Energy-Related Occupant Behavior in Office Buildings.” Building Simulation 10, no. 6 (2017): 785–98. https://doi.org/10.1007/s12273-017-0355-2.
Christofer O. (2014, August 4). Eye-Tracking Heatmaps Track Where Consumers are Looking. AndNowUKnow. https://www.andnowuknow.com/special-report/eye-tracking-heatmaps-track-where-consumers-are-looking/christofer-oberst/42420
Clark, Josh. “Design in the Era of the Algorithm: Big Medium.” Big Medium Full. Accessed December 19, 2022. https://bigmedium.com/speaking/design-in-the-era-of-the-algorithm.html.
Cooper, Alan. The Inmates Are Running the Asylum. Sams, 2015.
Duhigg, Charles. “24. How Companies Learn Your Secrets.” The Best Business Writing 2013, 2013, 421–44. https://doi.org/10.7312/star16075-025.
Duhigg, Charles. The Power of Habit: Why We Do What We Do in Life and Business. Anchor Canada, 2014.
Ekman, Paul, and Wallace V. Friesen. “Facial Action Coding System.” PsycTESTS Dataset, 1978. https://doi.org/10.1037/t27734-000.
Eyeware. (2022, February 21). How to Take Your Shelf and Merchandising Strategy to the Next Level, with 3D Eye Tracking Technology & Data. Eyeware. https://eyeware.tech/blog/data-driven-retail-merchandising/
Gladwell, Malcolm. “Choice, Happiness and Spaghetti Sauce.” Malcolm Gladwell: Choice, happiness and spaghetti sauce | TED Talk. Accessed December 19, 2022. https://www.ted.com/talks/malcolm_gladwell_choice_happiness_and_spaghetti_sauce.
Heat Map – Link Retail | Exclusive In-Store Shopper Behavior Analytics (2021). Link Retail. https://linkretail.com/retail-foot-traffic-how-does-people-counting-work/
Hernandez, Javier, Mohammed E. Hoque, and Rosalind W. Picard. “Mood Meter.” ACM SIGGRAPH 2012 Emerging Technologies on – SIGGRAPH ’12, 2012. https://doi.org/10.1145/2343456.2343471.
Kamvar, Sep, and Jonathan Jennings Harris. We Feel Fine: An Almanac of Human Emotion. Scribner, 2009.
Kanno, T., Ooyabu, T., & Furuta, K. (2011). “Integrating Human Modeling and Simulation with the Persona Method.” Lecture Notes in Computer Science, 51–60. https://doi.org/10.1007/978-3-642-21663-3_6
kllmit. “Kent Larson: Barcelona Smart City Expo World Congress.” YouTube, April 26, 2017. https://www.youtube.com/watch?v=aXFwZZ9cXD0.
Nawyn, Jason, Carson Smuts, and Kent Larson. “A Visualization Tool for Reconstructing Behavior Patterns in Built Spaces.” Proceedings of the 2017 ACM International Joint Conference on Pervasive and Ubiquitous Computing and Proceedings of the 2017 ACM International Symposium on Wearable Computers, 2017. https://doi.org/10.1145/3123024.3123181.
Neal, David T., Wendy Wood, Jennifer S. Labrecque, and Phillippa Lally. “How Do Habits Guide Behavior? Perceived and Actual Triggers of Habits in Daily Life.” Journal of Experimental Social Psychology 48, no. 2 (2012): 492–98. https://doi.org/10.1016/j.jesp.2011.10.011.
Nicol, Fergus & Humphreys, Michael & Olesen, Bjarne. (2004). A stochastic approach to thermal comfort – Occupant behavior and energy use in buildings. ASHRAE Transactions. 110. 554-568.
Nielsen, Lene, Personas. In: Soegaard, Mads and Dam, Rikke Friis (eds.). The Encyclopedia of Human-Computer Interaction, 2nd Ed. Aarhus, Denmark: The Interaction Design Foundation, 2013. https://www.interaction-design.org/literature/book/the-encyclopedia-of-human-computer-interaction-2nd-ed/personas
Pham, Tara. “Know Your Streets – Multimodal Data for Urban Planners & Facilities ManagersTara.” Numina, March 5, 2021. https://numina.co/.
Powering The Future One Step at a Time. (2022). SolePower. http://www.solepowertech.com/#solepower
Reuters Staff, “New Zealand Passport Robot Tells Applicant of Asian Descent to Open Eyes.” Reuters. Thomson Reuters, December 7, 2016. https://www.reuters.com/article/us-newzealand-passport-error/new-zealand-passport-robot-tells-applicant-of-asian-descent-to-open-eyes-idUSKBN13W0RL.
Shalev, Idit, and John Bargh. “On the Association between Loneliness and Physical Warmth-Seeking through Bathing: Reply to Donnellan Et Al. (2014) and Three Further Replications of Bargh and Shalev (2012) Study 1.” Emotion 15, no. 1 (2015): 120–23. https://doi.org/10.1037/emo0000014.
Smuts, Carson. Termites, July 2018. http://termites.synthetic.space/.
TheOasysSoftware. “Oasys MassMotion: Danta Arquitectura – Cendana Apartment Building, Flythrough.” YouTube. YouTube, November 14, 2022. https://www.youtube.com/watch?v=0d0vrcbSN1g.
Vallet, F., S. Hörl, and T. Gall. “Matching Synthetic Populations with Personas: A Test Application for Urban Mobility.” Proceedings of the Design Society 2 (2022): 1795–1804. https://doi.org/10.1017/pds.2022.182.