The project attempts to understand correlations between several relevant factors of today where it aims at identifying the correlations between the mental health data of the city, urban heat island effect, land surface temperature, population, green areas, total neighborhood area, green ratio per neighborhood, NDVI values, distances in 500m to the green spaces, distances in 500m to the water bodies, distances to the industrial buildings. The project then does a space syntax analysis to study the centralities of the identified vulnerable zone to check the distances to the nearby amenities.
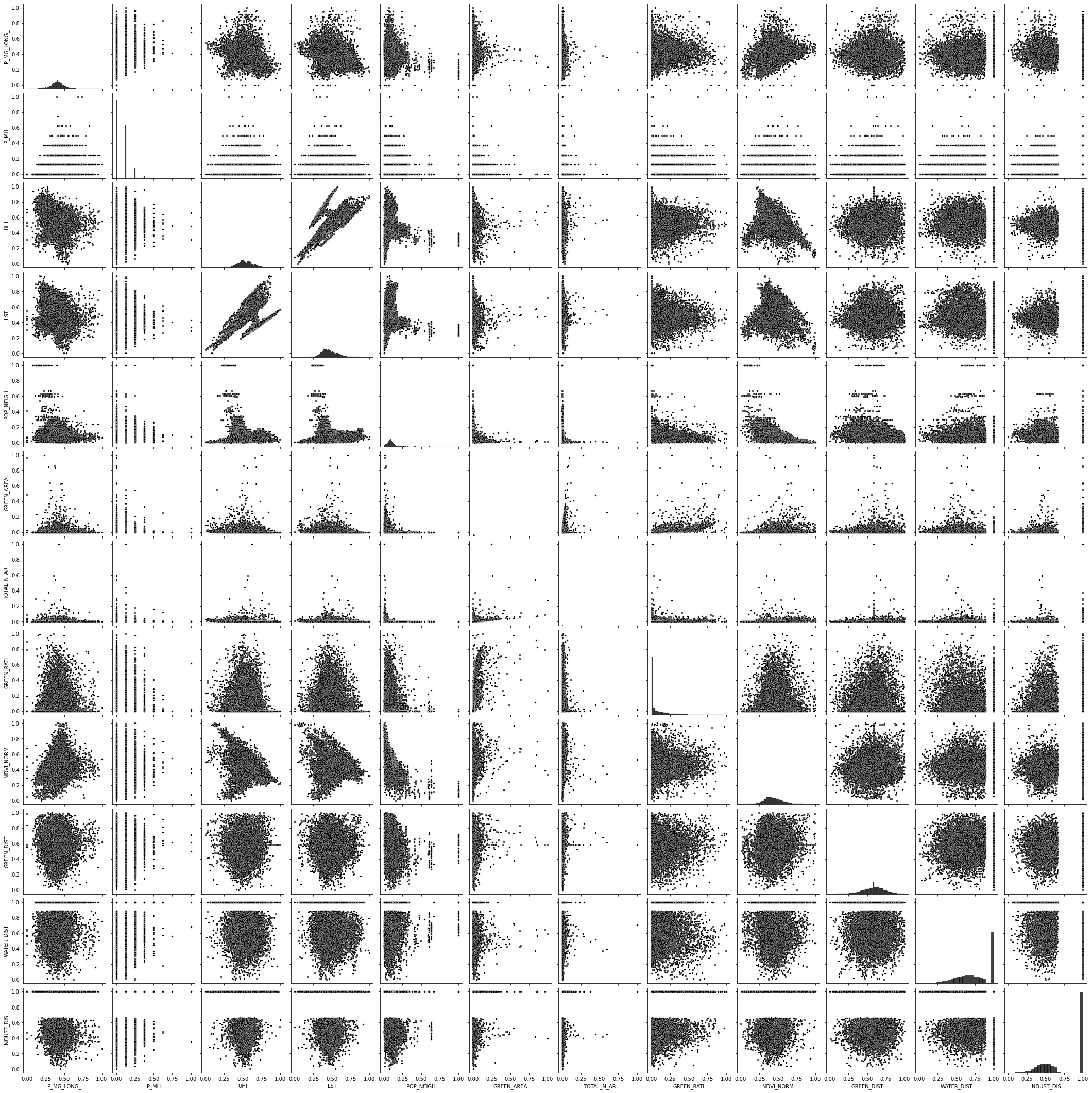
A correlation is a measure that describes the degree of relationship between two variables. A correlation coefficient (r) ranges from -1 to 1. When it is close to 1, it means that there is a strong positive correlation; when it is close to -1, it means there is a strong negative correlation; and when it is close to 0, it means that there is no correlation or very weak correlation.
In this case, the value of ‘LST’ and ‘UHI’ show a positive correlation with a correlation coefficient of 0.63, which means that as the value of ‘LST’ increases, the value of ‘UHI’ also tends to increase. ‘GREEN_RATI’ and ‘GREEN_AREA’ show a positive correlation with a correlation coefficient of 0.52, which means that as the ‘GREEN_RATI’ increases, the ‘GREEN_AREA’ also tends to increase. For all other combinations of variables, the correlation coefficient is close to 0 (ranging from -0.48 to 0.47), indicating that there is no or very weak correlation. This means that changes in one variable are not necessarily associated with changes in the other variable. For example, ‘P_MH’ and ‘P_MG_LONG_’ have a correlation coefficient of 0.20, which is close to zero and hence, suggests that there is no correlation or very weak correlation. It means that ‘P_MH’ and ‘P_MG_LONG_’ tend to change independently of each other. The presence or absence of correlation between variables does not imply causation. Just because two variables move together does not mean that one variable’s movement causes the other’s movement. It only means that they tend to move together. Other factors may be influencing both variables.
So, in this analysis, only ‘LST’ and ‘UHI’, and ‘GREEN_RATI’ and ‘GREEN_AREA’ show a significant correlation, while all other combinations of variables show no or very weak correlation.
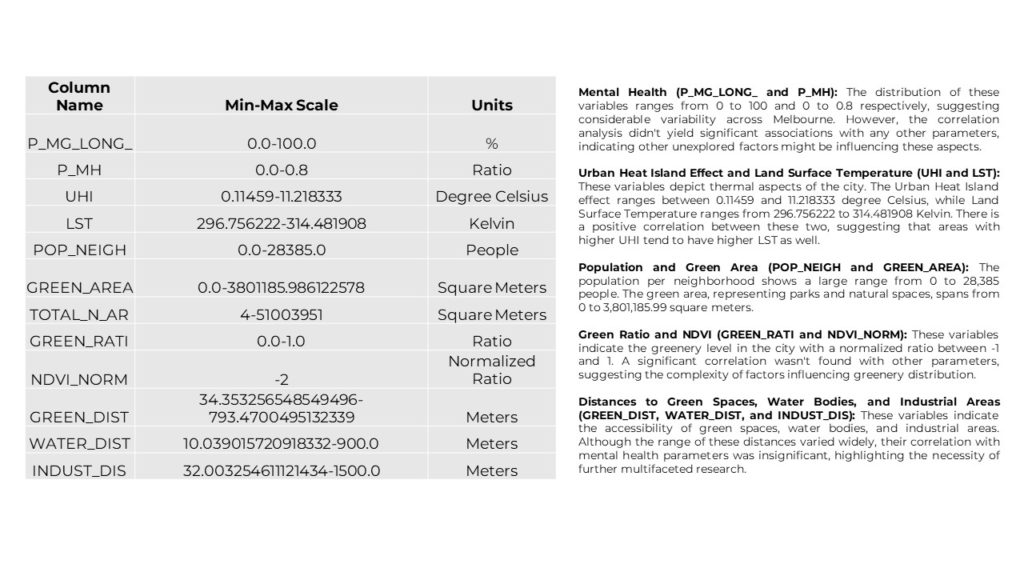
K-Means Clustering of Neighborhoods
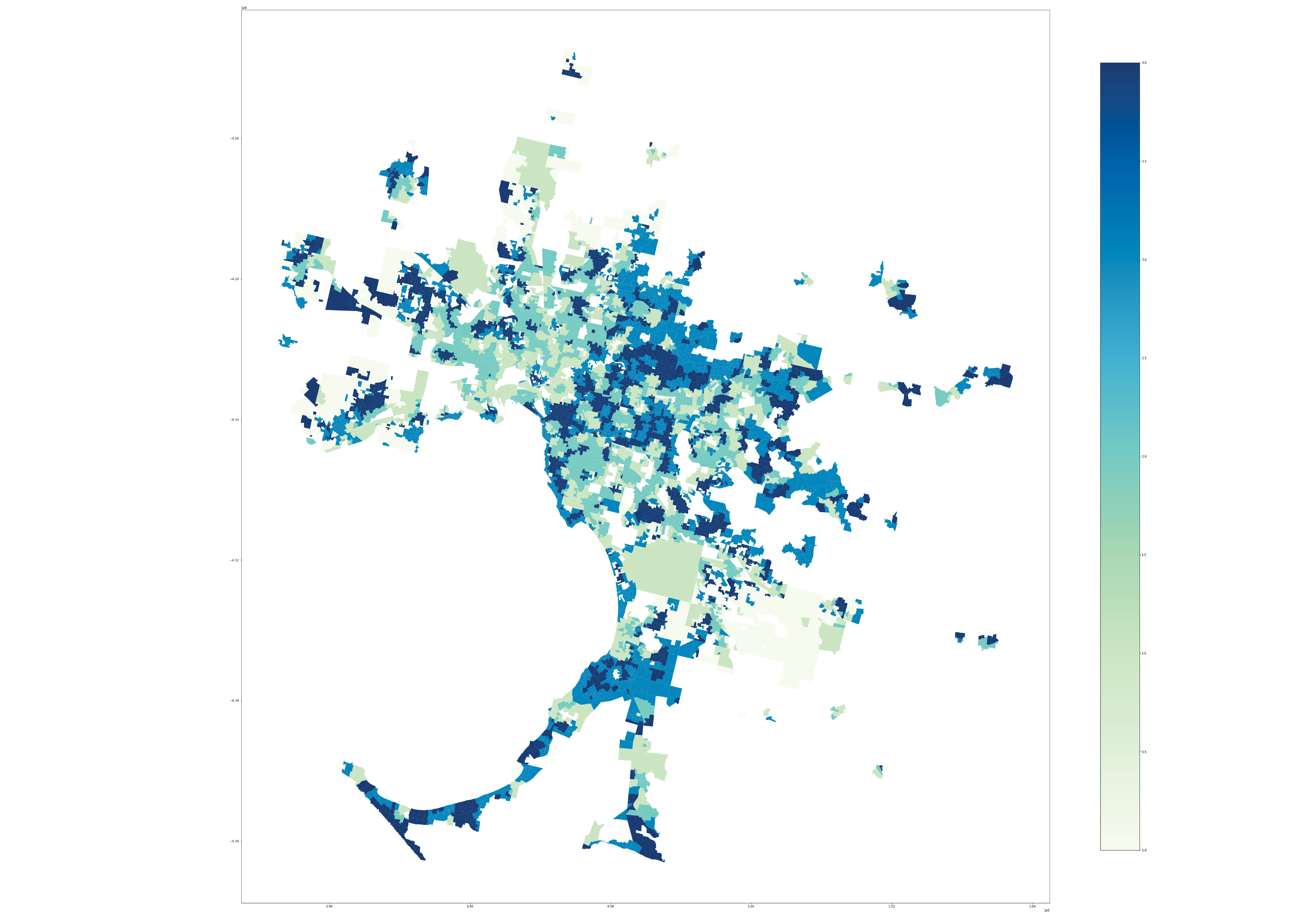
Silhouette method to determine the number of clusters
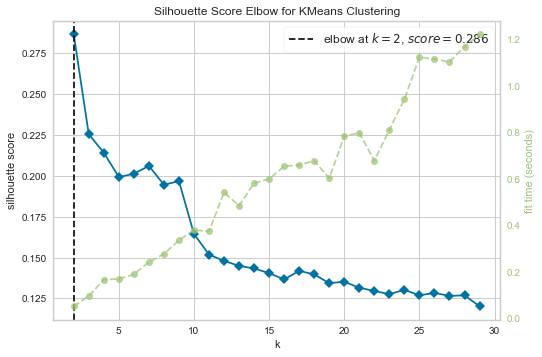
K-Means Clustering with 2 clusters from the Silhouette Score
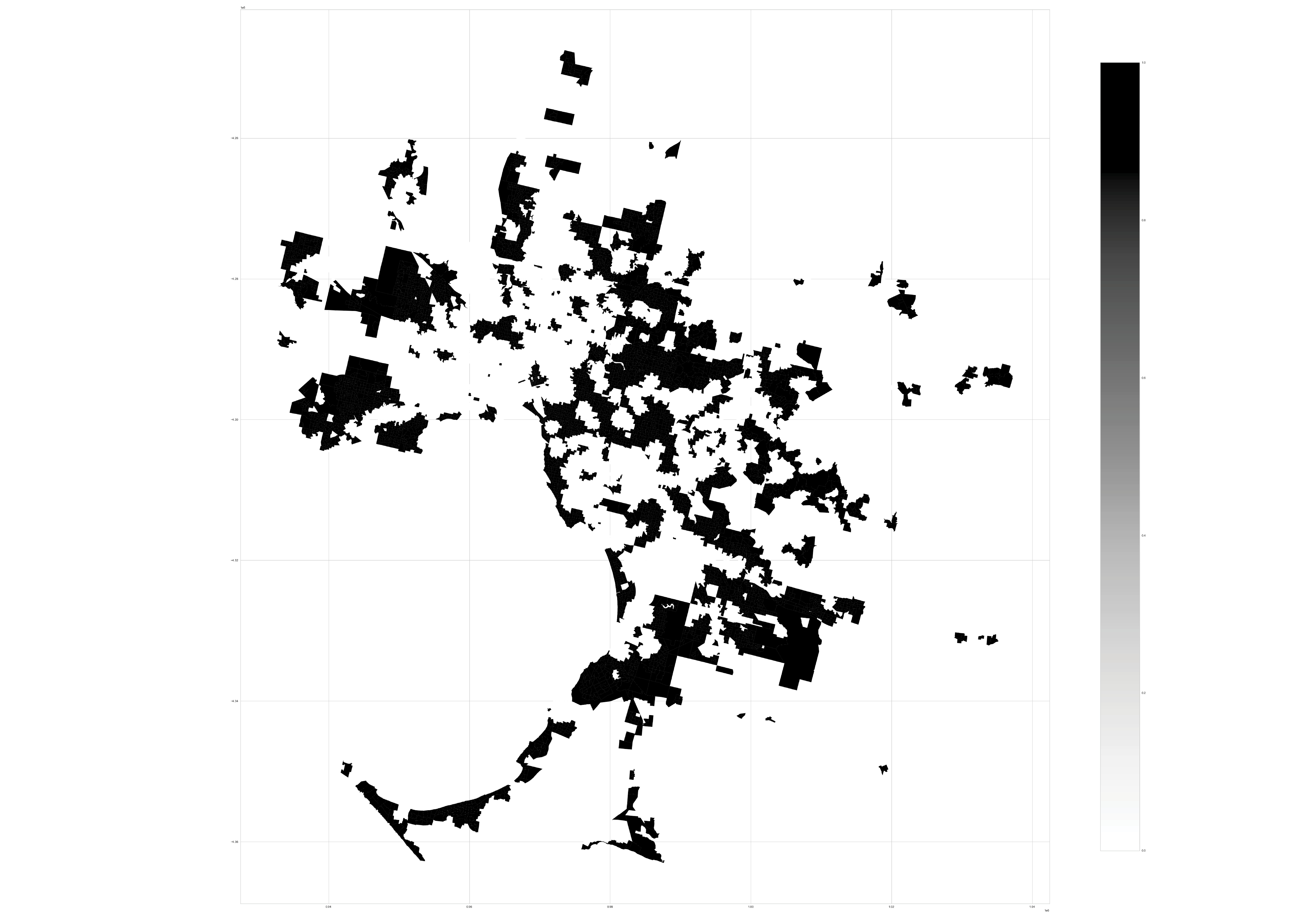
Understanding the highest average values of the variables from the factors considered
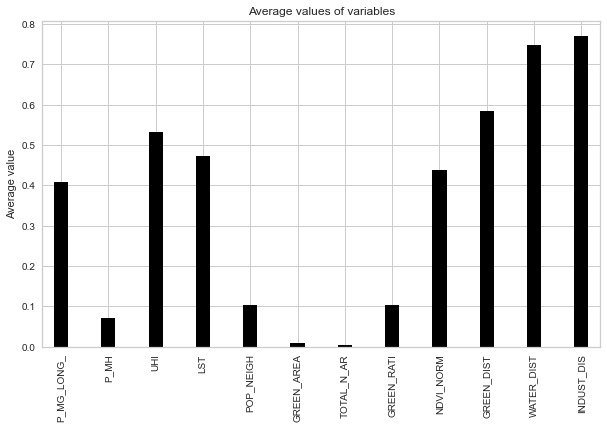
Checking for correlation between the wighted values of the variables and the clusters
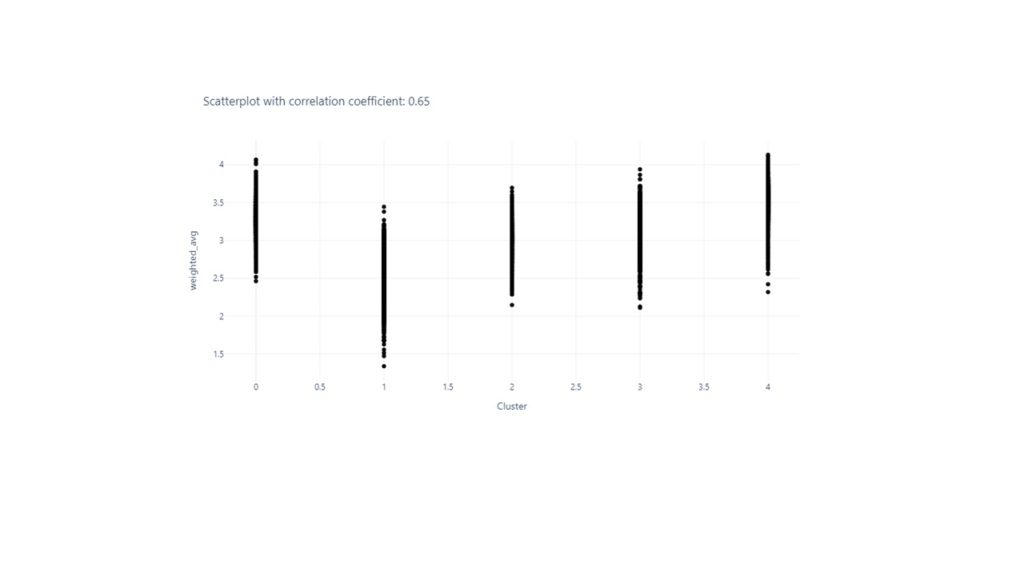
Map of the neighbourhoods based on the weighted average highest sum values
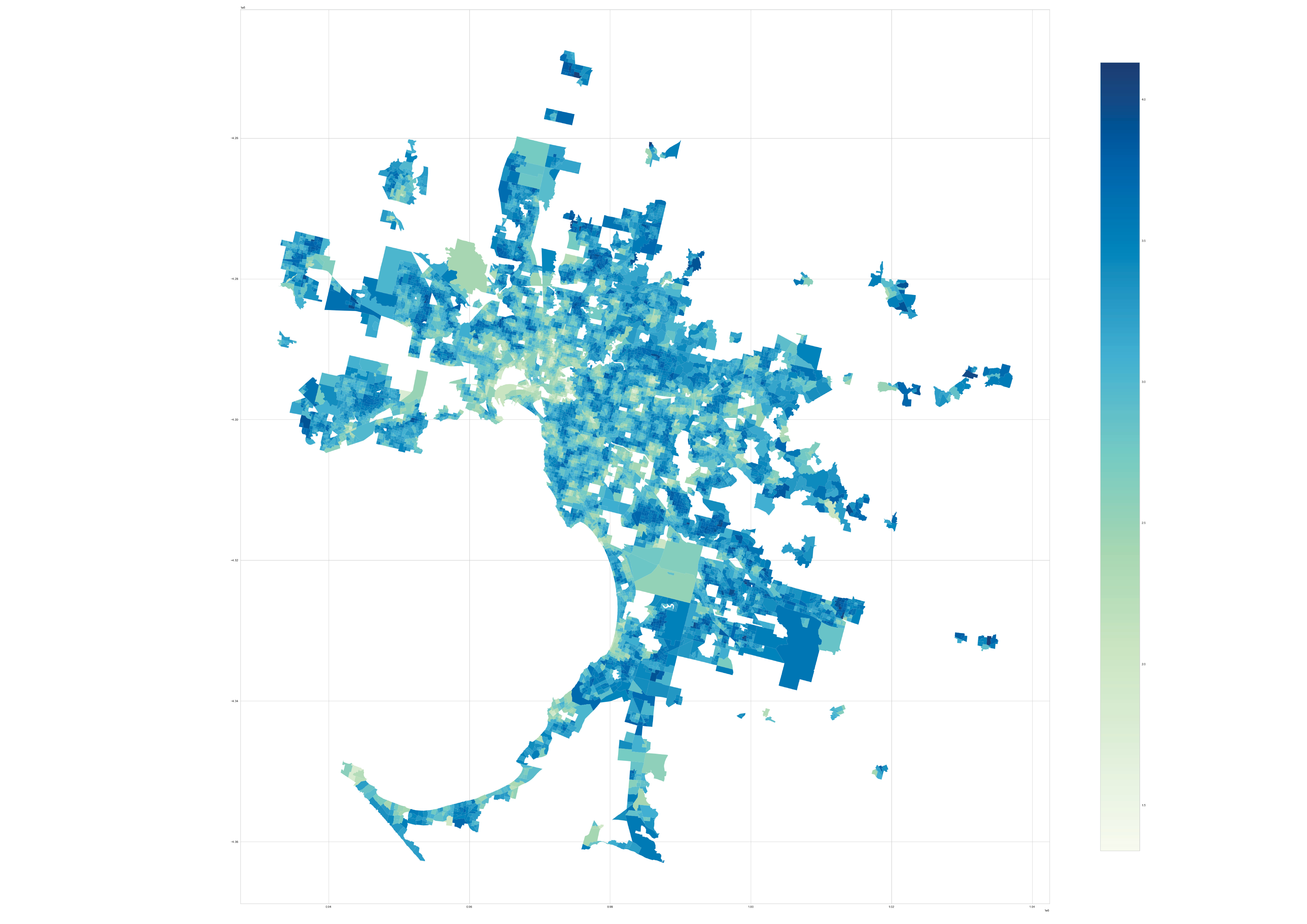
Top 100 vulnerable neighborhoods– The weighted average of the sum of highest values
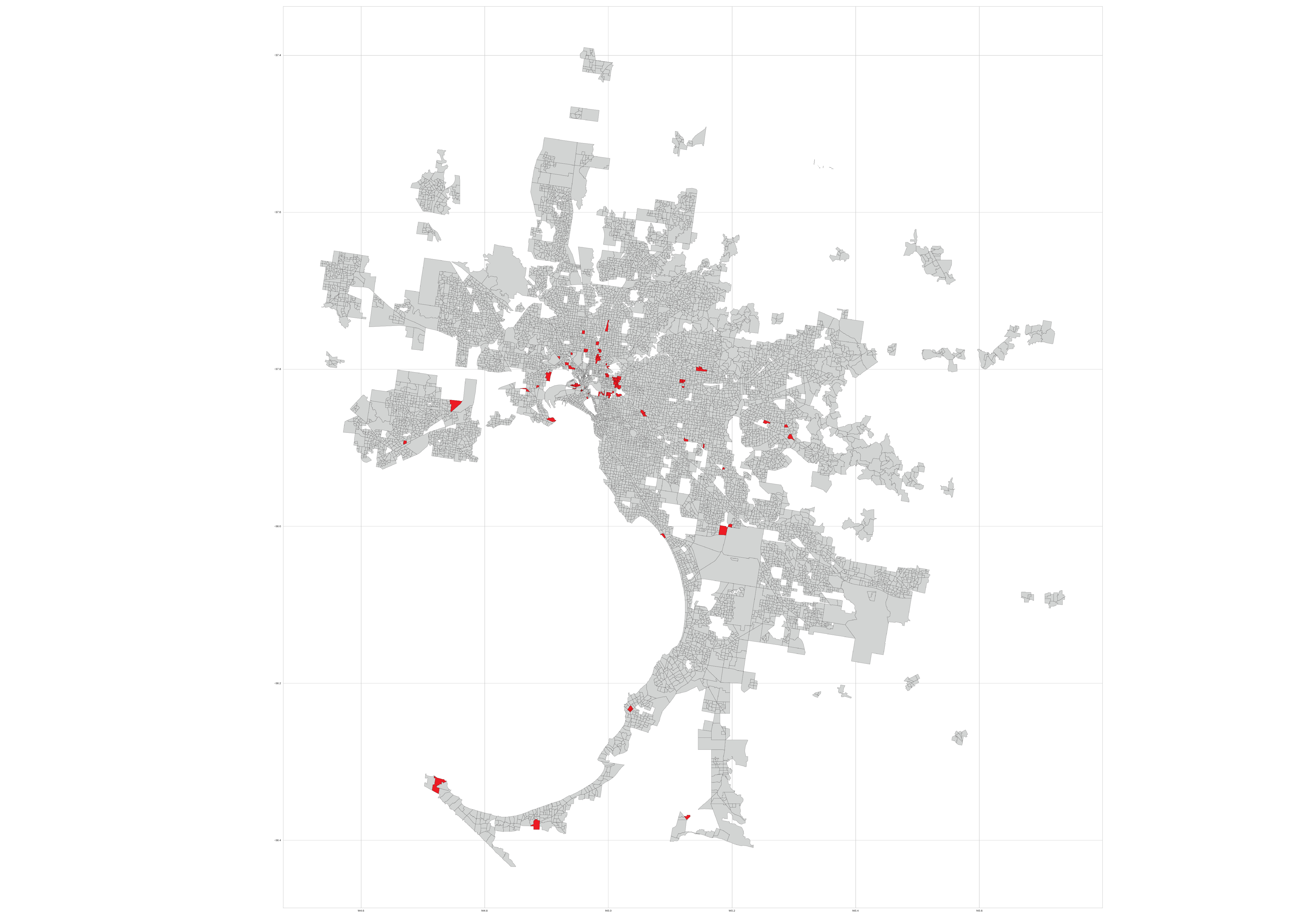
Zooming in to the first vulnerable neighbourhood with coordinates: (-37.821444, 144.942956)
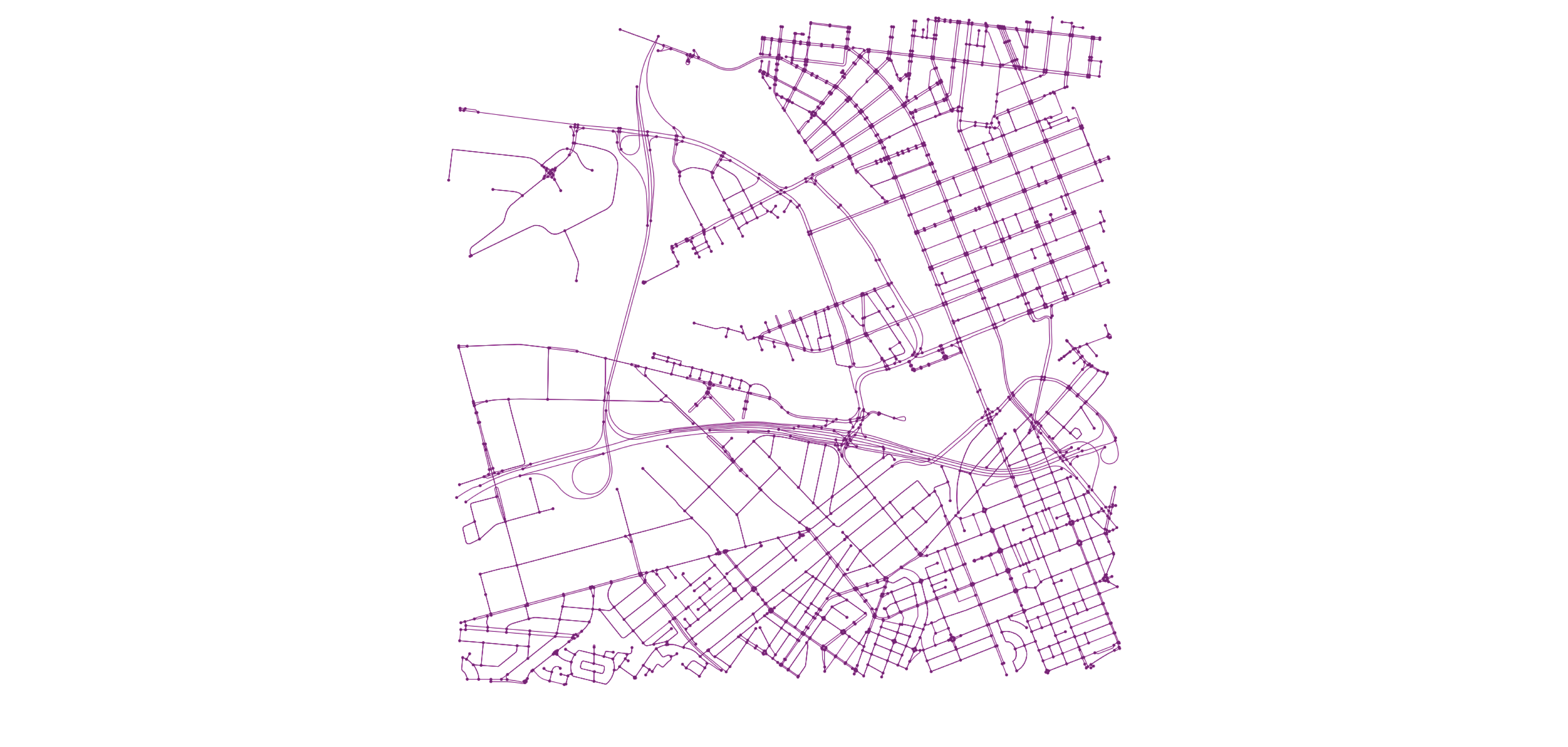
Mapping to find the shortest path from the centre points of the streets to the amenities
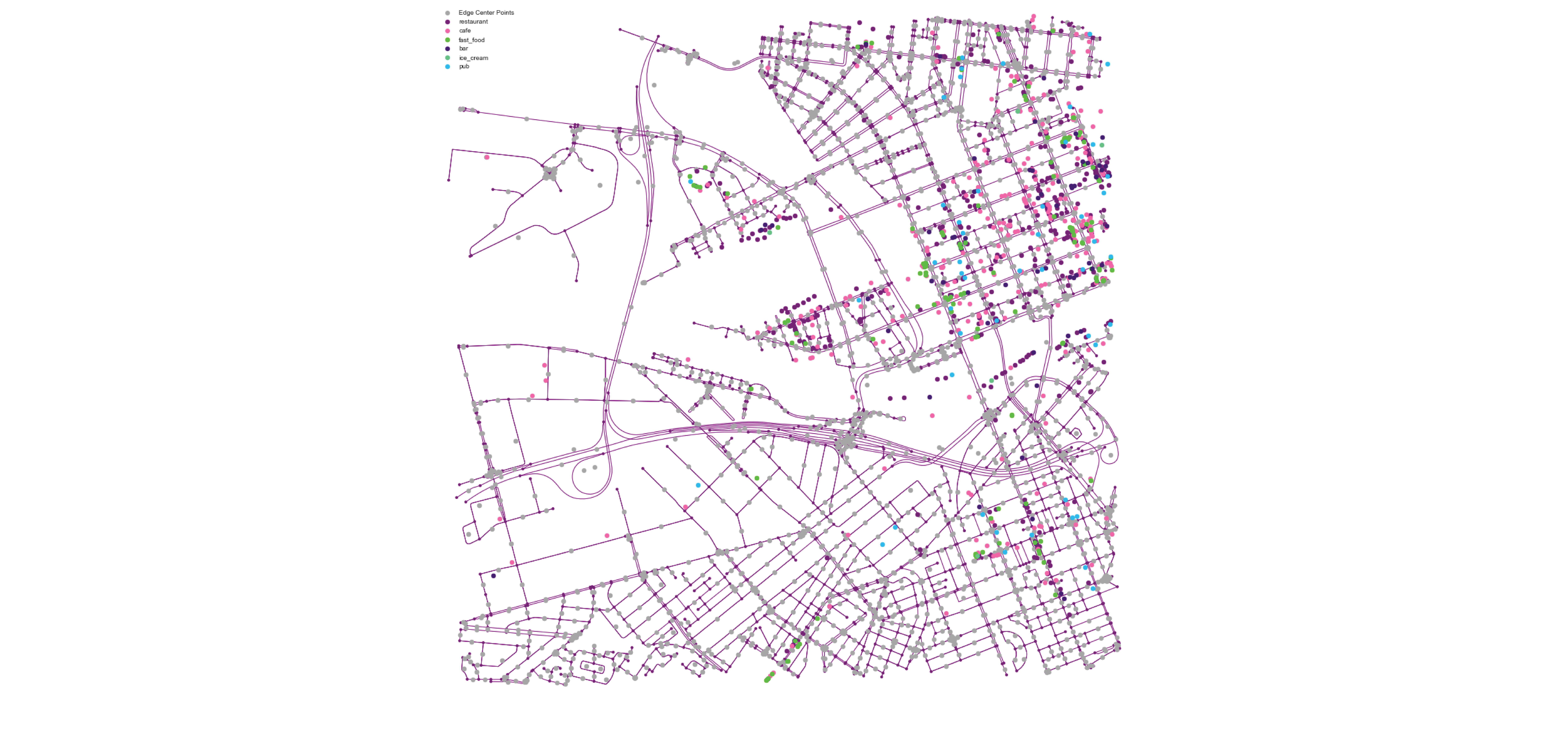
Betweenness centrality to understand the nodes of highest centrality in termsof the shortest paths
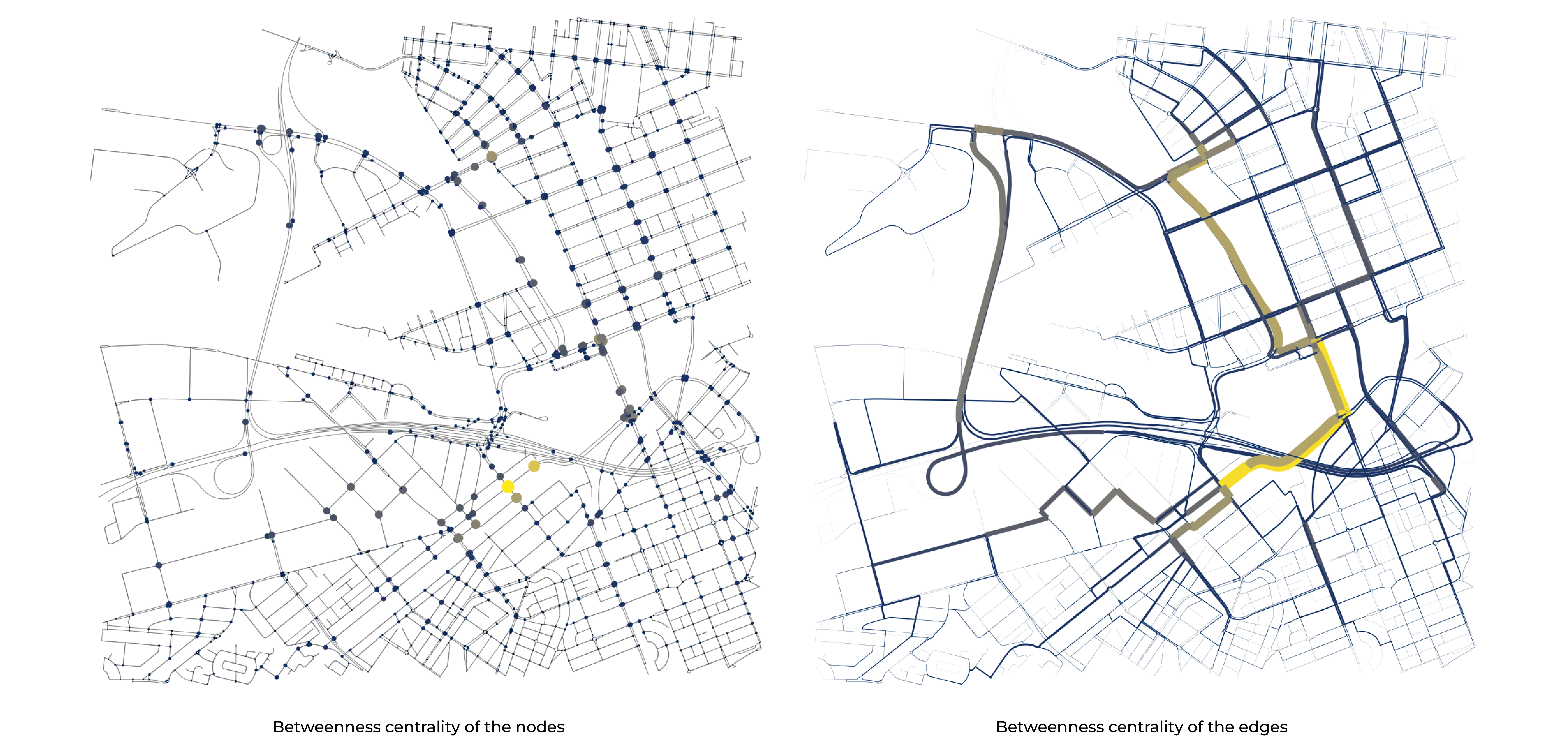
Closeness centrality to understand the nodes of centralities in terms of the immediate shortest path with the rest of the paths
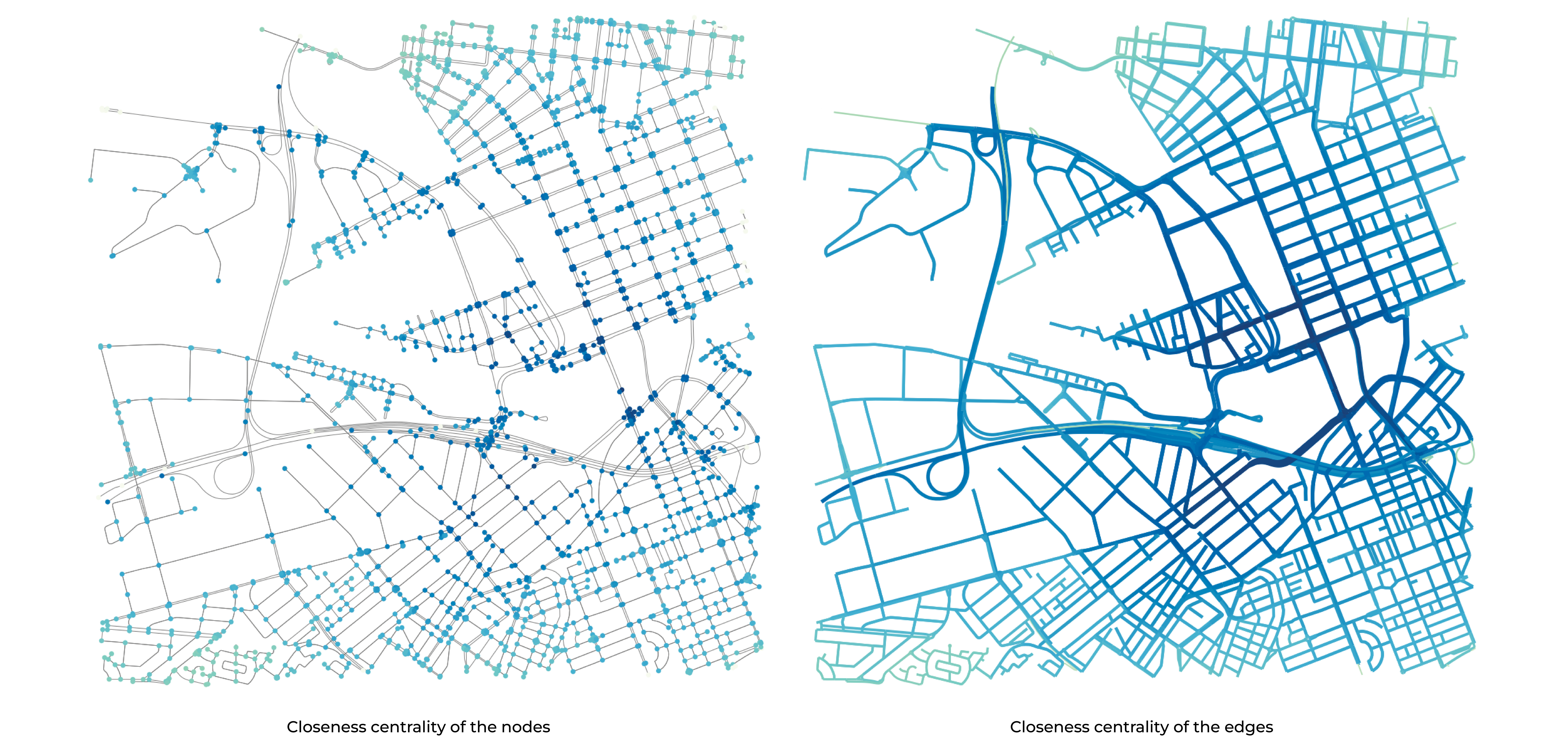
Degree centrality to understand the nodes of centralities with highest connectivity
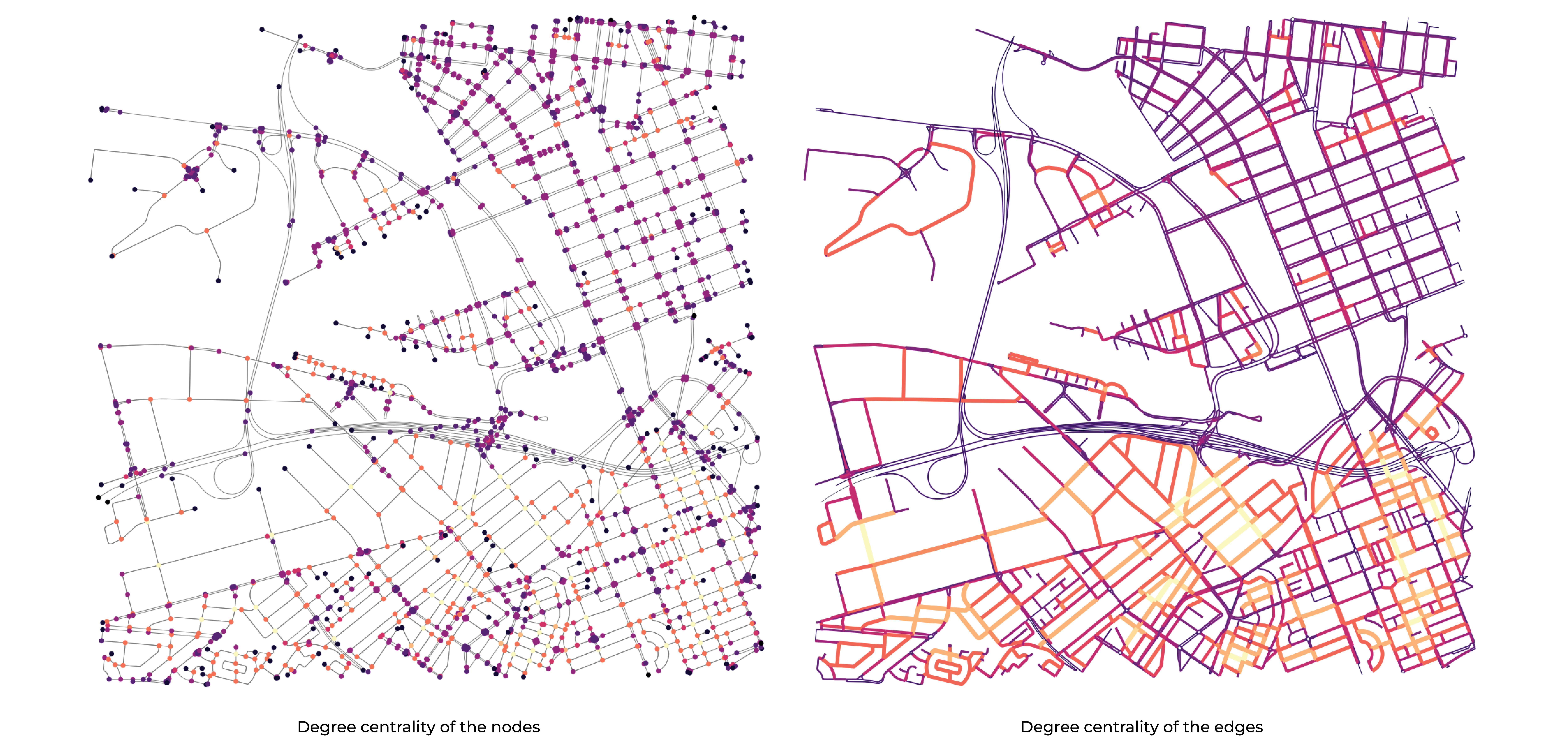
Correlations between Betweenness Centrality, Closeness Centrality and Degree Centrality
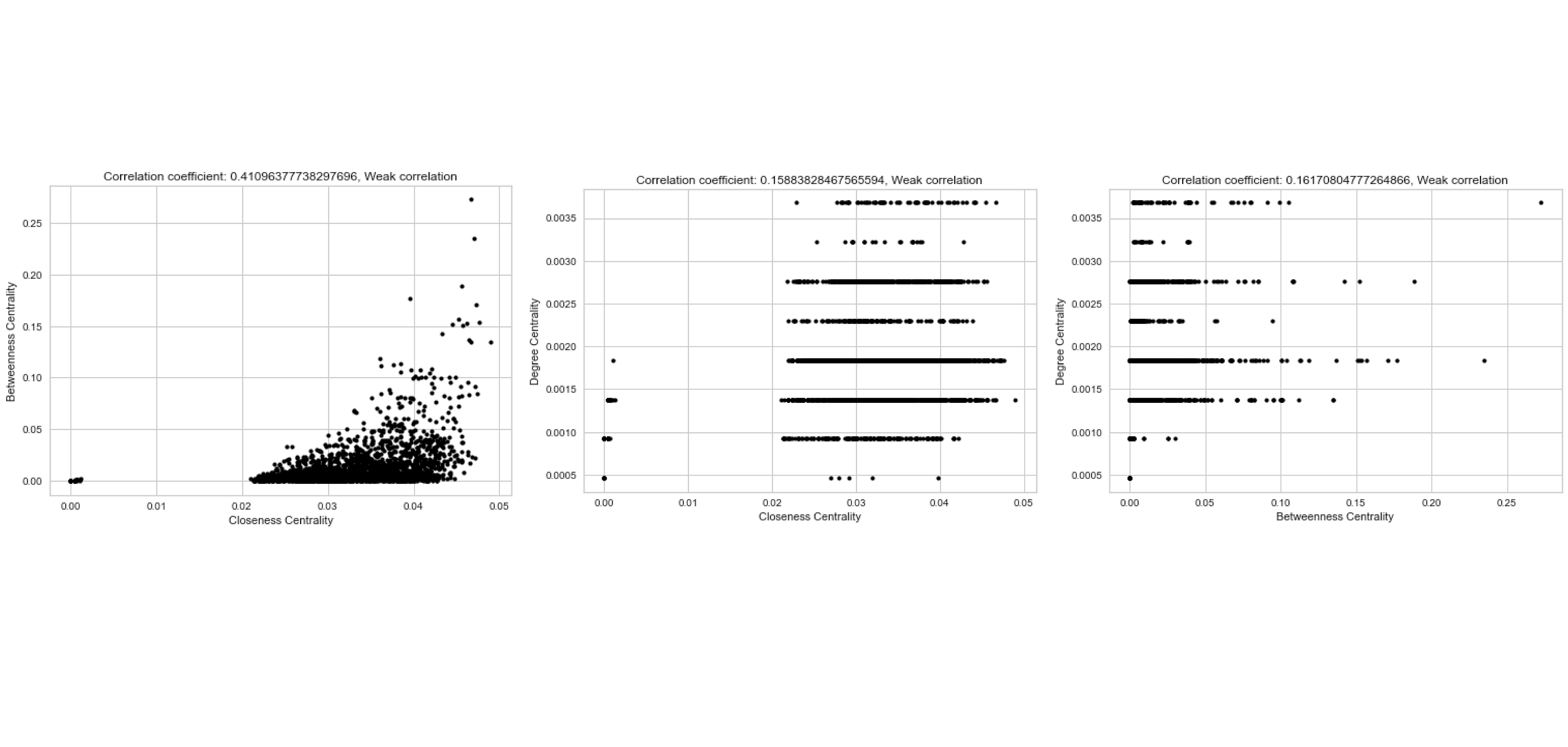
Further Steps of Analysis
To find the shortest path between the center points of the street segments to each of the amenities.
To find the shortest path between significant the node centralities from the betweenness centrality, closeness centrality and the degree centrality to each of the amenities.
To check if the distances in the above two steps are within the walking range of 500m-800m by performing an isochrone analysis.