Abstract
Parametric design tools like Grasshopper empower architects to create adaptive and efficient spatial layouts, but their complexity presents a barrier for non-experts. This research proposes a community-driven design tool that leverages Graph Neural Networks (GNNs) and Large Language Models (LLMs) to learn from expert-authored Grasshopper scripts, enabling prompt-based parametric layout design. By encoding design logic as graph structures, GNNs capture relationships between architectural components, while LLMs interpret user prompts to generate adaptable solutions.’
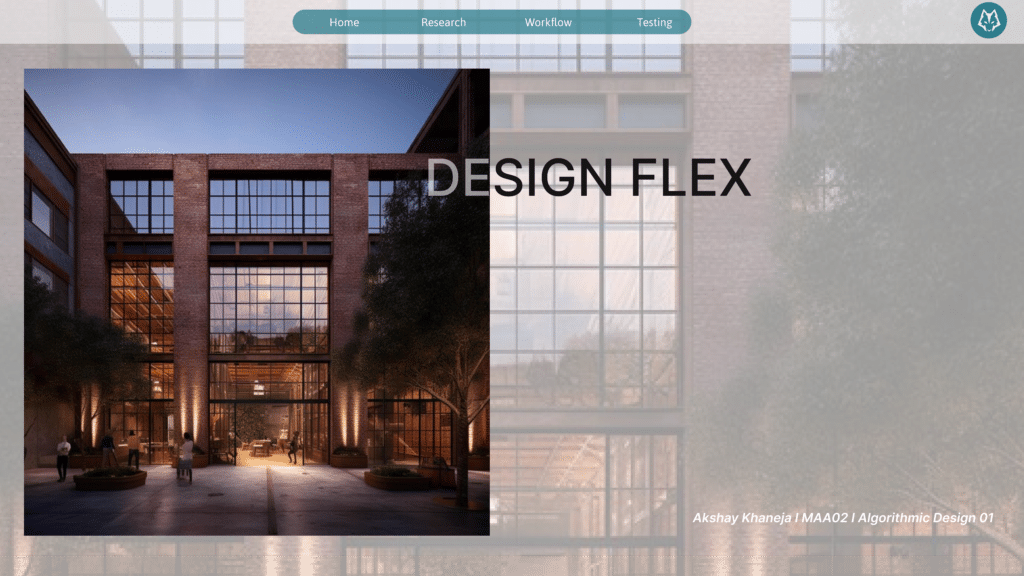
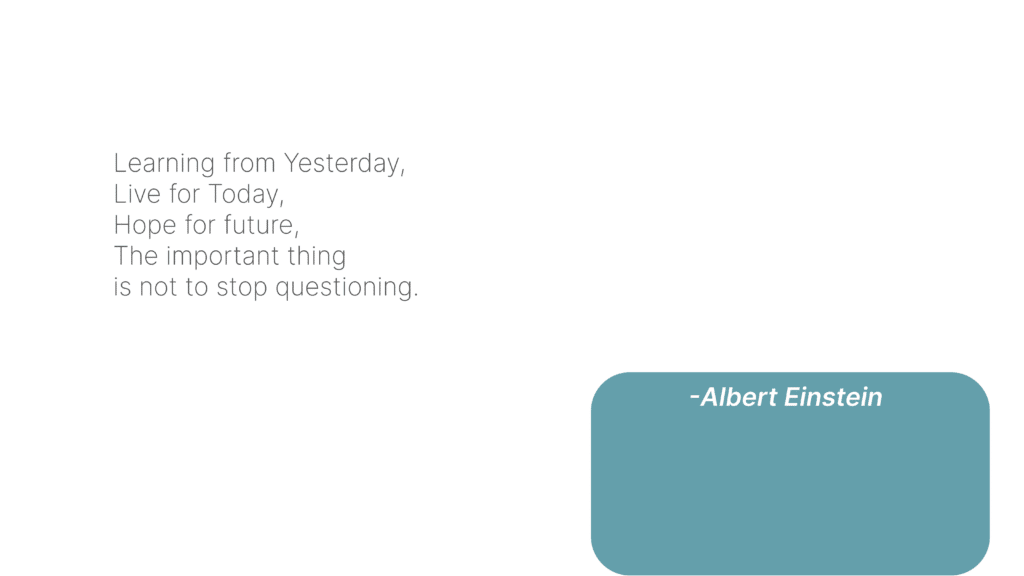
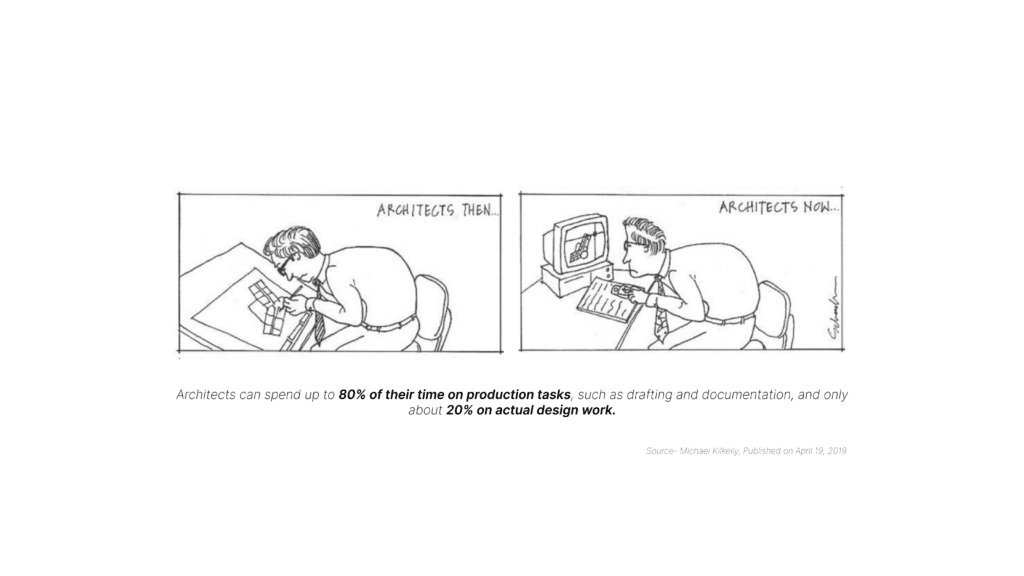
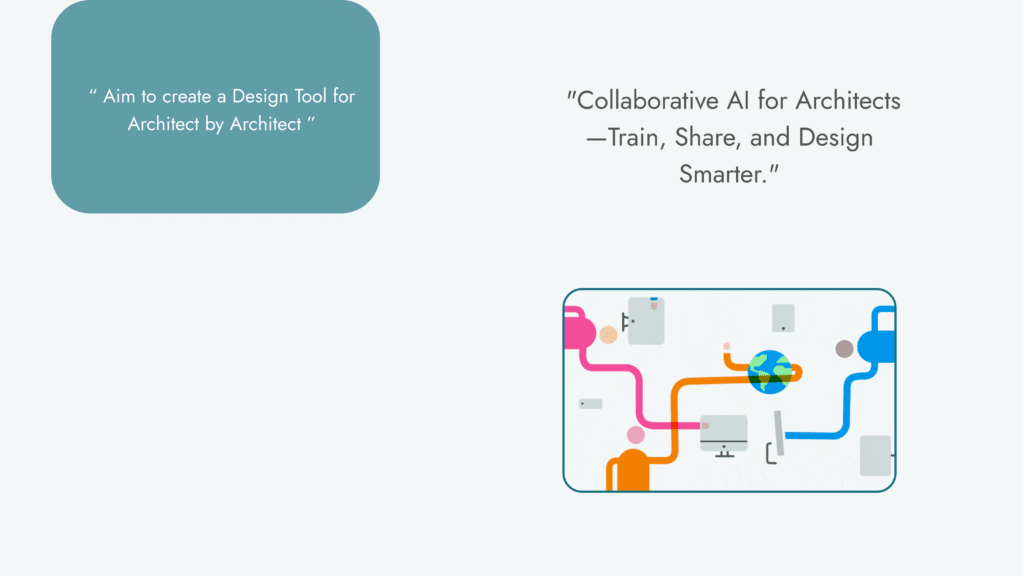
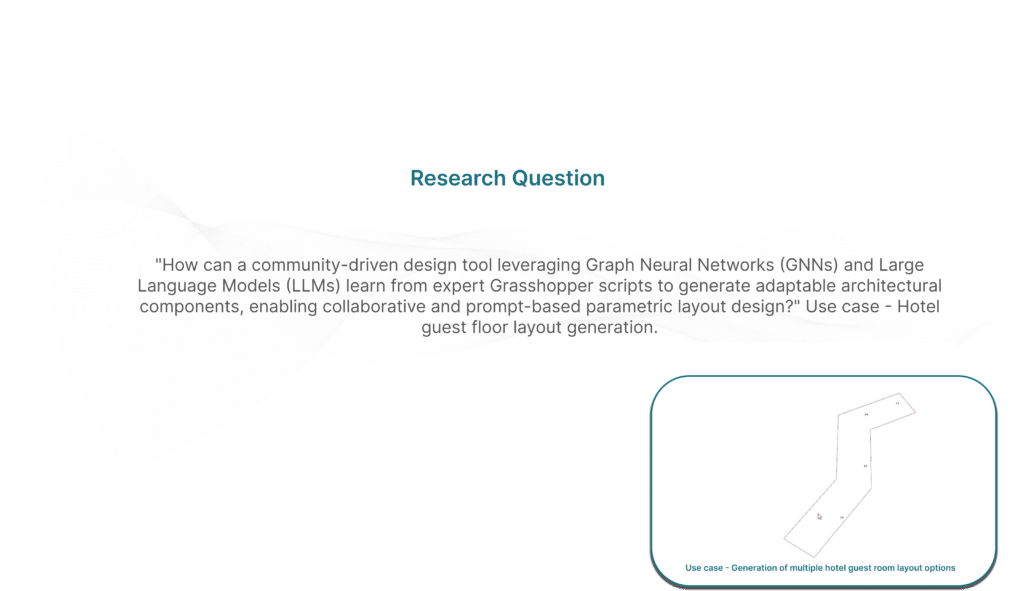
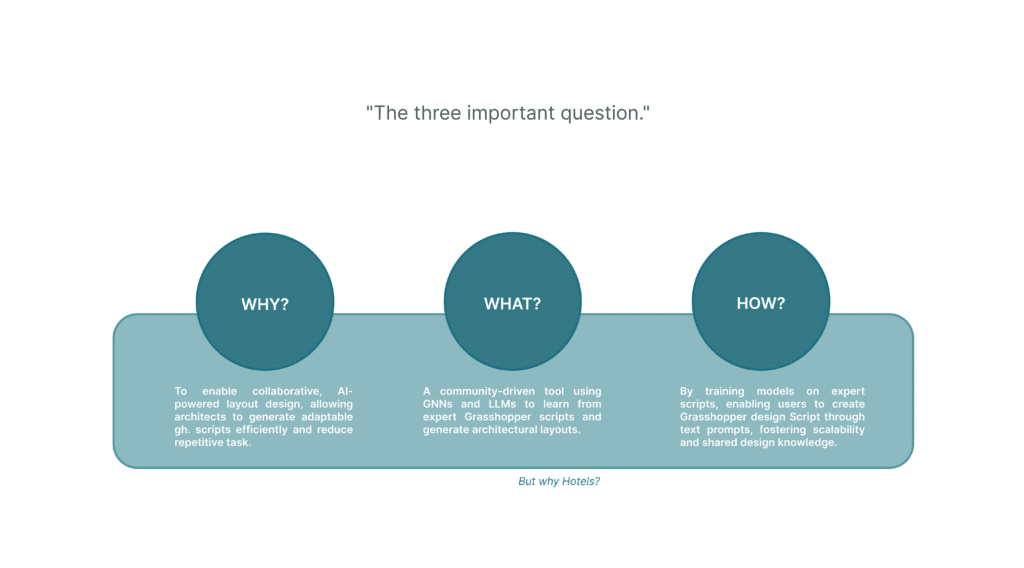
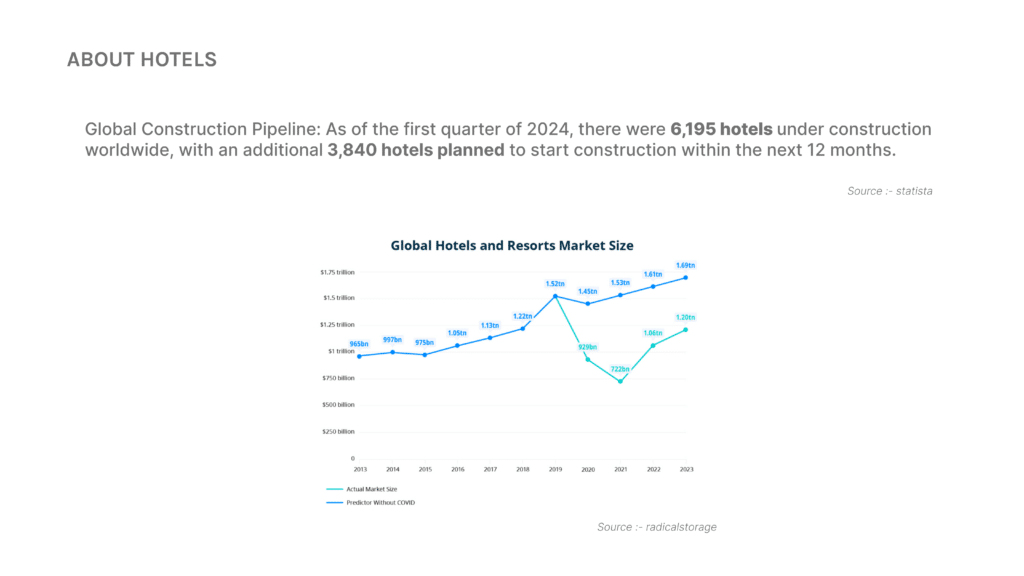
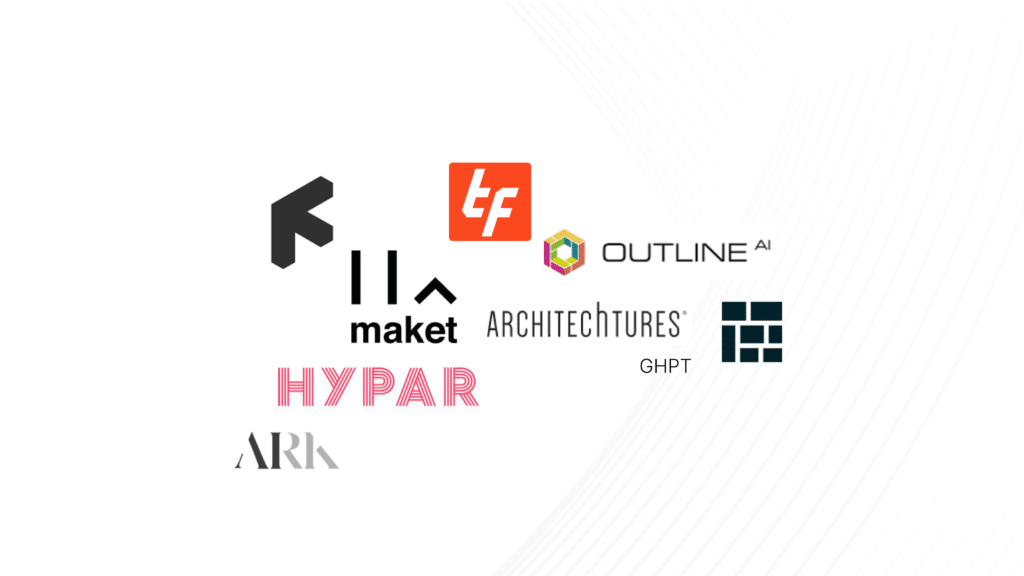
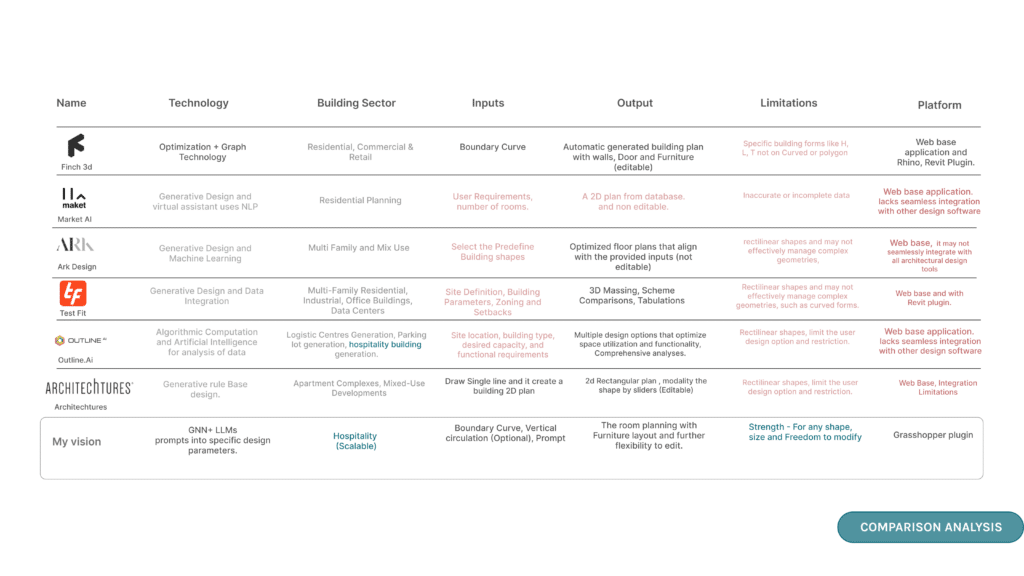
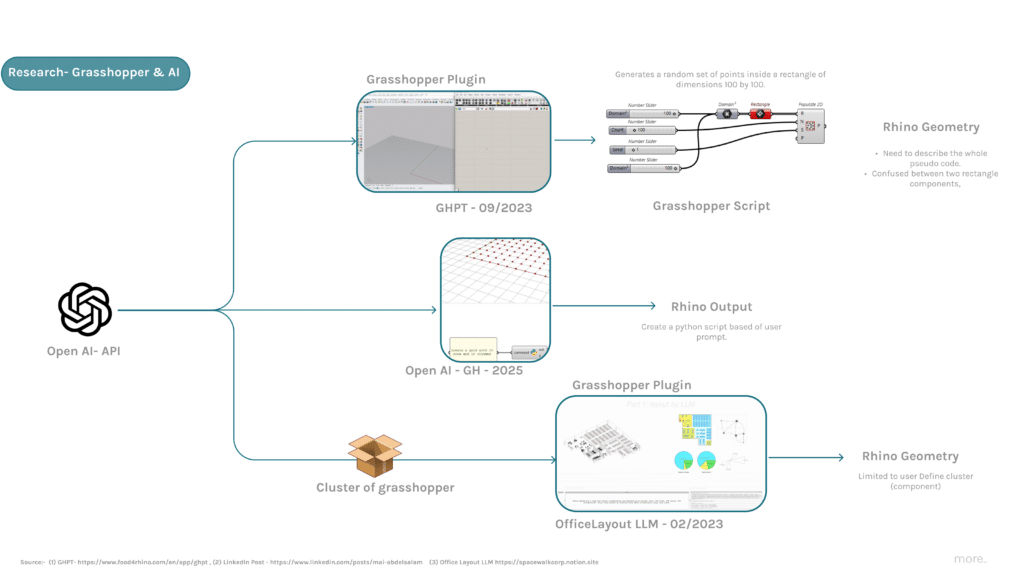
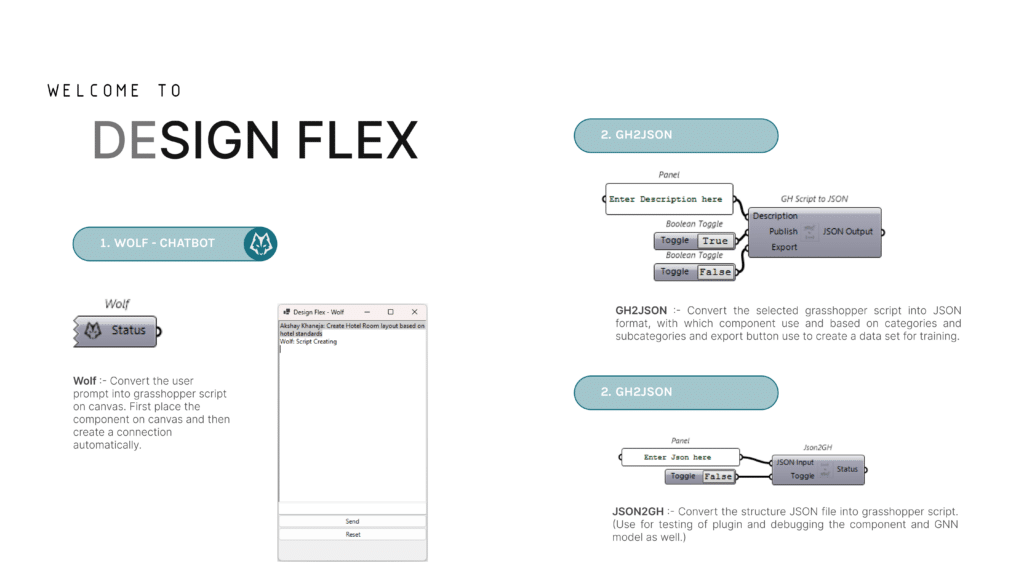
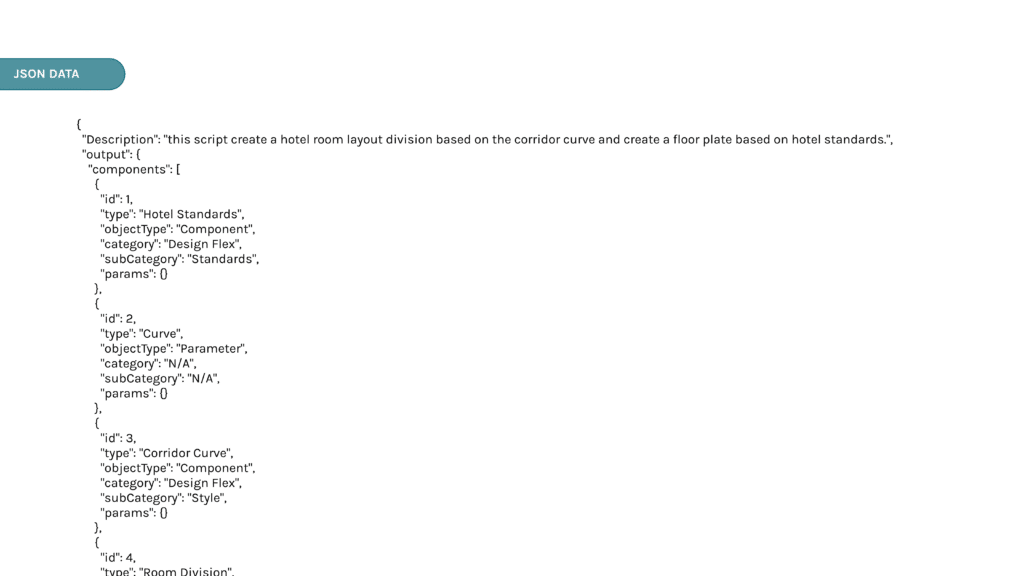
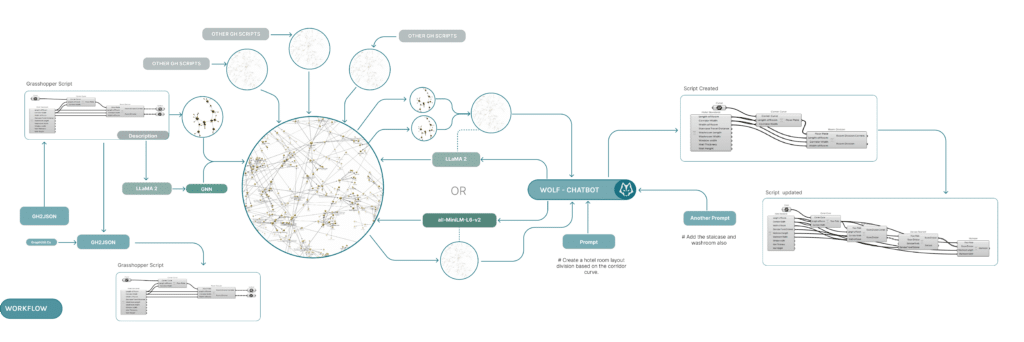
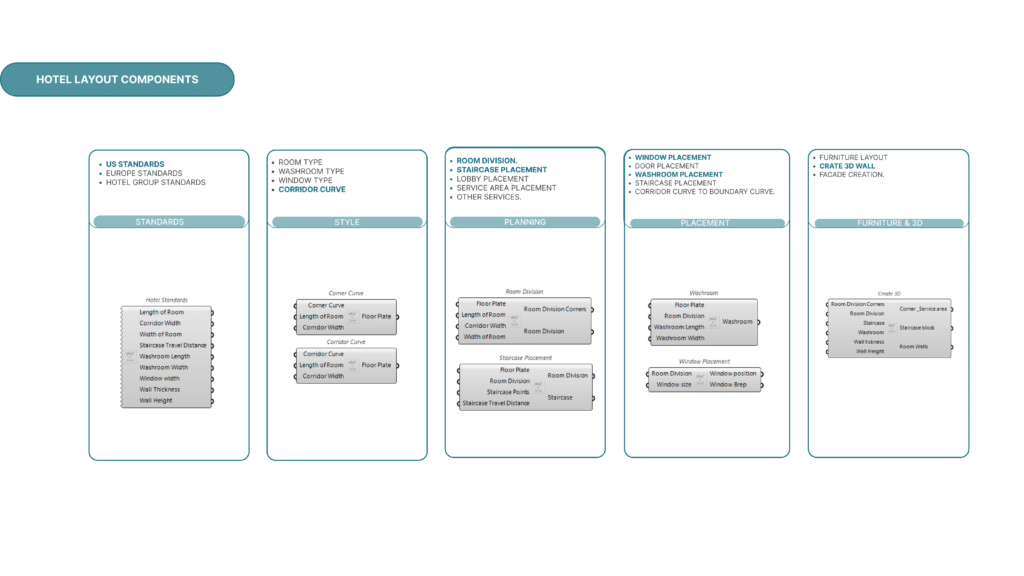