TIMBER SENSING TECHNOLOGY
A material system combining timber and graphene, replacing traditional sensors through graphene’s sensing capabilities. This innovative approach allows first, real-time tracking of deformations inside the wood. Second, creating a digital twin that improves maintenance efficiency in the operational phase. Third, providing a detailed service life passport, this system supports reuse and reduces classification time and processes after disassembly, extending the lifecycle of timber structures.
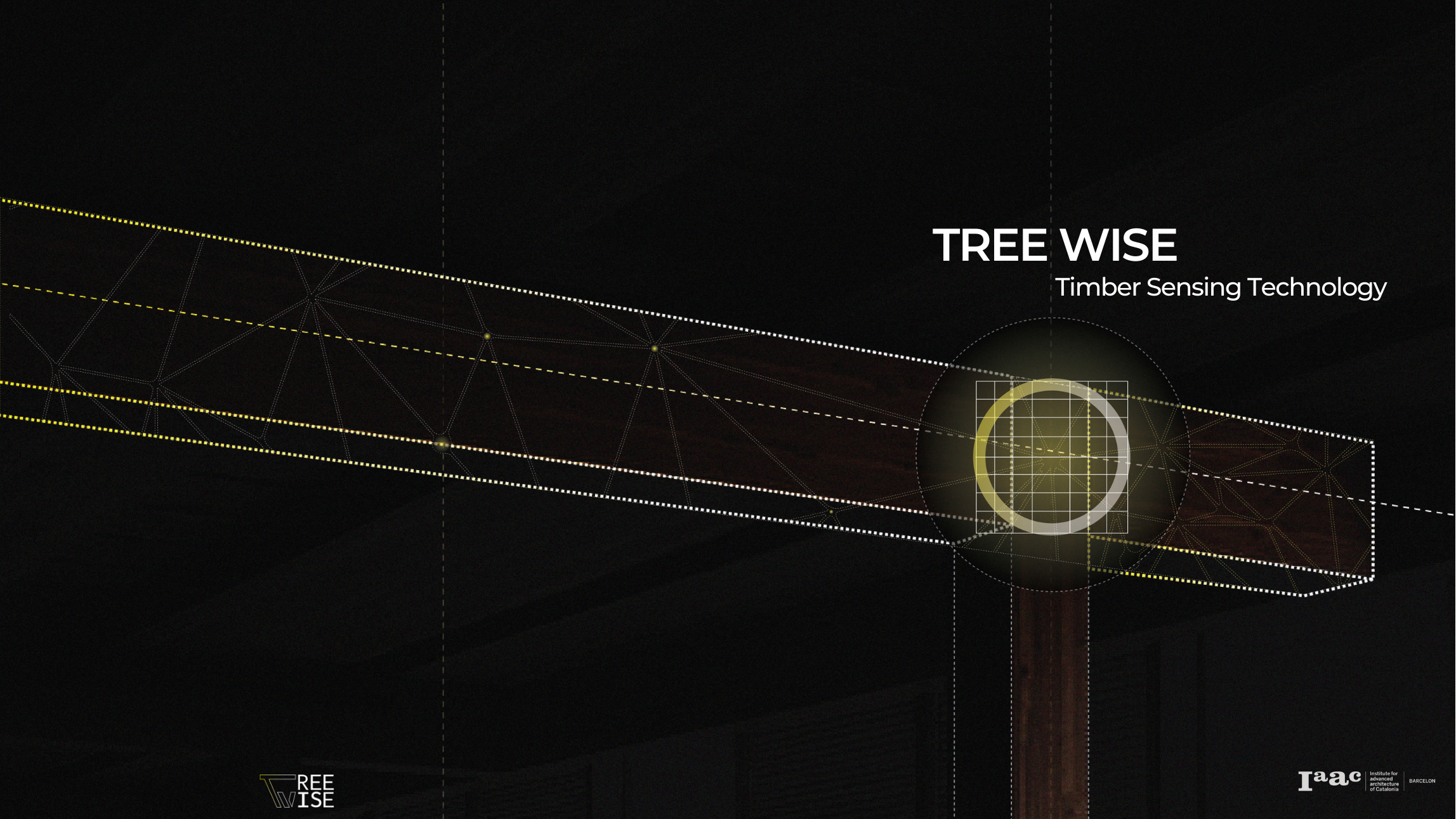
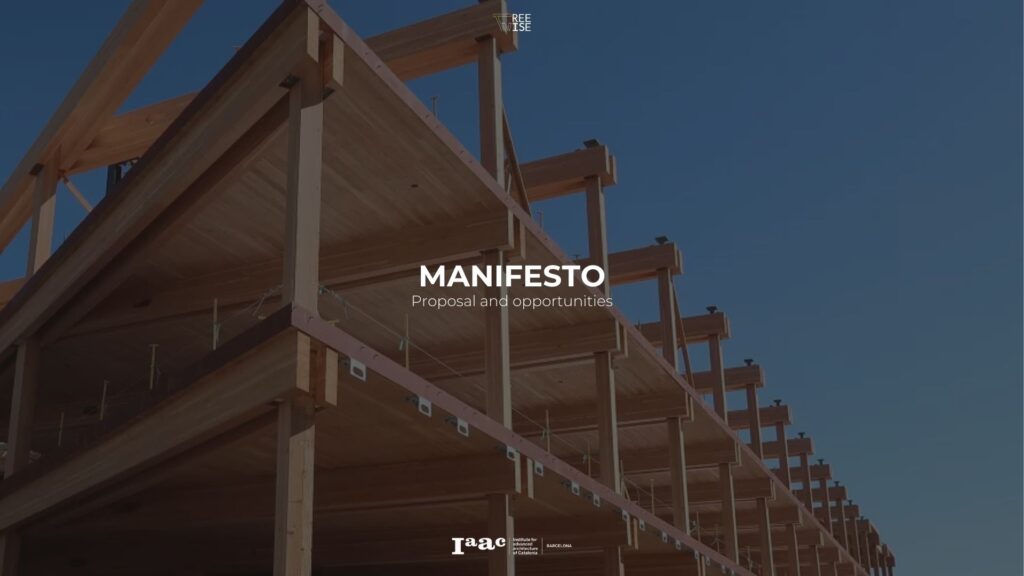
Wood is a highly versatile biomaterial that can be sustainably sourced from nature, used in construction to take advantage of its carbon sequestration capabilities, and then be returned to the soil at the end of its lifespan. To decrease the CO2 emissions caused by the construction industry mass timber has potentially grown relying on engineered timber or glue laminated timber to build.
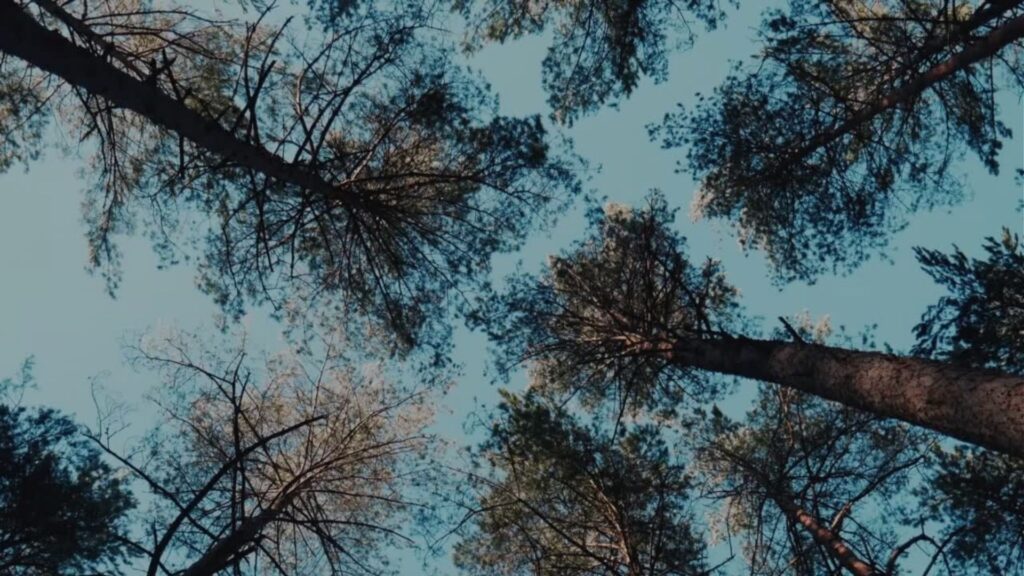
Great advancements in construction technologies have been achieved in both architecture using standardized engineered timber components and in free-form architecture with customized components and complex geometries.
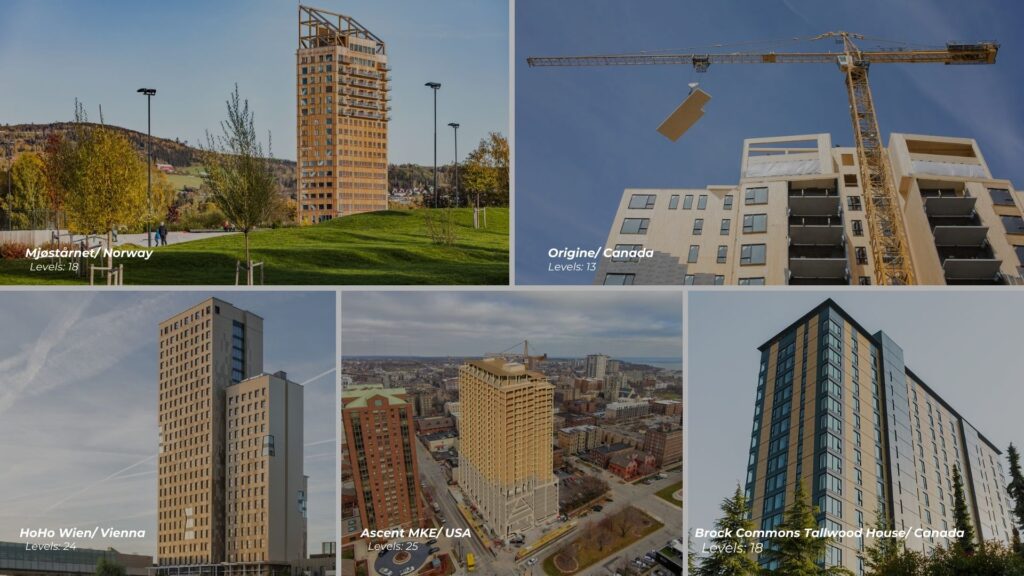
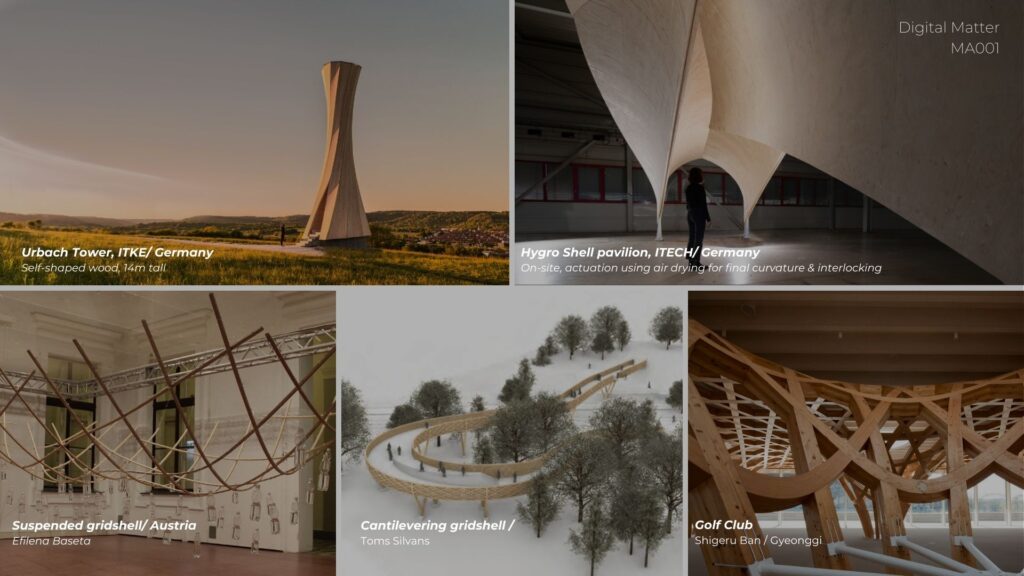
The mass timber industry is expected to continue its exponential growth in the next years, not just in residential buildings but with skyscraper and even wooden cities projections
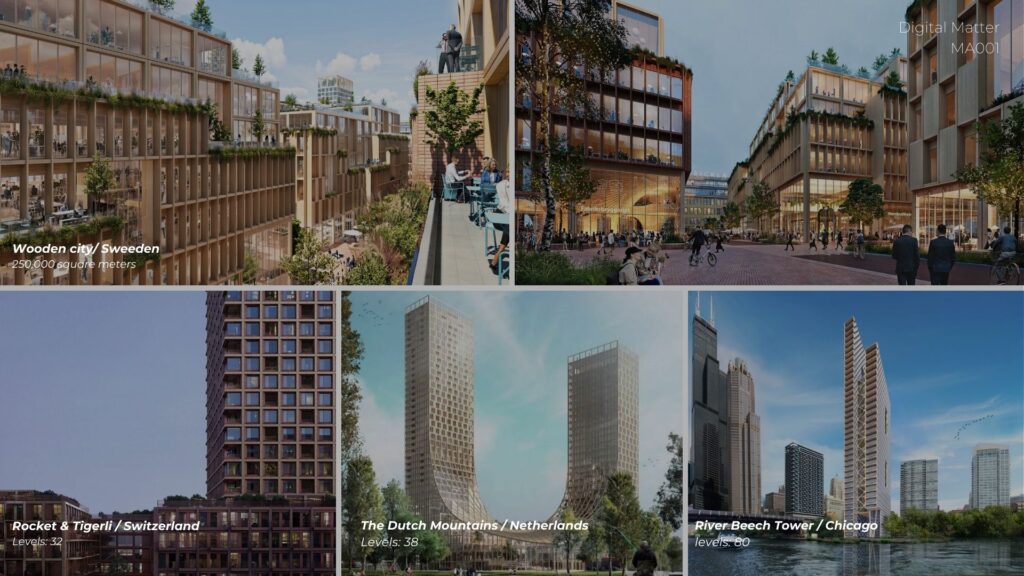
Today, 50% of the harvested wood goes to the construction industry
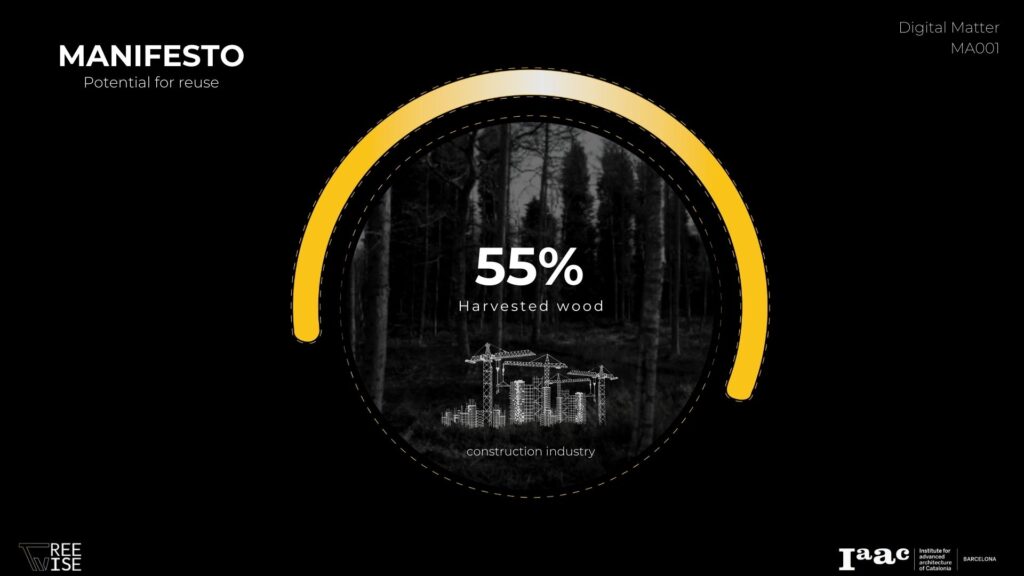
Despite of the potential of timber components to be recycled (95%),
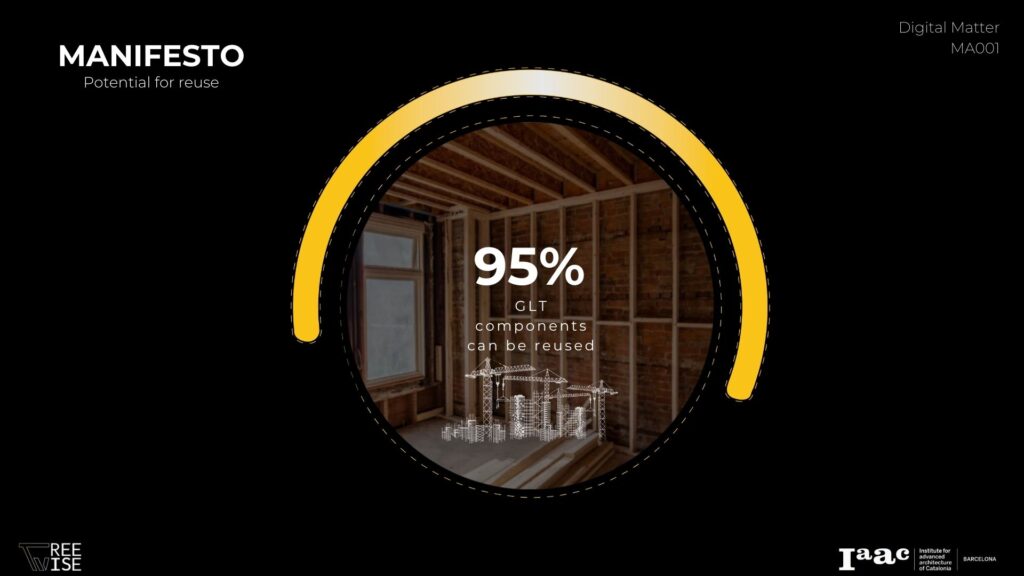
80% of the waste ends up in landfills, while only 20% is directed towards the cascading process for reuse.
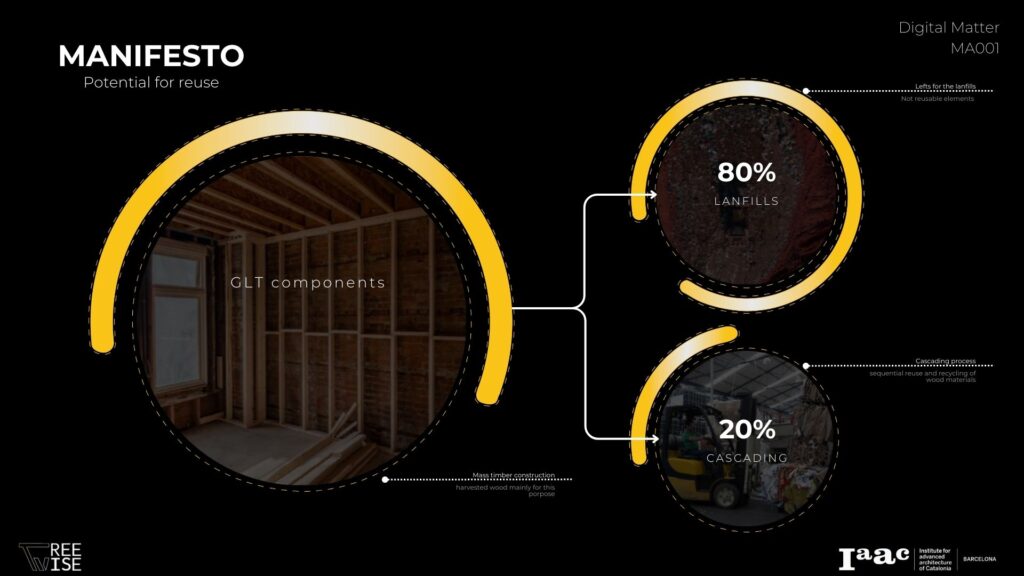
What if we prolongate the lifespan of the timber elements by disassembling and reusing this components?
By reusing GLT components we could decrease the amount of harvested wood, energy consumption and sequester carbon even for a longer period of time before returning wood into the soil.
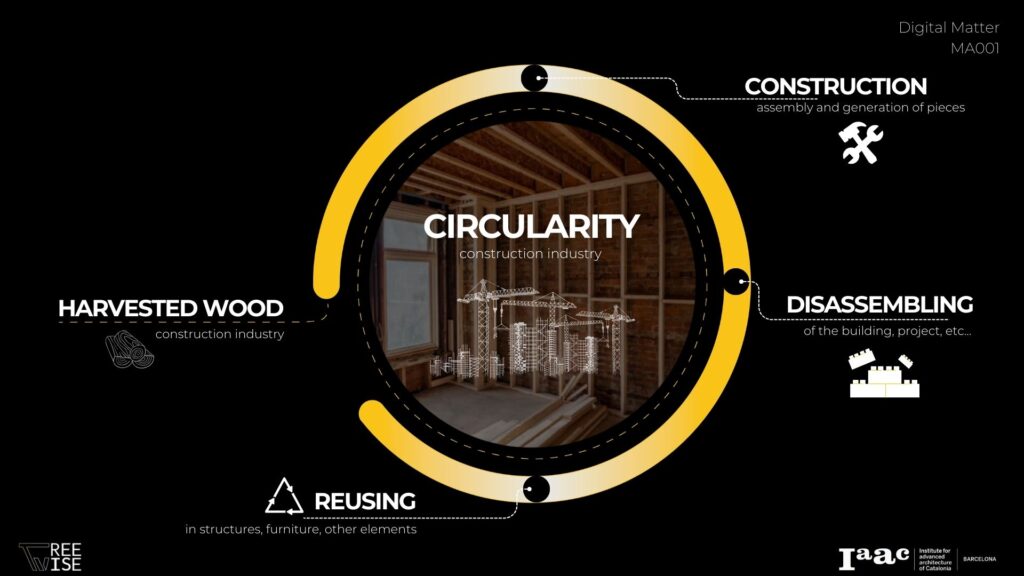
How can we be sure of the state of the timber and the performance of it for future processes?
As timber projects expand, security policies and recycling guidelines must evolve accordingly. This necessitates comprehensive monitoring, which can now be achieved through the implementation of the Internet of Things (IoT). IoT enables us to continuously track our models and implement digital twins that communicate, analyze, and aggregate data via actuators, sensors, and other devices.
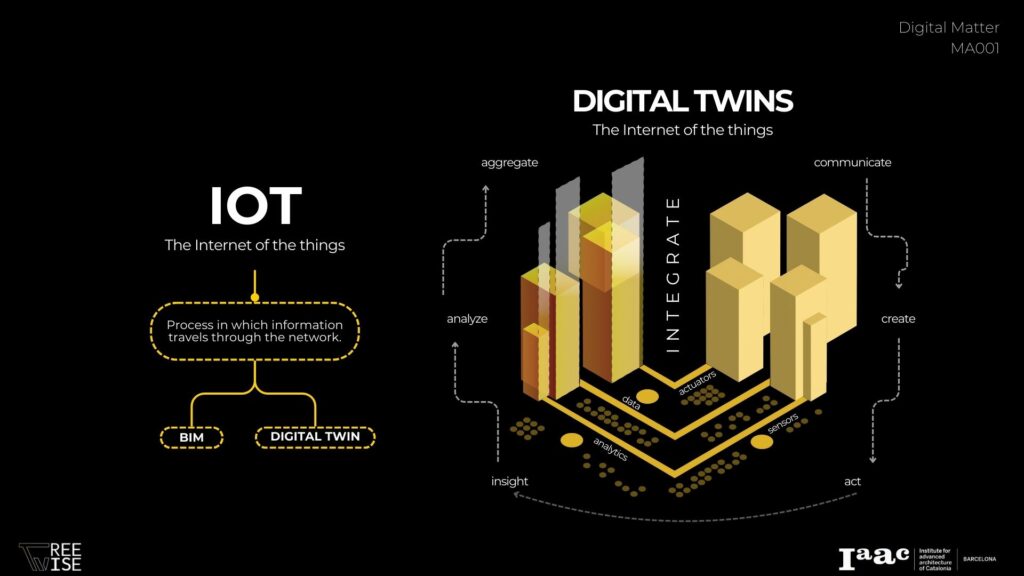
However, current monitoring technologies used in huge projects are very expensive; and in timber it is limited to humidity and heat sensing.
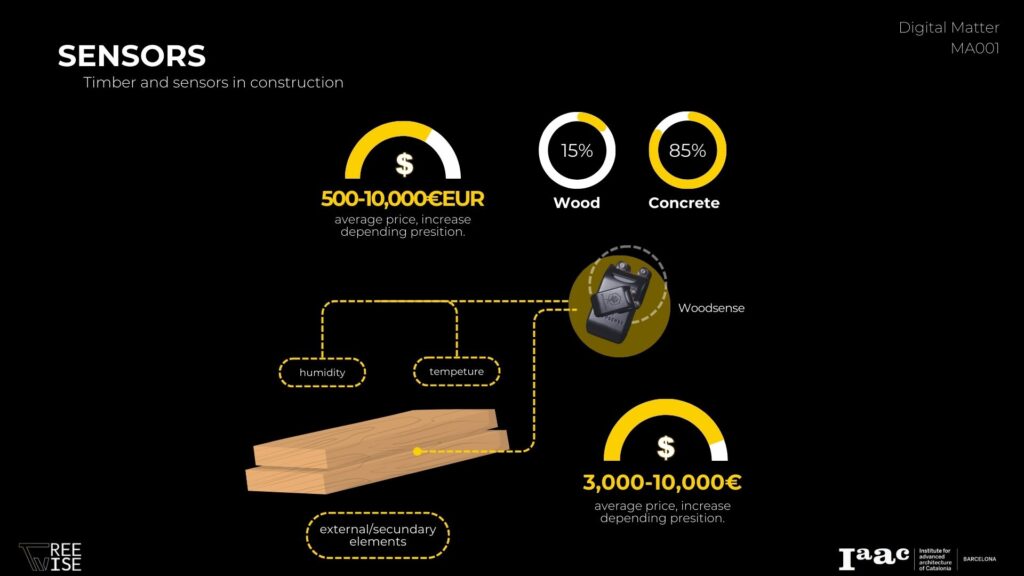
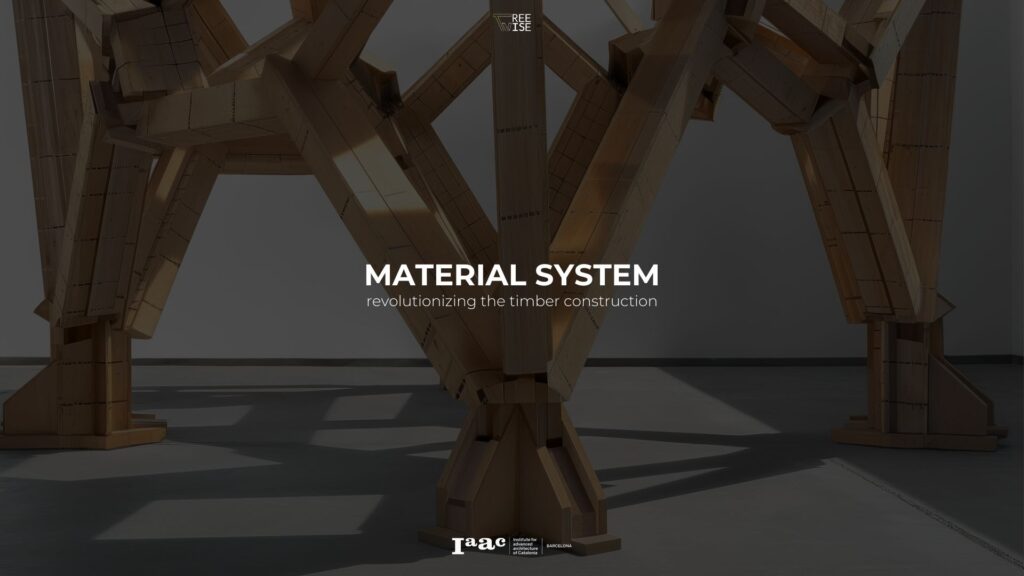
Recent studies have demonstrated graphene’s capability as a material that enables sensing due to its properties such as high conductivity, high capacitance, and biodegradability. Additionally, this material functions as an actuator, with the capability to activate other systems, depending the scenario.
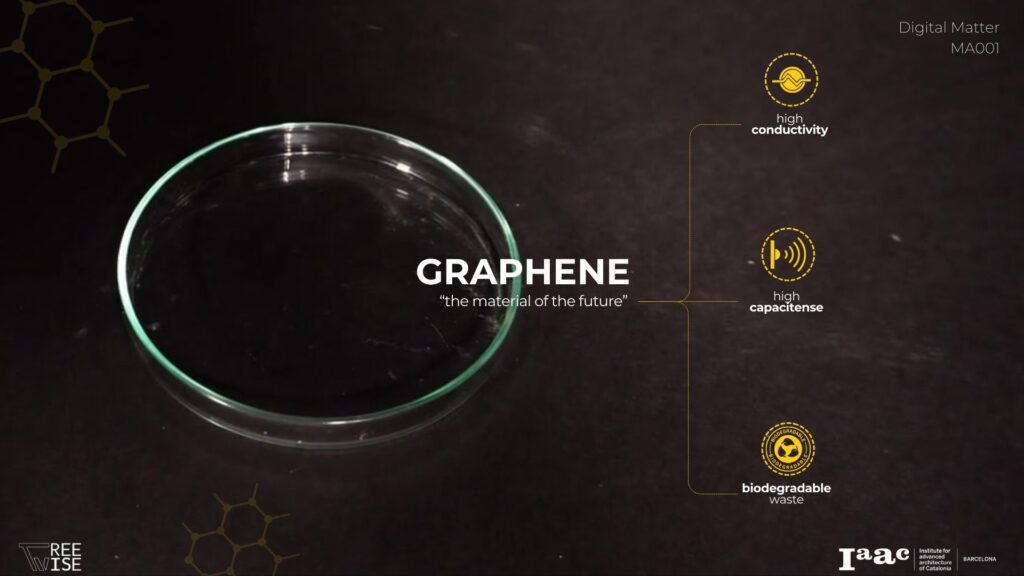
Now a days, mass timber construction system works as a linear process. A Material system combining timber and graphene,
would allow us to replace traditional sensors through graphene’s sensing capabilities.
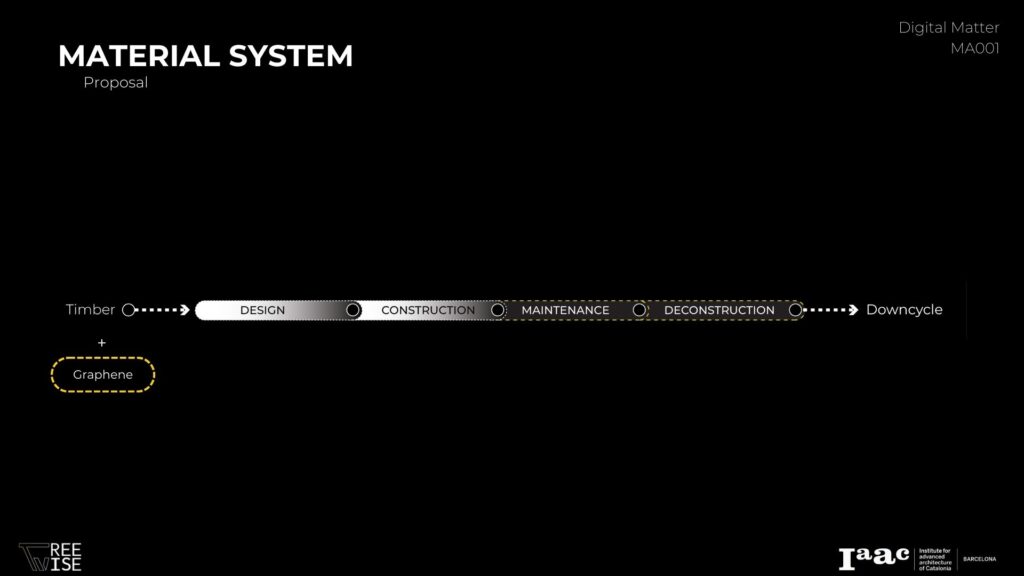
This innovative approach allow us real-time tracking of deformations inside the wood, creating a digital twin that improves maintenance efficiency in the operational phase and providing a detailed service life passport. This system increase the ability to reuse the material and reduces classification time when disassembling.
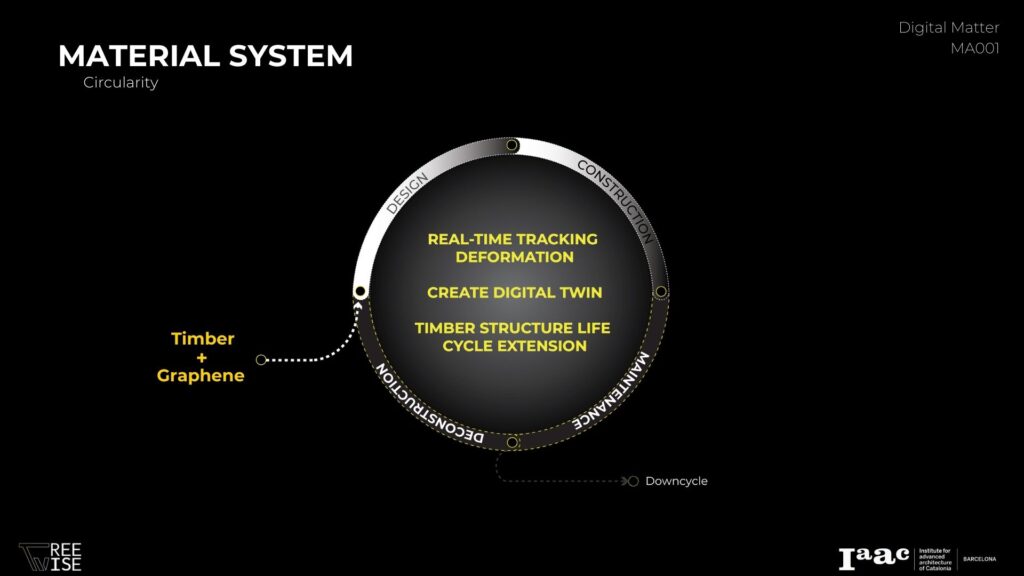
Our material system consists of laminated timber combined with graphene ink made from nanoplates in the top and bottom layers to monitored change of
conductivity when it detects any load or deformation.
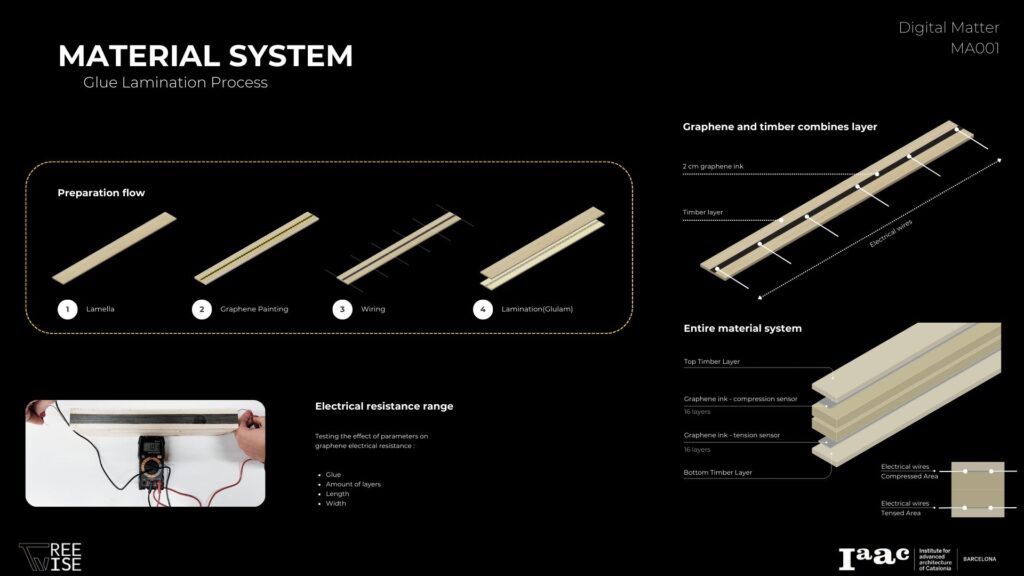
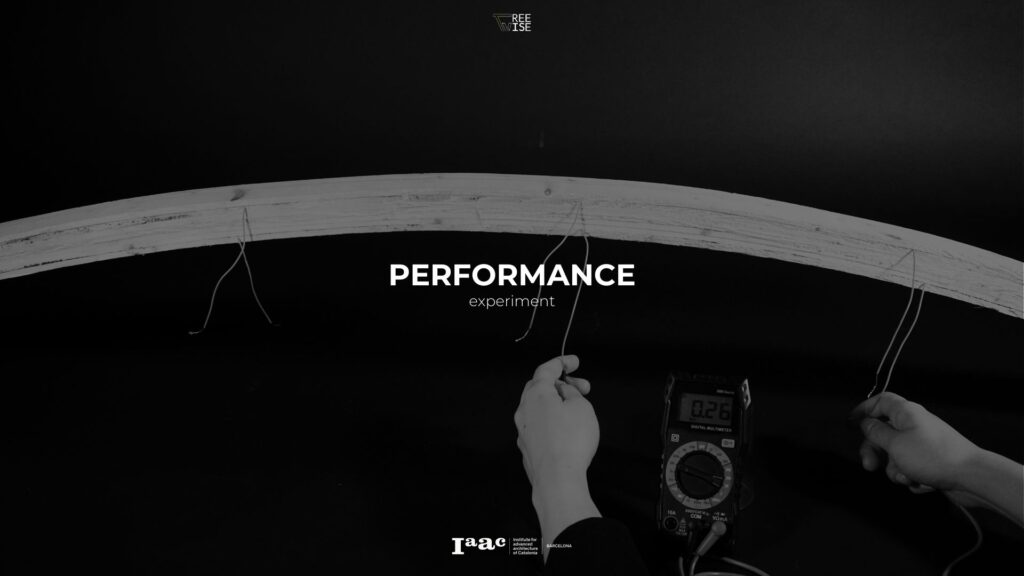
To categorize each wooden element for reuse or maintenance alerts, it is crucial to evaluate the deformations represented in the strain/stress graph.
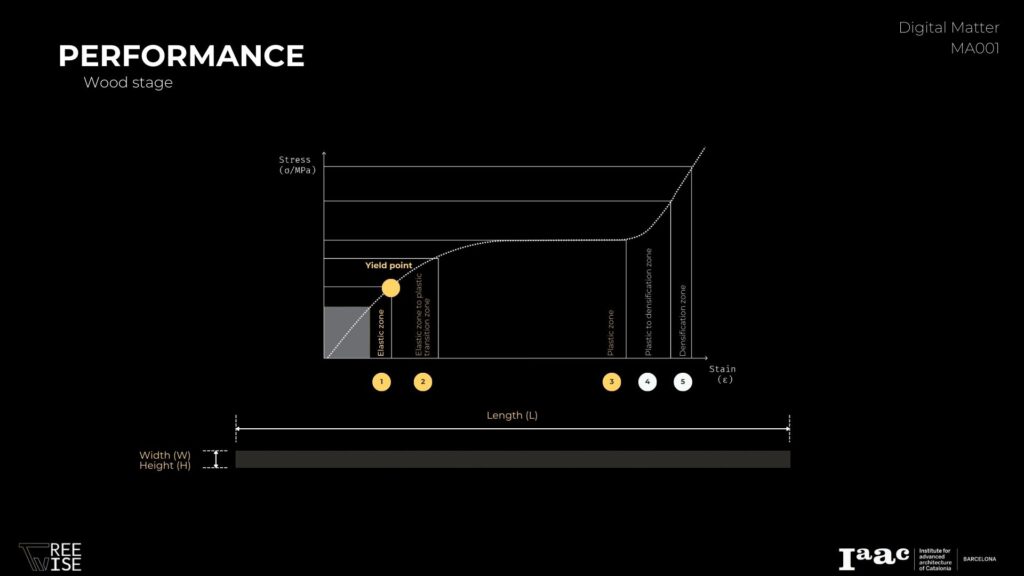
Within the elastic zone, a structural element cannot exceed the maximum deflections allowed by timber structure regulations.
Beyond this point, the timber element will need to be reassessed for a different purpose.
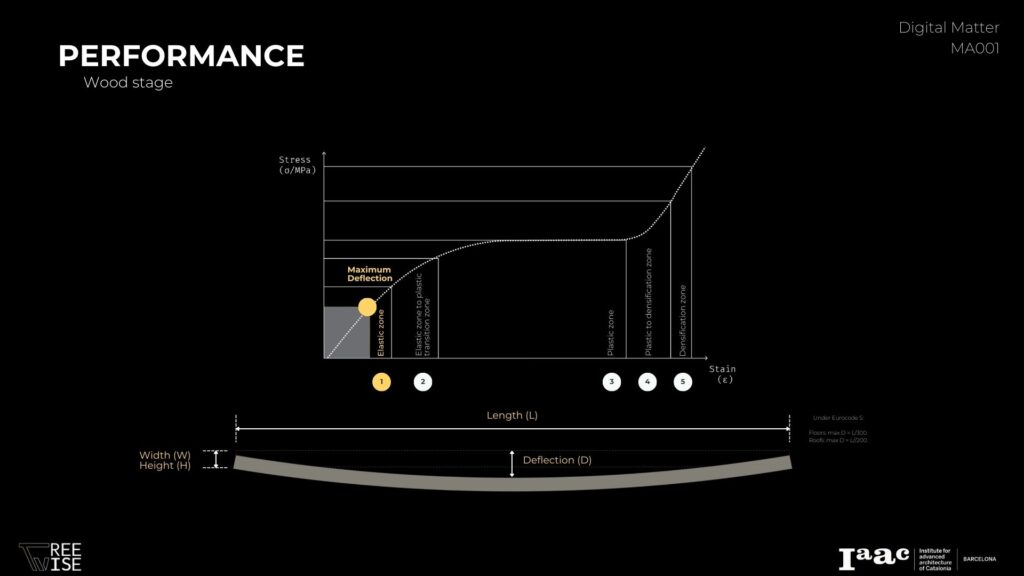
When the strain is beyond the elastic zone the element will need maintenance before being reused.
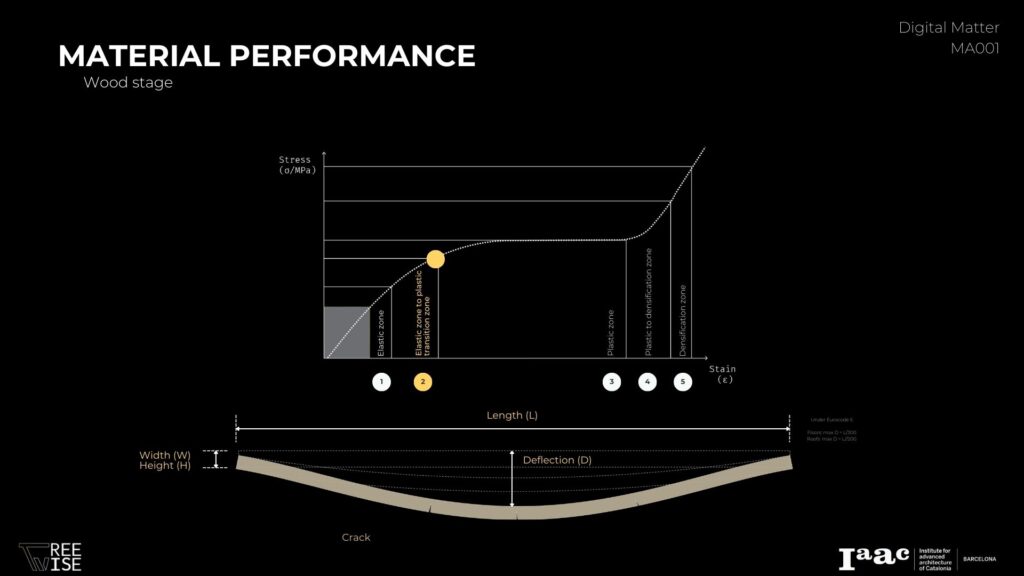
Similar to wood, graphene reacts to deformations by changing its electrical resistance. In the video, you can see its resistance increasing as the beam bends
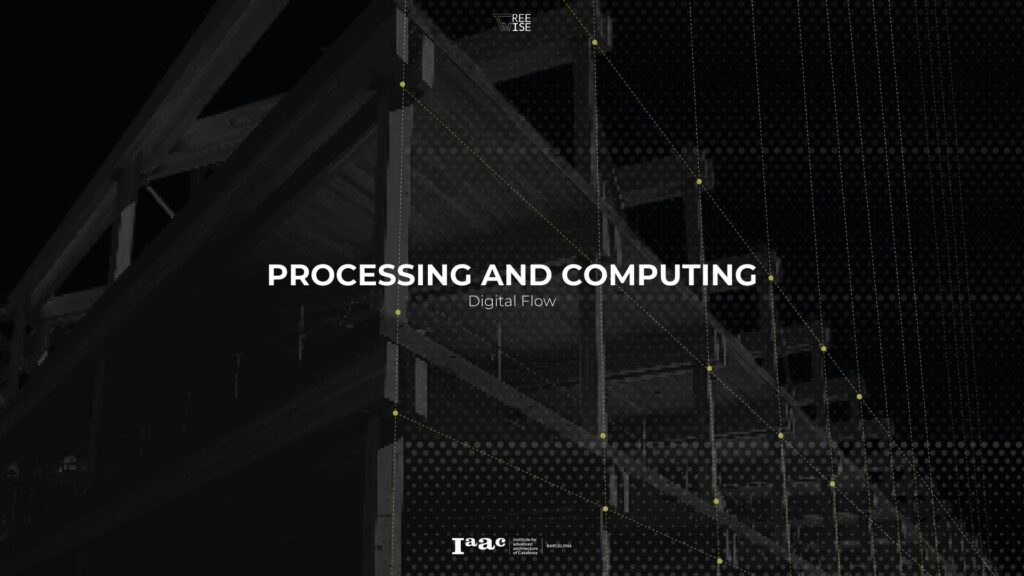
To link the state of a wooden element to the change in the electrical resistance of graphene, we trained a machine learning model.
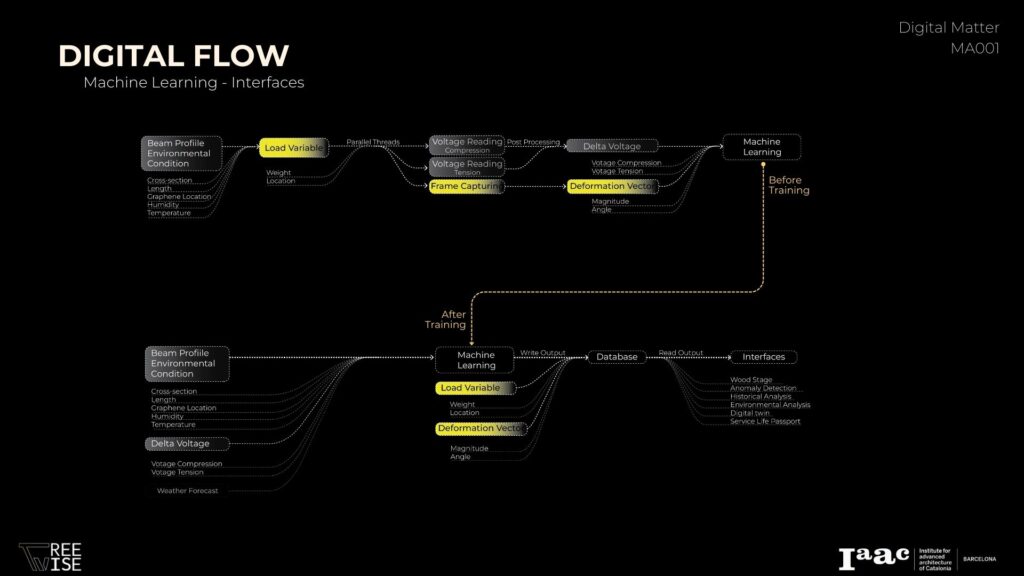
Create a database for training, we fabricated diverse glue-laminated beams with graphene in various sizes and curvatures
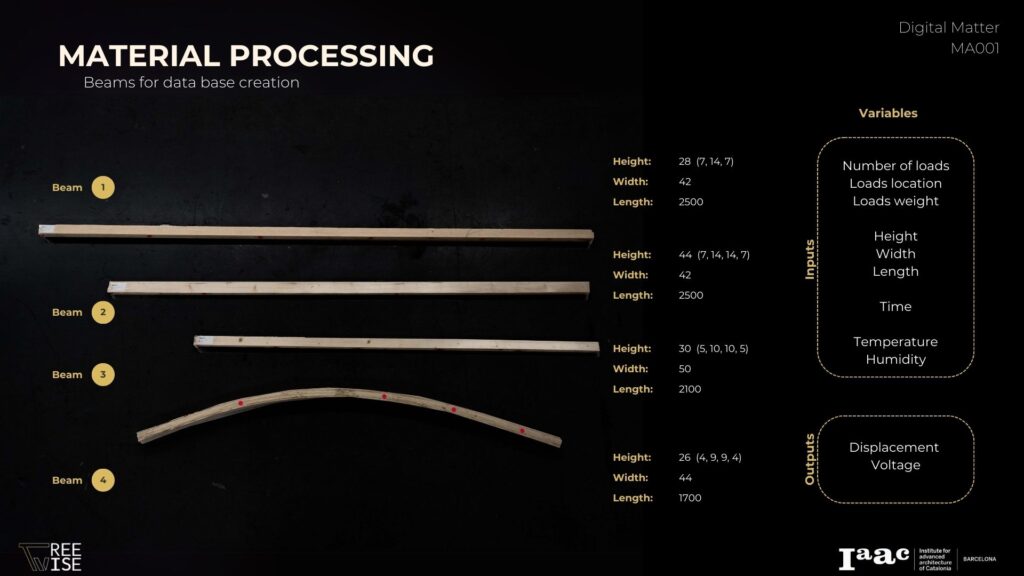
For data collection, we generated the following setup:
A beam placed on two joints, Arduino circuits for voltage readings in the tensed and compressed layers and camera capturing images of deformations.
All the data was collected simultaneously in parallel threads and was linked with timestamps
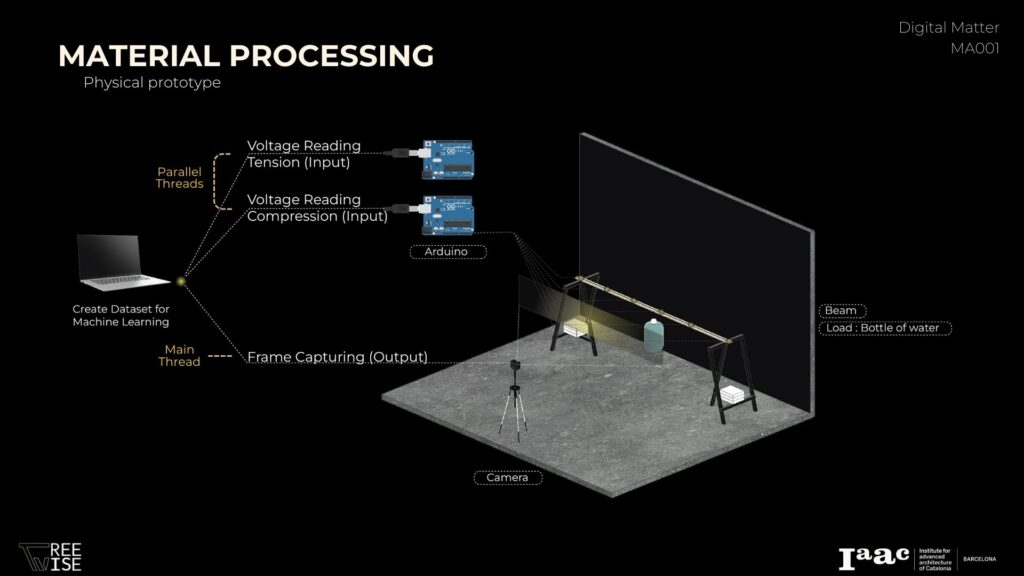
Additionally, data on the beam properties and environmental conditions were collected as well.
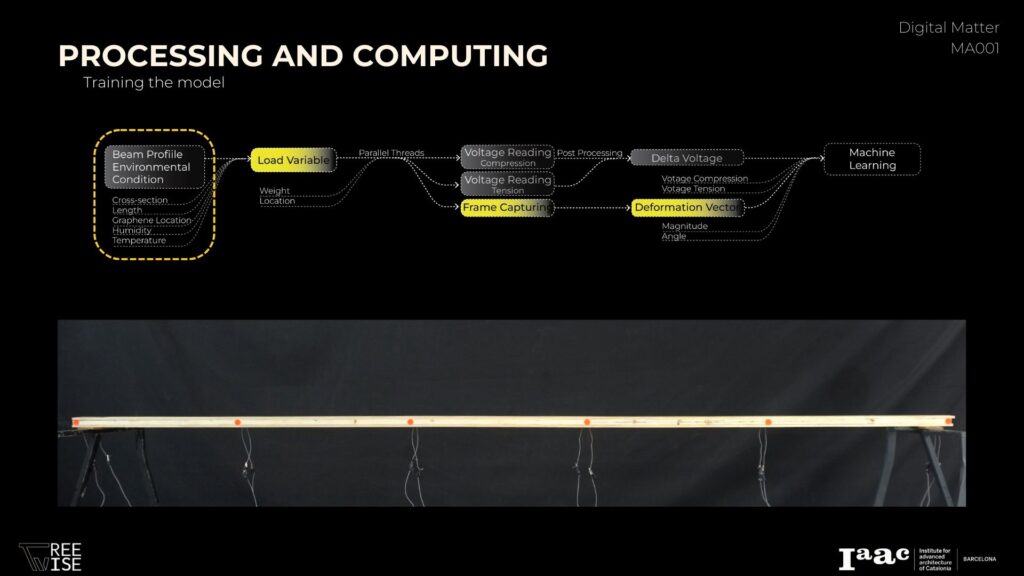
In each recording set, one or multiple loads were hung on the beam at five different locations.
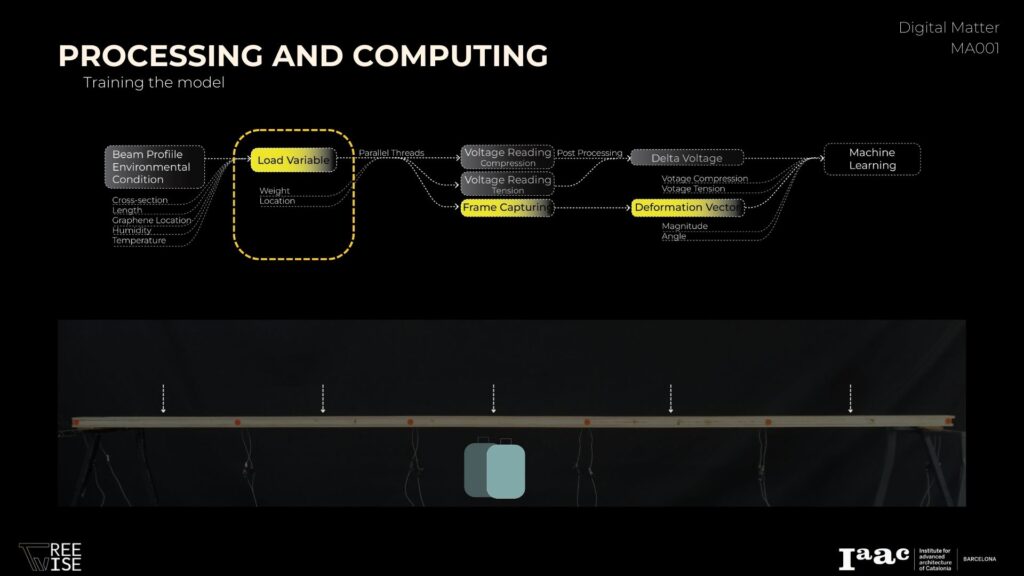
and the changes in voltages from the neutral position were gathered
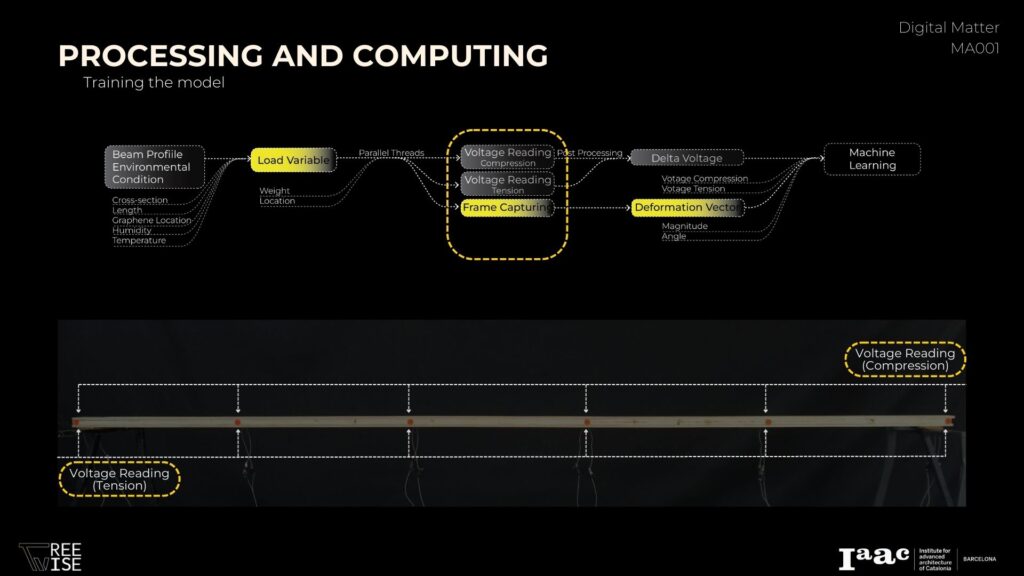
For deformation recordings, the images were post-processed with OpenCV code to extract the magnitude and angle of the displacement vectors.
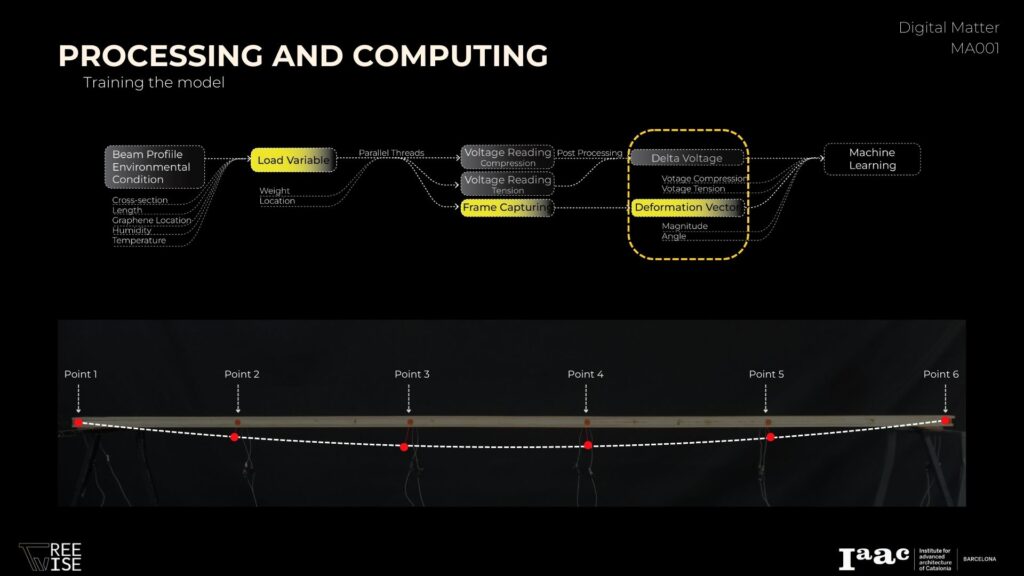
A clean database of around 50,000 samples was used in a TensorFlow Sequential model. Input data included voltage changes, properties, and environmental conditions. Output data included deformation vectors, load positions, and weights. The model had three hidden layers with batch normalization and a linear output layer.
We used a custom loss function, an exponential learning rate decay, and early stopping to prevent overfitting. As our main inputs were voltage readings, the input data was very noisy, making it harder to achieve accuracy. After the model was trained, it is used to predict the deformation vectors, load weight and location by reading the change in voltage in the graphene. This predicted data is constantly pushed to an interface we will now present.
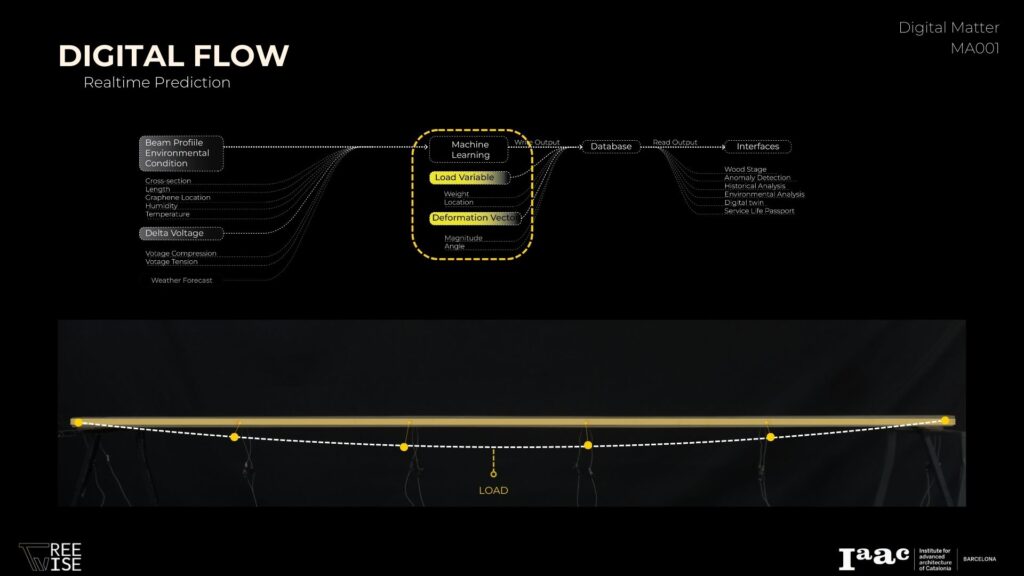
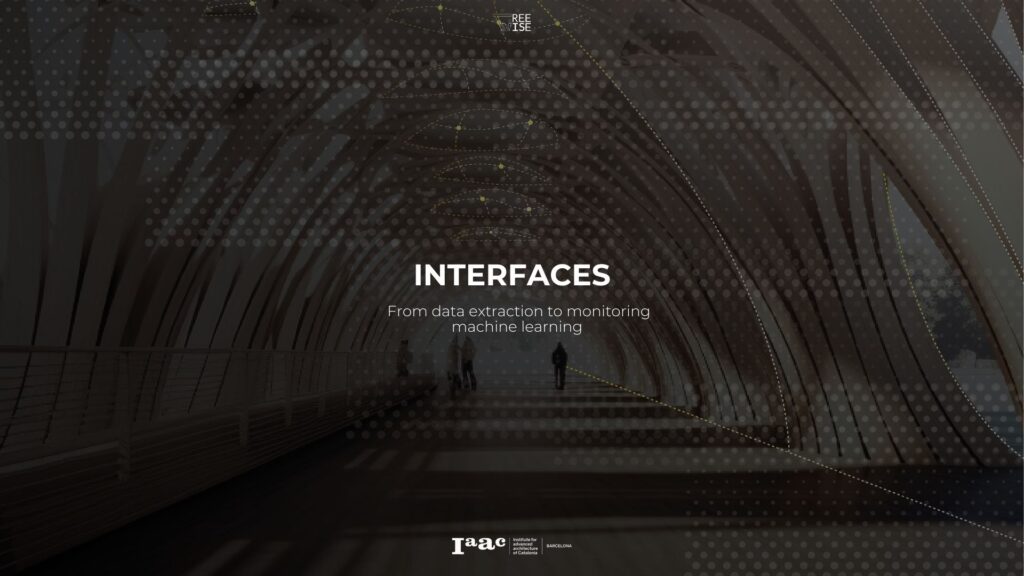
The interface is designed to support professionals in building facility management. It provides a data dashboard and creates a digital twin based on
the precise data obtained from the structural performance. This dashboard allows for accurate monitoring of structural performance,
showing material displacement and identifying maintenance needs.
The data dashboard features an anomaly detection section and a graph monitoring of the strain gauges on timber in real-time,
ensuring fast and efficient checks for immediate action.
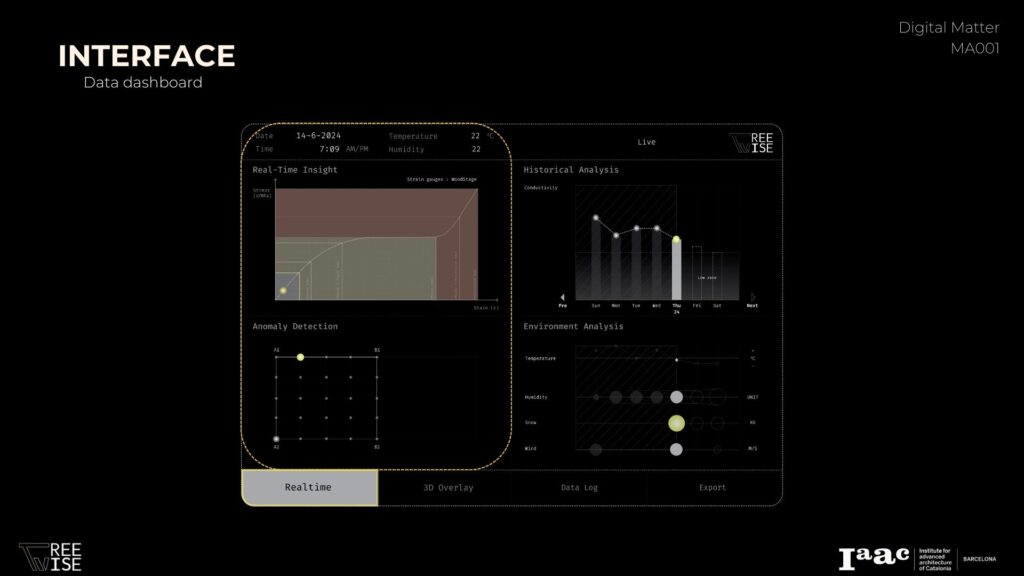
in the same dashboard the interface also offers weekly data records along with environmental factors such as weather and humidity
to optimize maintenance performance, prevent timber deformation, and ensure structural security.
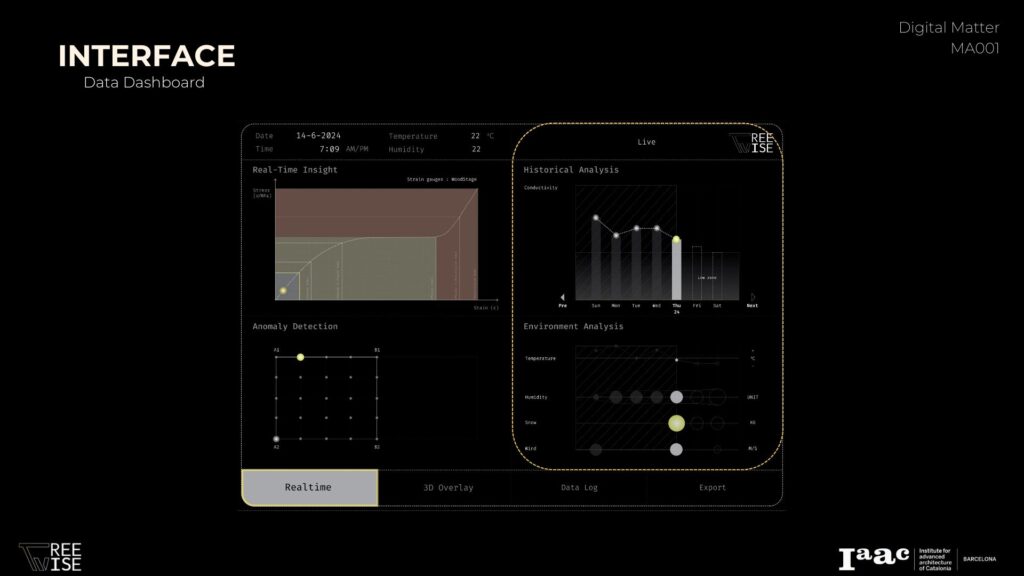
Second dashboard allows users to monitor each graphene-enhanced element to observe displacements and deformations caused by loads.
This data is managed through a digital twin for precise and efficient analysis
Last dashboard shows A Service Life Passport of the material The passport is included for the additional value of using digital twin to extend the timber life span.
Each element’s digital twin and service life passport are categorized by the state of the material in the strain gauge.
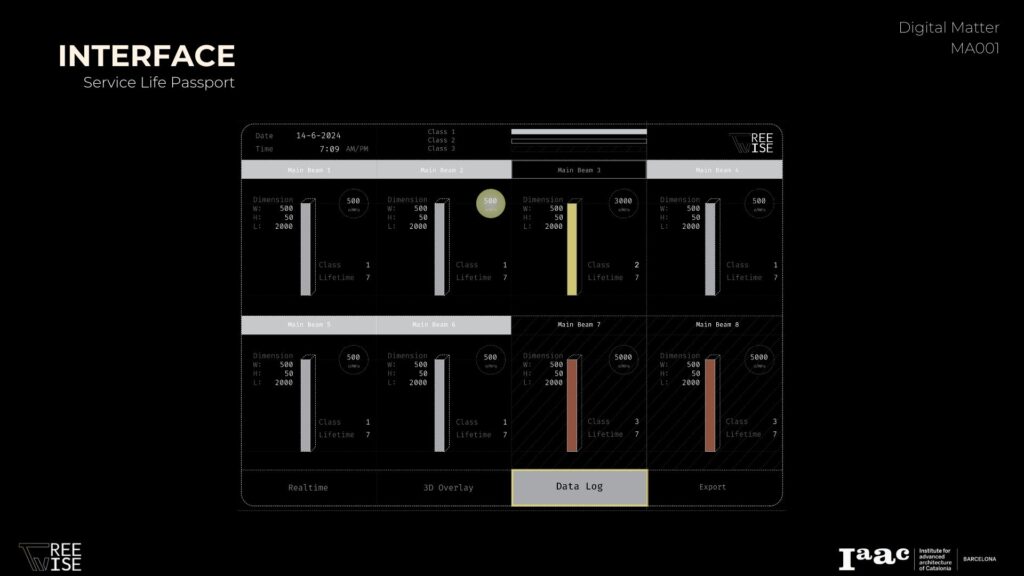
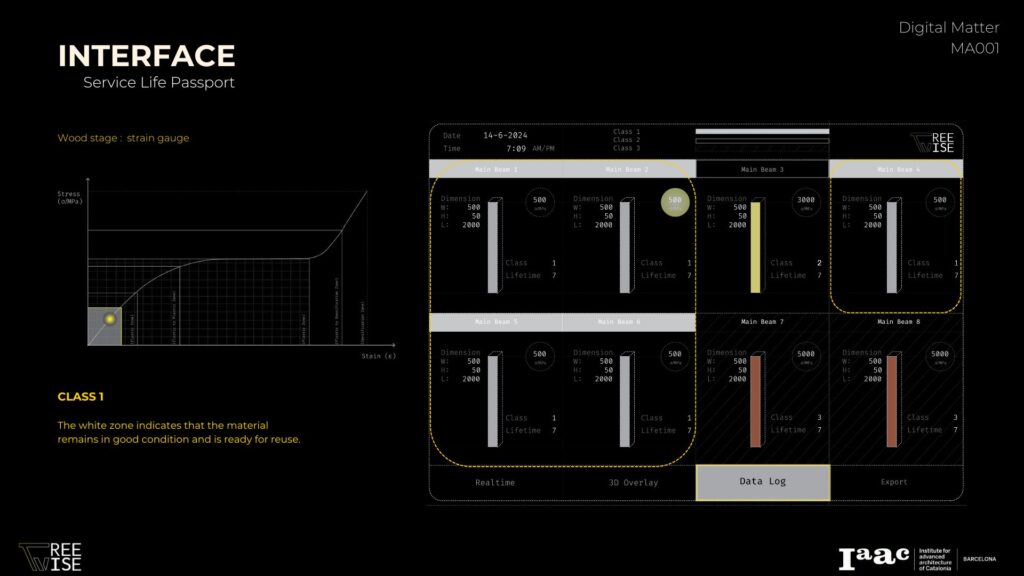
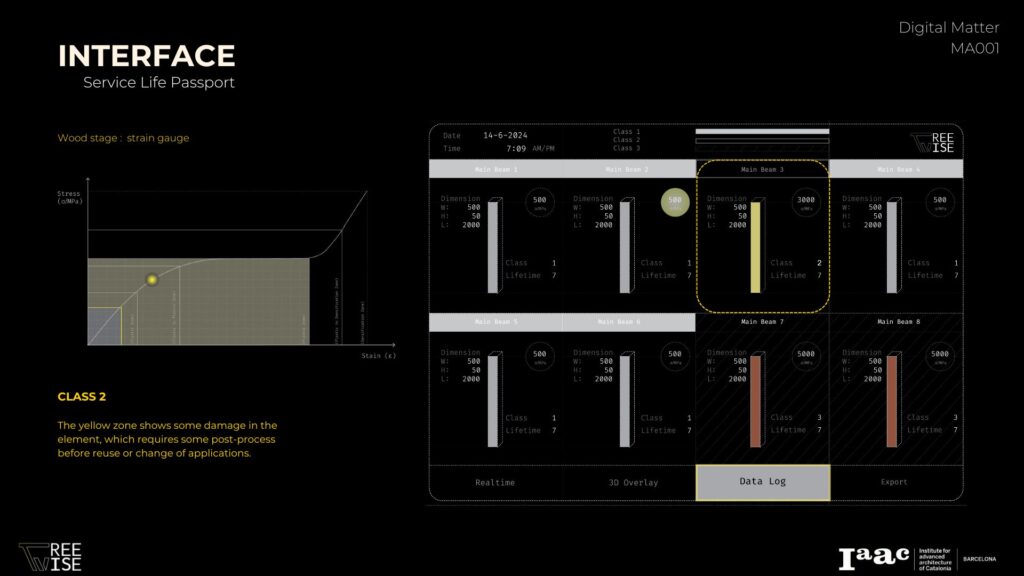
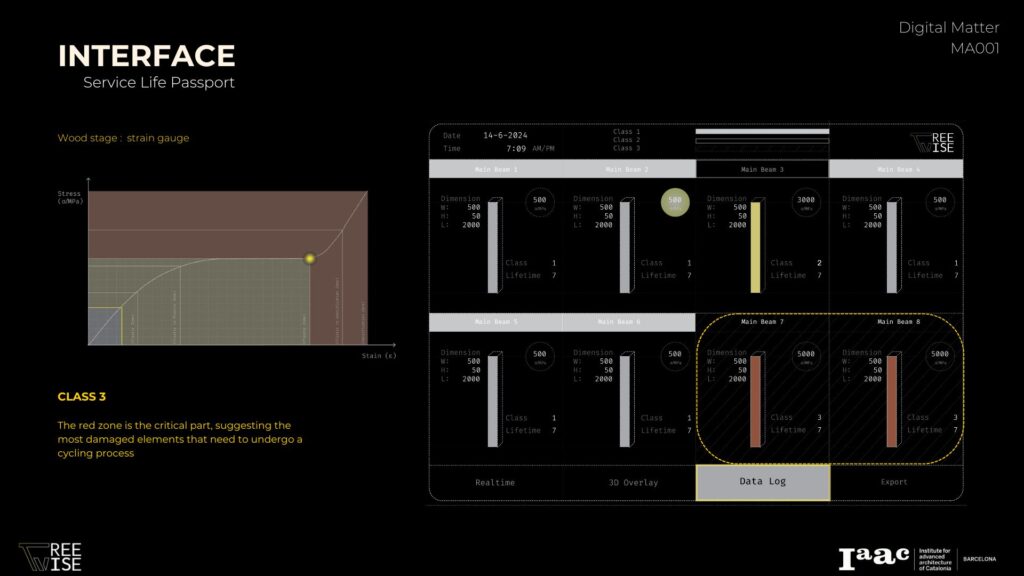
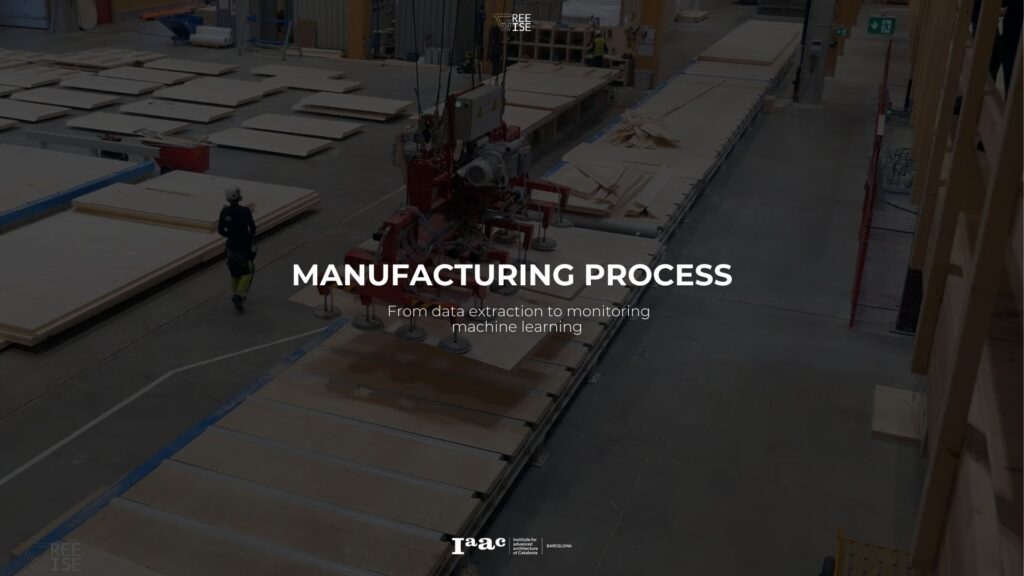
The manufacturing process consists in mainly three processes, the laser assisted deposition into the wood, the glue lamination as it is now at the common industry and the application of the data loggers for the manipulation of different pieces at the same time.
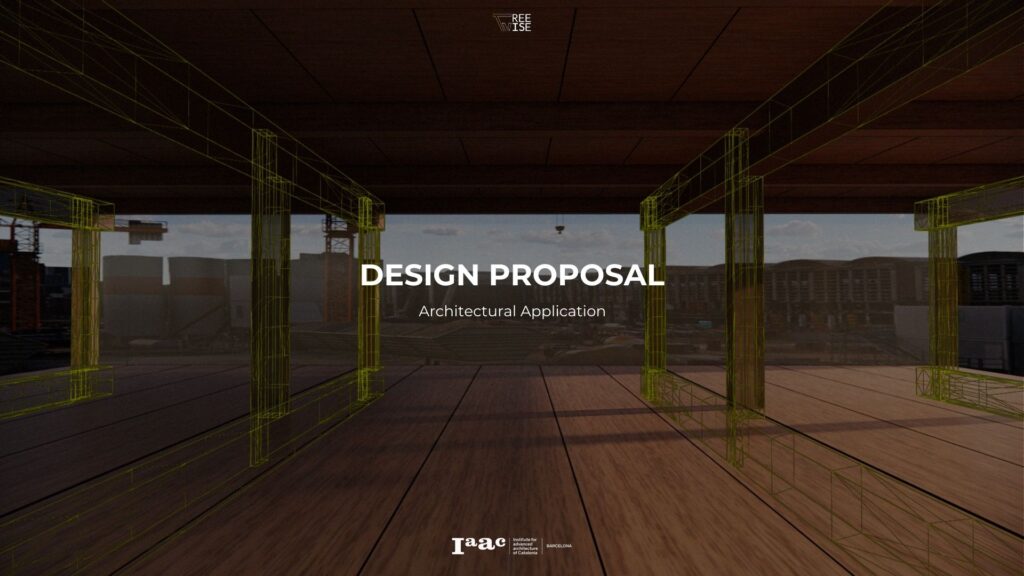
Our design proposal takes 2 scenarios: the first one for structure reuse and the second one, for complex geometry monitoring.
First scenario, implementation of graphene sensing material in timber elements for industrial design and reuse.
That would allow in the disassembly process to have a classification depending on the service life passport of each element after being monitored to use it as the same structural element or even going into a downcycle process and define for it a new use.
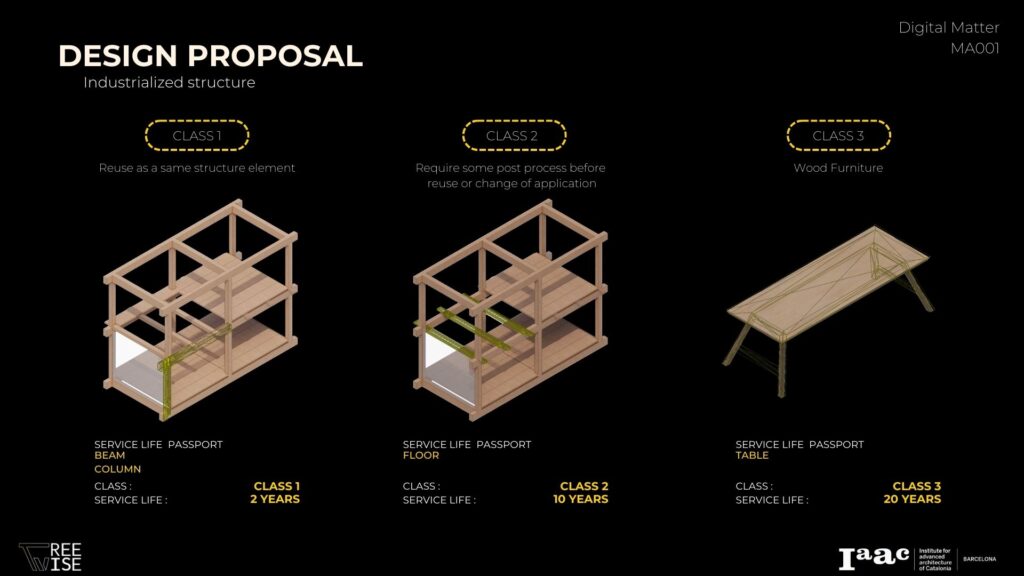
Second scenario, optimizing the graphene placement in complex geometries for supplying real time alerts and need for maintenance
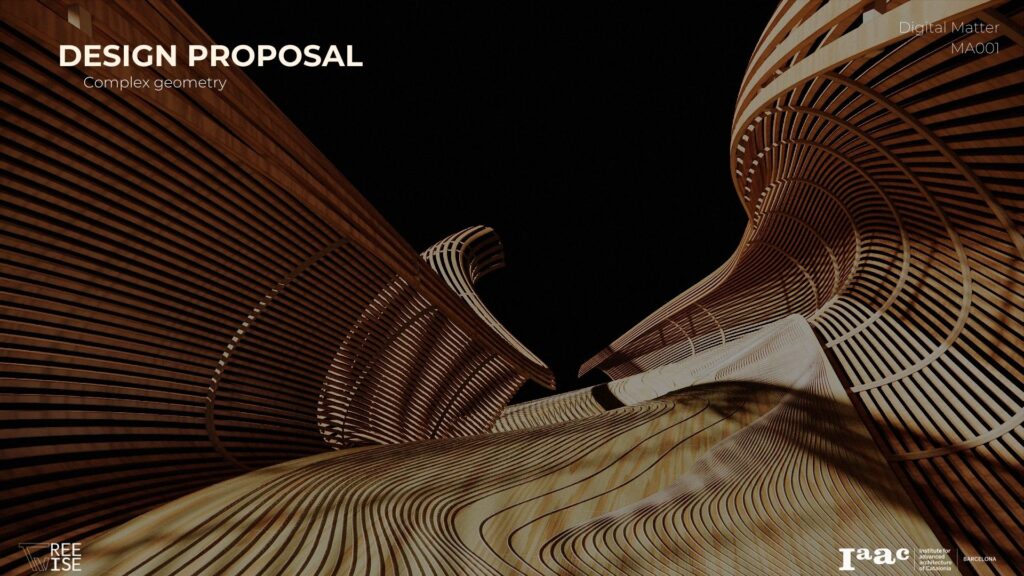
As in this scenario each element is unique, the graphene deposition can be optimized through the combination of Karamba Analysis.
The graphene then will be place in the weakest parts of the structure.
In both scenarios, the structure synchronizes with a digital twin through wireless data transmission,
The system allows for remote monitoring and provides real-time alerts through data logger.
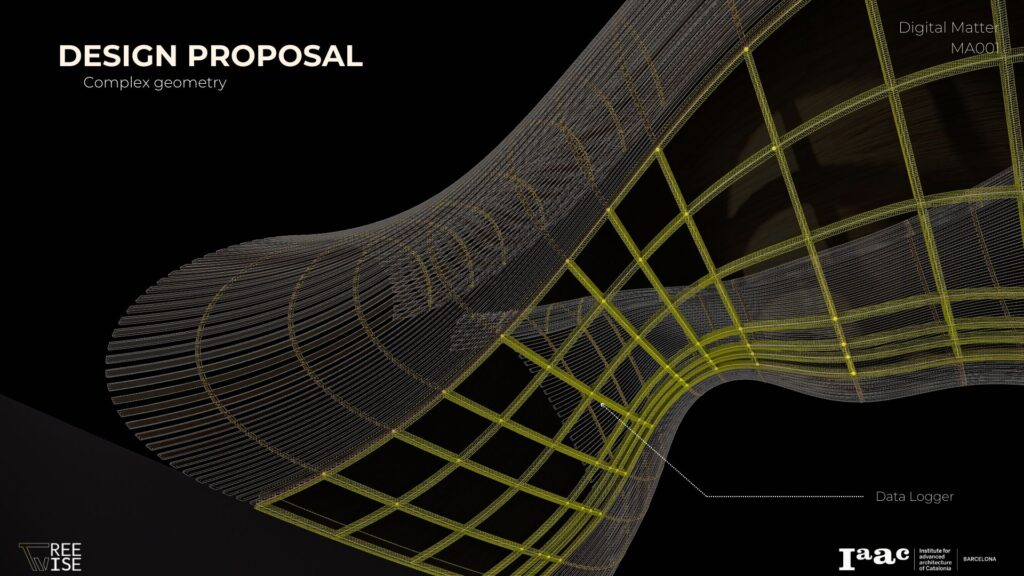
Interface and Bridge mock-up
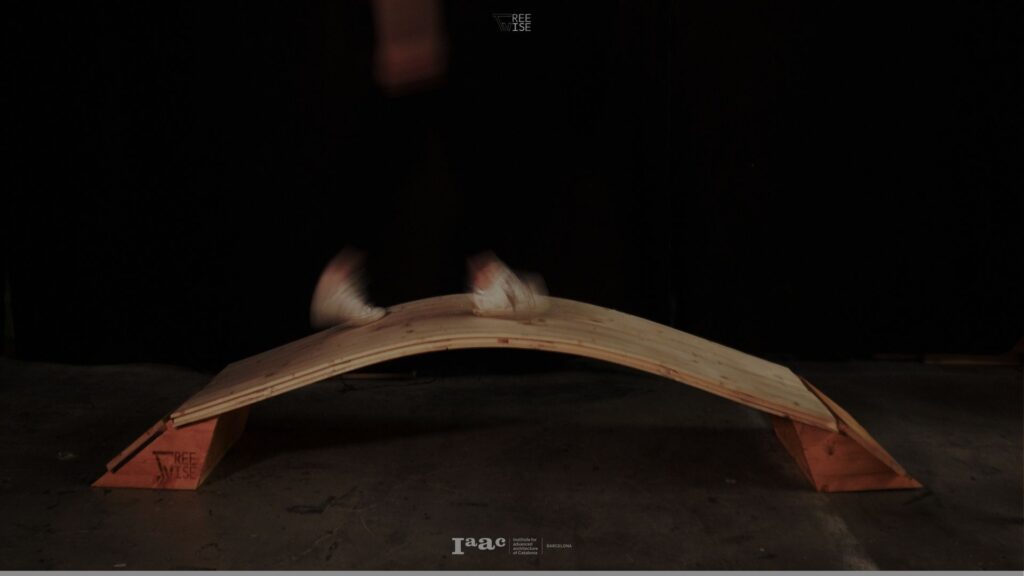
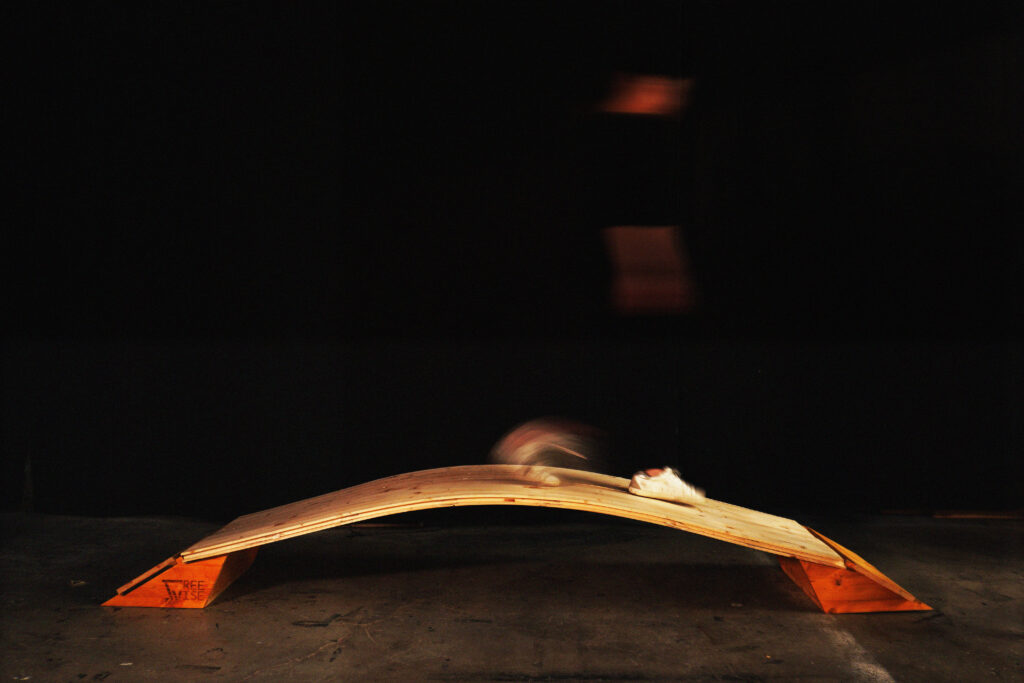