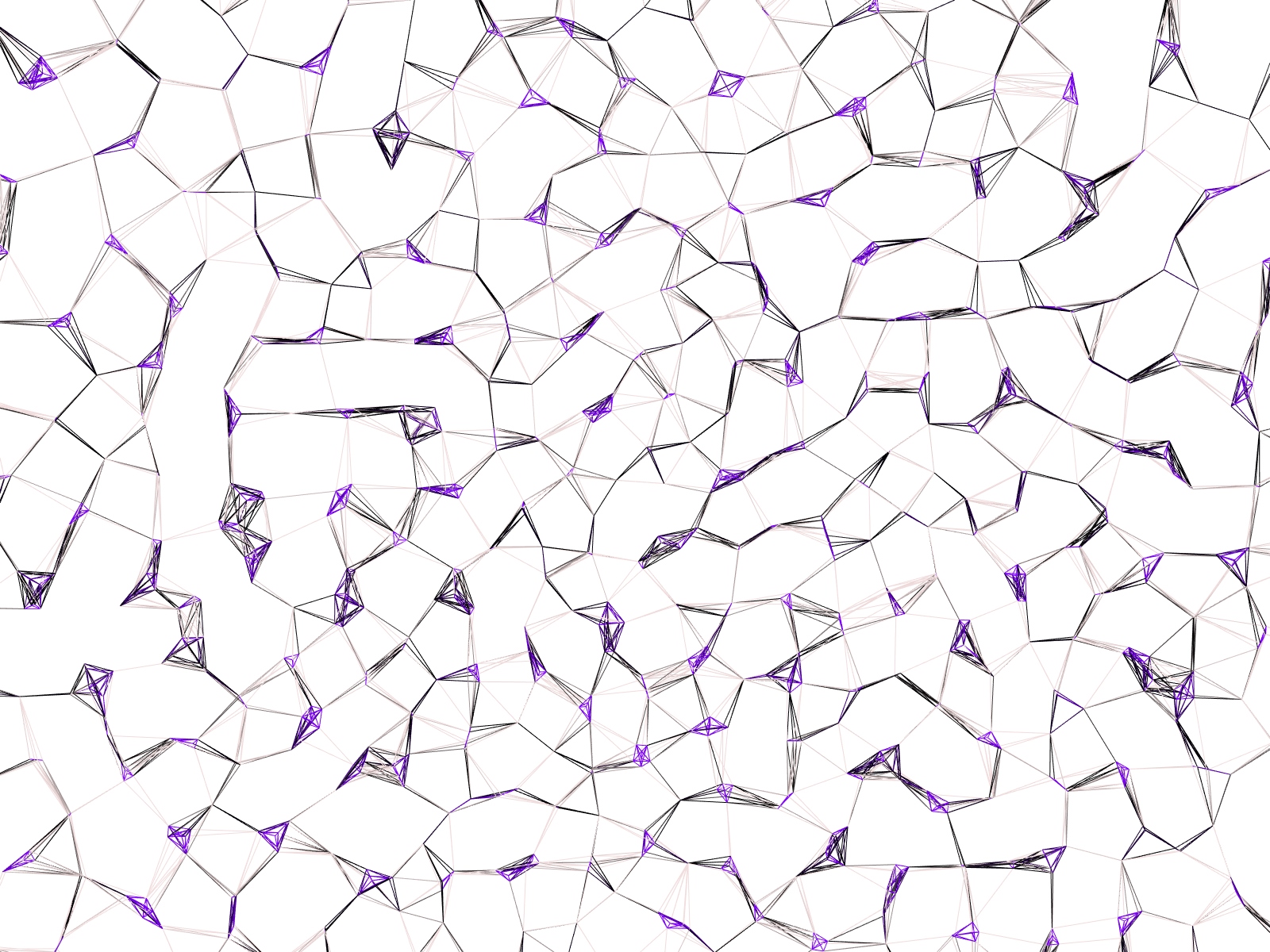
Photo credit: Mateusz Zwierzycki
Description
This course will be an opportunity to experiment with various machine learning tools and algorithms available in Owl. It will start with a lecture explaining the current and the past problems of AI in design. The presentation will introduce the notions of supervised, unsupervised and reinforcement learning. Using Owl, the participants will then learn the Grasshopper-based workflow for neural networks (supervised learning). Introduction will end with an overview of Owl components used for data pre- and post-processing.
After the introduction, the focus will be put on the group projects and the ways to utilise ML to aid the designs either in sensing/responding, controlling and predicting. Neural networks will be used for both regression and classification problems. The students will learn how to understand and control the learning process through hyperparameters.
Over the course of the semester this knowledge shall be extended to application of ANN in the students’ projects.
Learning Objectives
During this course the students will be challenged to incorporate artificial neural network models either for sensing/response, control or prediction.
To be able to work efficiently with the NN models, the students will have to:
- understand the meaning of the training parameters
- understand the consequences of NN architecture changes
- learn how to prepare and post-process the data for ML