The Environmental Asset Resilience (EAR) Tool is a response to the pressing global challenge of environmental instability, particularly in urban areas. These areas face substantial costs associated with environmental problems and climate change. However, decision-making in addressing these issues is complicated by conflicting priorities, limited resources, and a lack of expertise. The EAR Project aims to develop methodologies to foster and strengthen environmental assets in urban areas, ultimately achieving ecological balance.
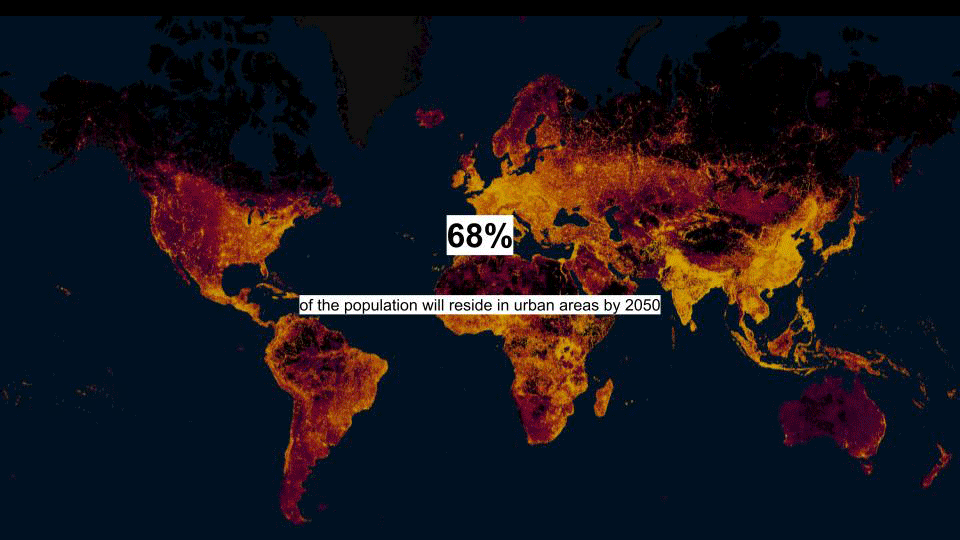
In the past 30 years we have witnessed the alarming loss of nearly a quarter of our forests, concerning this trend, experts suggest that at least 50% of our landscapes need to be protected. However, with an estimated 70% of people predicted to reside in urban areas in the future, the pressure on landscapes further intensifies.
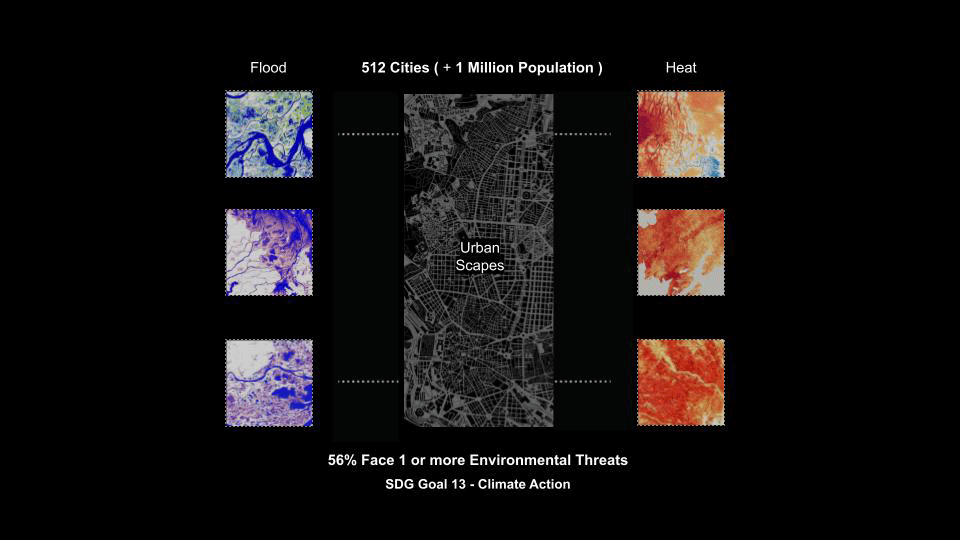
Out of 512 cities where the majority of the population resides, over half of them confront one or more climate challenges, predominantly characterized by excessive heat and flooding. The complexity of rapid urbanization, coupled with heightened vulnerability to climate disasters, often prevents cities from effectively identifying potential uncertainties and allocating resources accordingly contributing to the formation of dysfunctional landscapes.
FRAMEWORK POTENTIALS
Several frameworks and analysis tools have been examined, including the U.N.D.R.R. (United Nations Office for Disaster Risk Reduction), which focuses on disaster reduction, and ESRI Green Infrastructure, which focuses on planning and categorizing landscapes for protection and adaptation purposes. These frameworks predominantly encompass perspectives from various domains, which are policy, ecology, disaster reduction, and planning, each with its own distinct expertise.
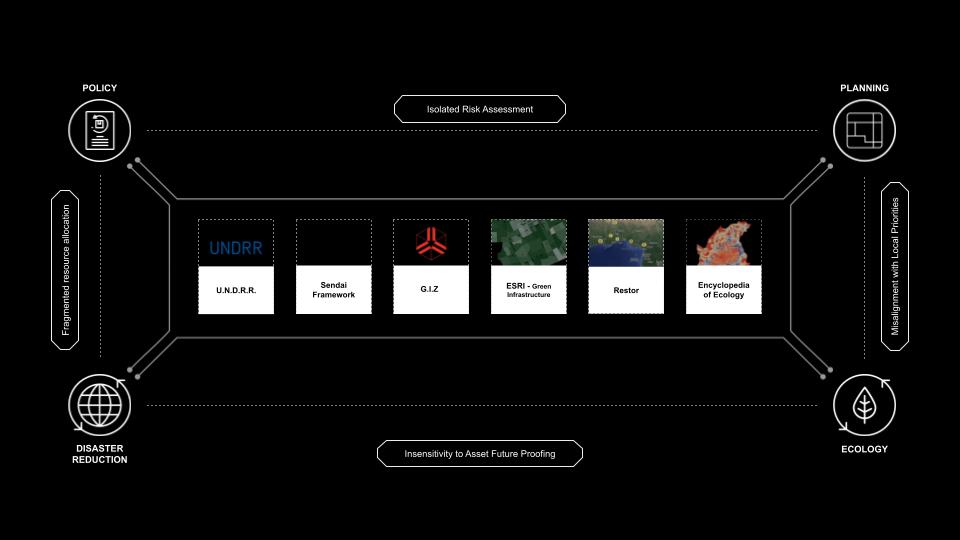
Although these perspectives can occasionally complement each other, they frequently encounter gaps and discrepancies. While formulating planning policies, Risk assessments are often conducted independently. In the realm of disaster reduction, resource allocation through policies tends to be fragmented, lacking the capability to precisely identify areas of urgency. Furthermore, disaster reduction and ecology approaches prioritize future-proofing assets without adequately considering the assessment of the assets themselves, working in isolation from risk assessments.
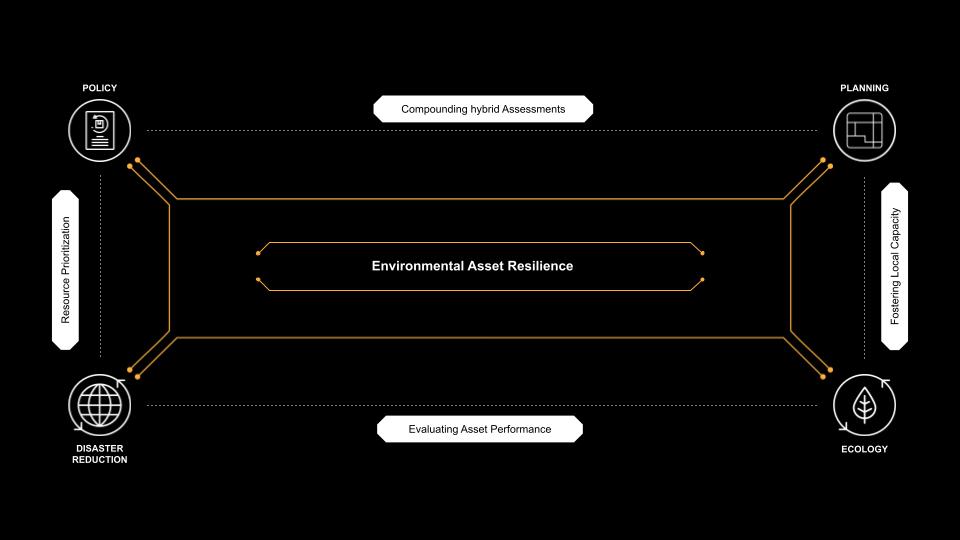
The new responsive framework aims to embrace these challenges as opportunities, recognizing the necessity of combining hybrid assessments and evaluating asset performances. By doing so, it prioritizes the allocation of resources and nurtures local capacity in urban areas.
FRAMEWORK METHODOLOGIES
The methodology comprises a learning model that can be continuously updated to adapt to evolving challenges and uncertainties. This learning model encompasses an iterative process of identifying assets, assessing the hazards posed to those assets, evaluating the assets themselves, and utilizing this information to identify and capitalize on opportunities.
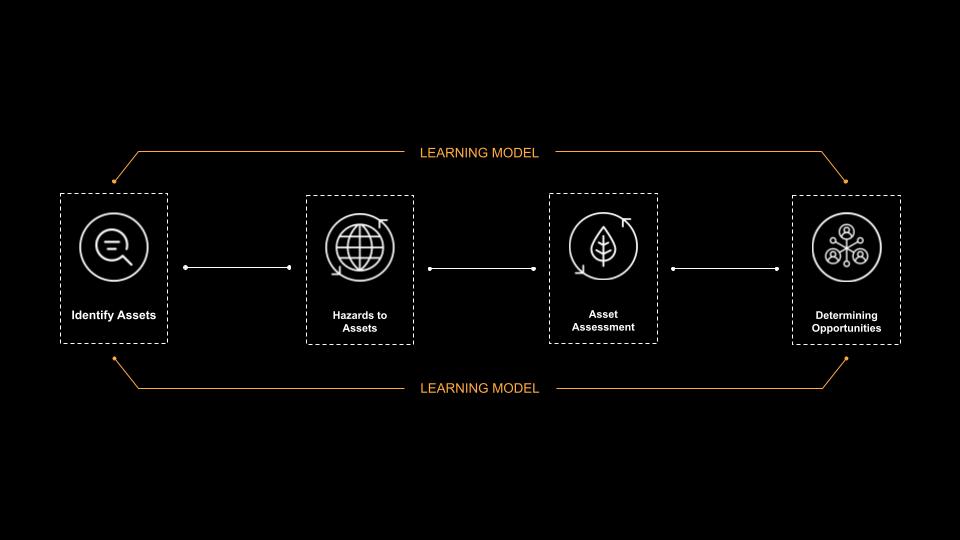
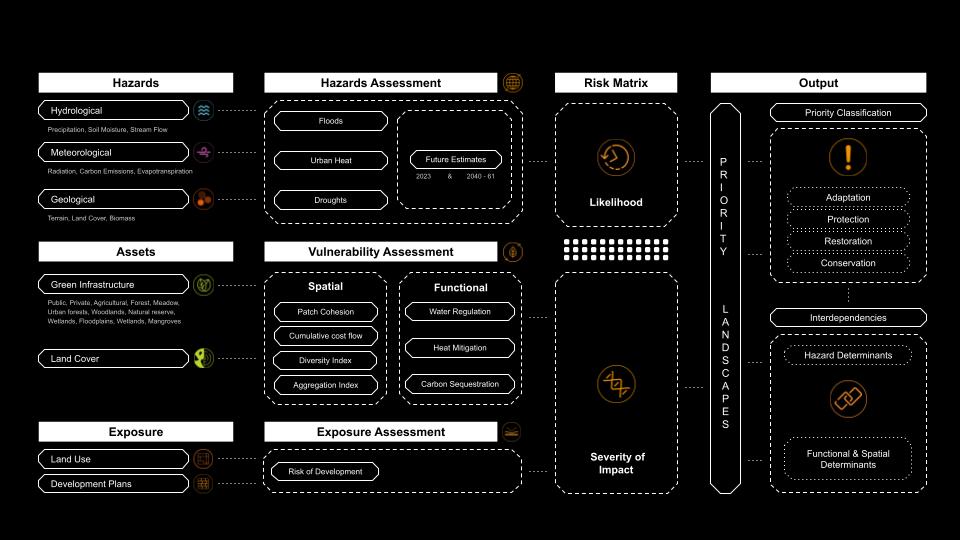
The Methodology comprises of conducting hazard assessments and vulnerability assessments, further forming a risk matrix to identify priority landscapes in the city, classifying them. and in the final step understanding the interdependencies of hazards to assets using machine learning.
ASSESSMENTS
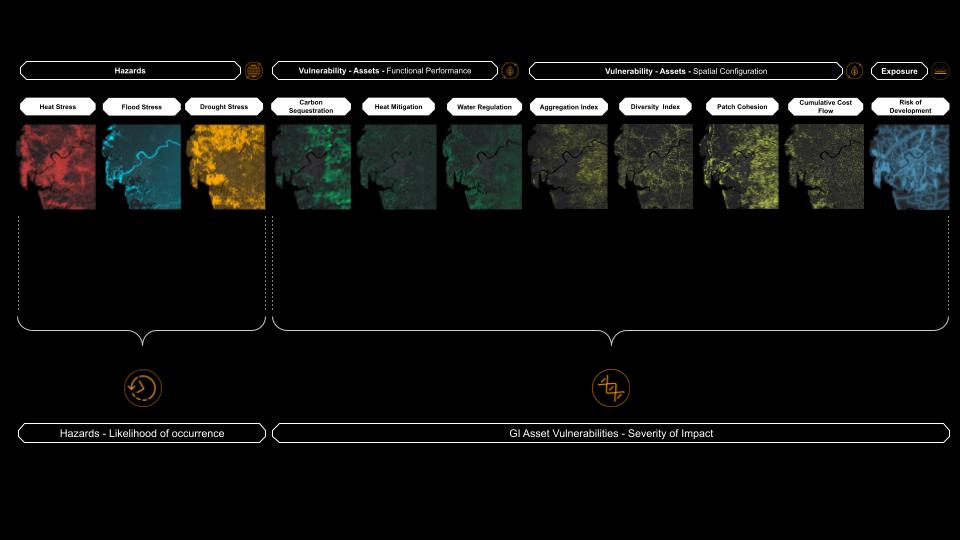
combining the hazards and their determinants, we formulate the Likelihood of Occurrence Index. Simultaneously, by integrating spatial and functional assessments with exposures, we obtain the Severity of Impact Index.
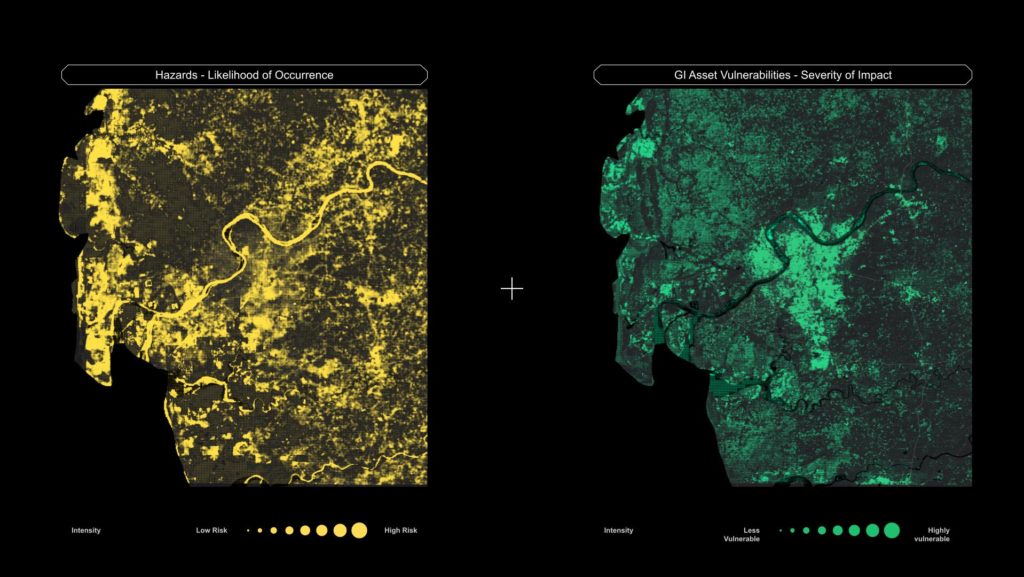
By combining these, we can gain insight into the areas that are most significantly impacted by the combined influence of both factors.
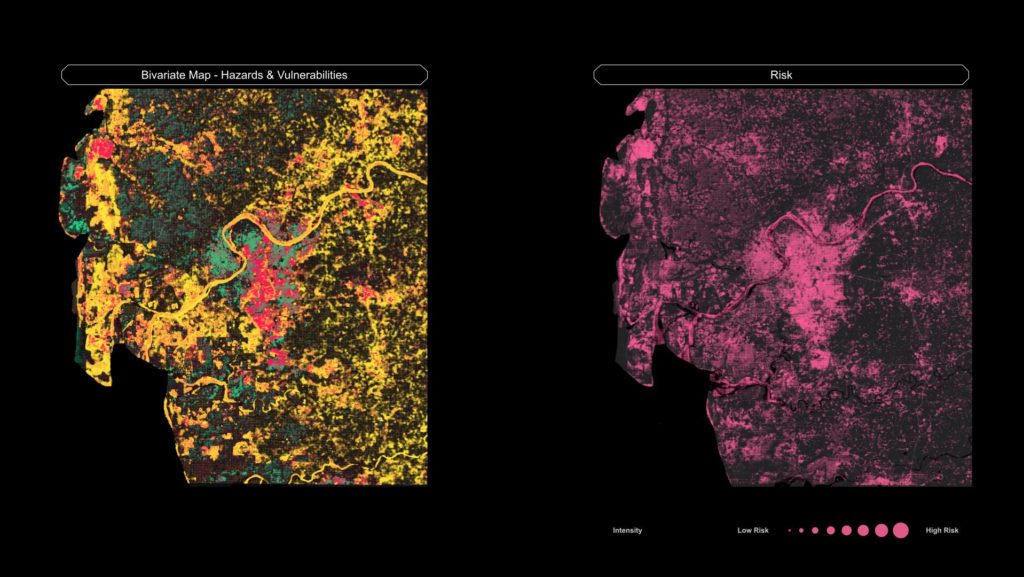
And thus we create a comprehensive risk matrix. This matrix identifies the highest-risk zones with a greater potential for adverse impacts.
PRIORITY LANDSCAPES
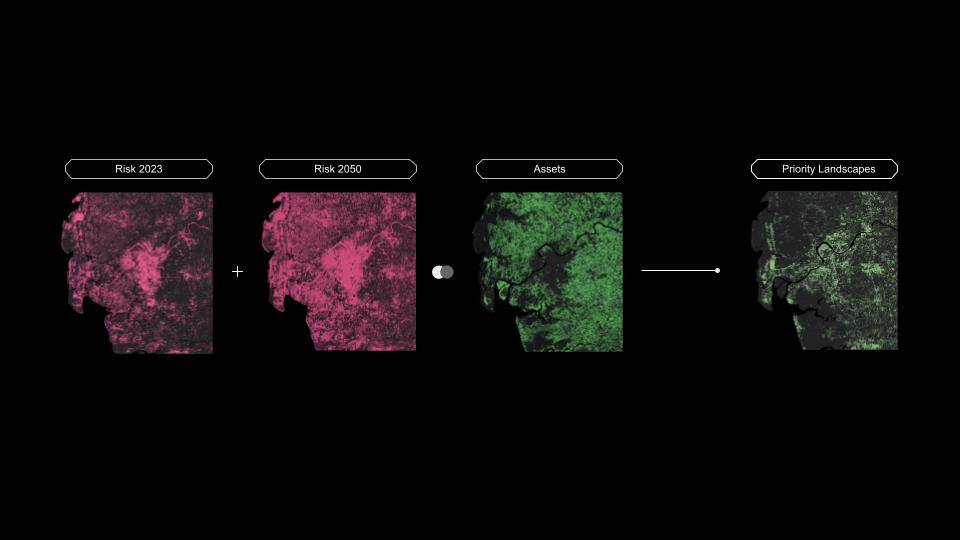
By overlaying identified assets with mapped risk areas and considering the projected aggravation of risk from 2023 to 2041-60, we prioritize landscapes based on the severity of future challenges. To clarify the responsible use of landscapes and address different environmental objective there is a further need to categorize the landscapes
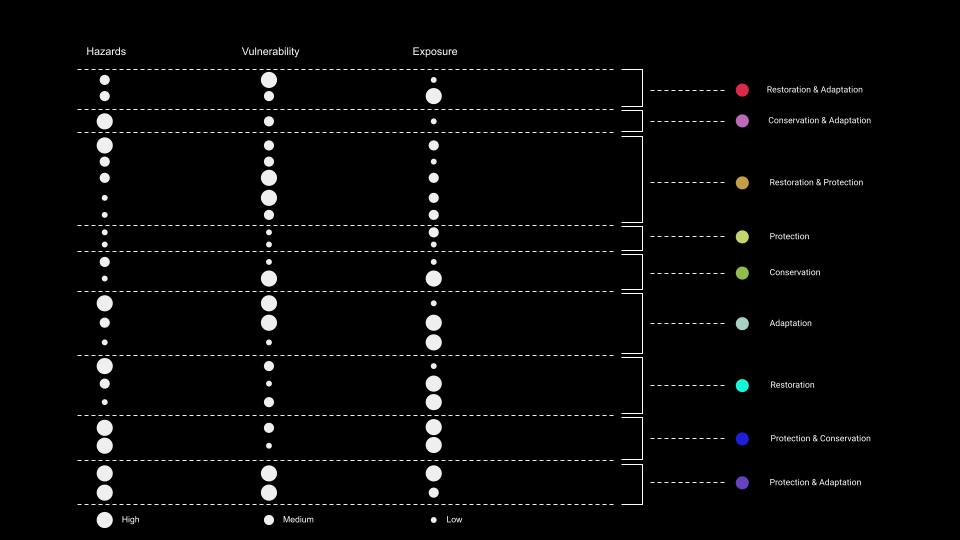
This classification is based on evaluating the varying intensities (high, medium, and low) of different assessments, including hazards, vulnerability, and exposures. By considering the permutations and combinations of these assessments, we gain a comprehensive understanding of the landscapes that require restoration, adaptation, conservation, or protection.
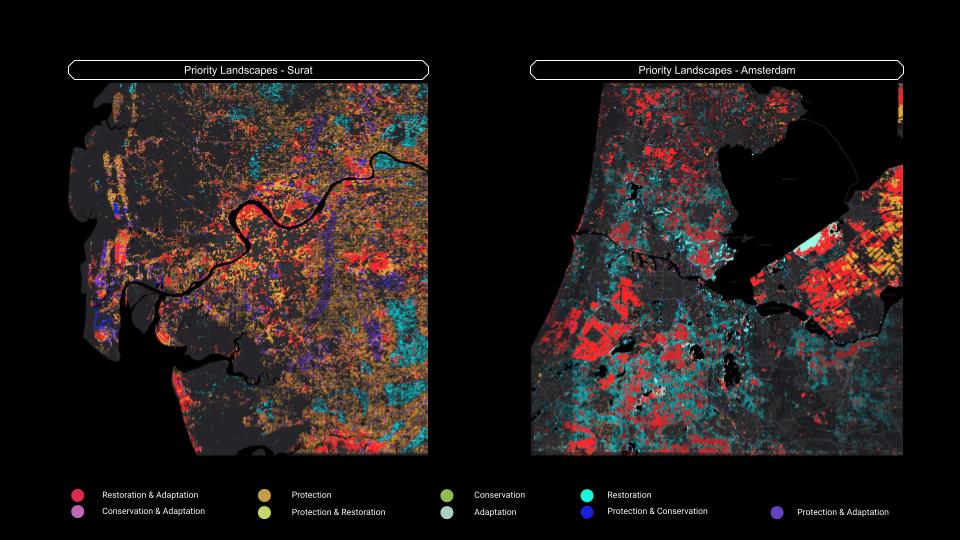
Based on the results, we find that in Surat, the majority of priority landscapes require protection due to significant exposure from rapid development this is seen in the core city and spread across some of the dense vegetation on the east side.. On the other hand, in Amsterdam, the priority landscapes are predominantly classified as restoration which is crucial as these landscapes do not perform optimally, mostly the agriculture lands.
INTERDEPENDENCIES
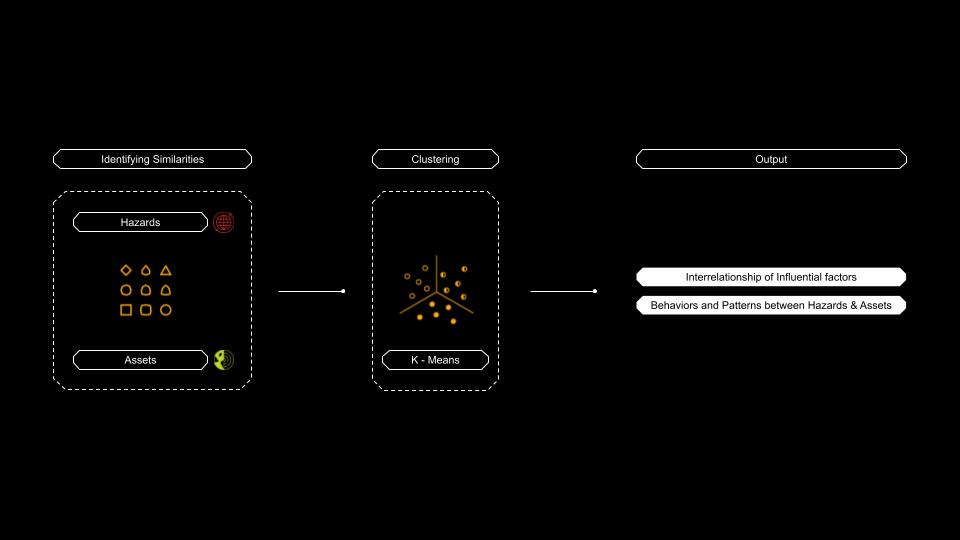
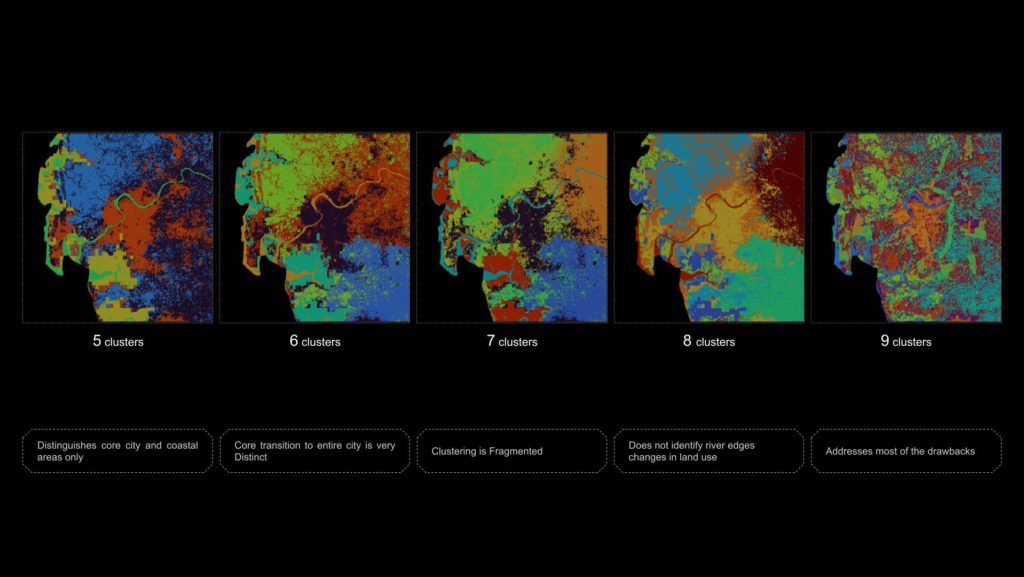
To analyze trade-offs and synergies between Hazards Determinants and Functional & Spatial determinants the methodology incorporates machine learning k means model. The k- means is a grouping algorithm that groups similar data points together which helps understand where spatially similar trends exist across variables. Several experiments were performed and the best suitable one is then taken into consideration.
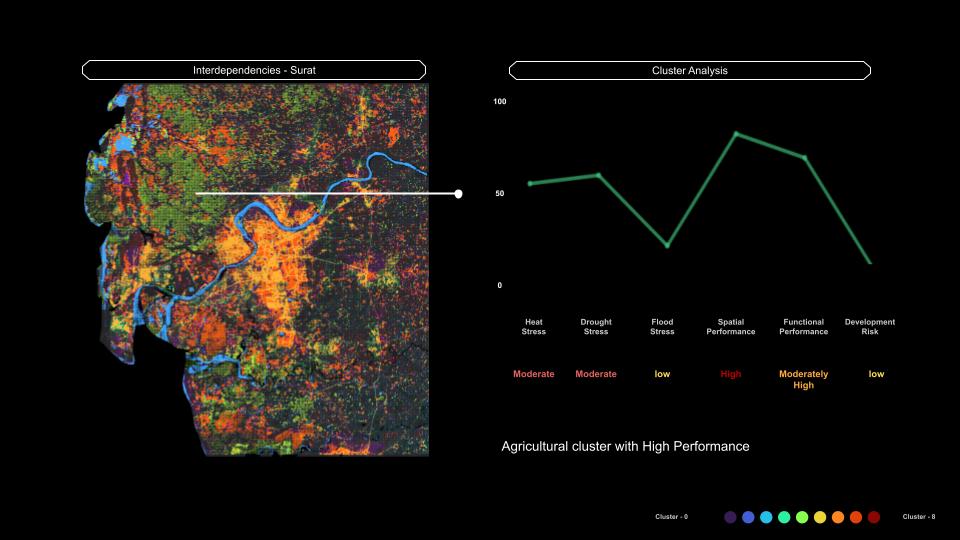
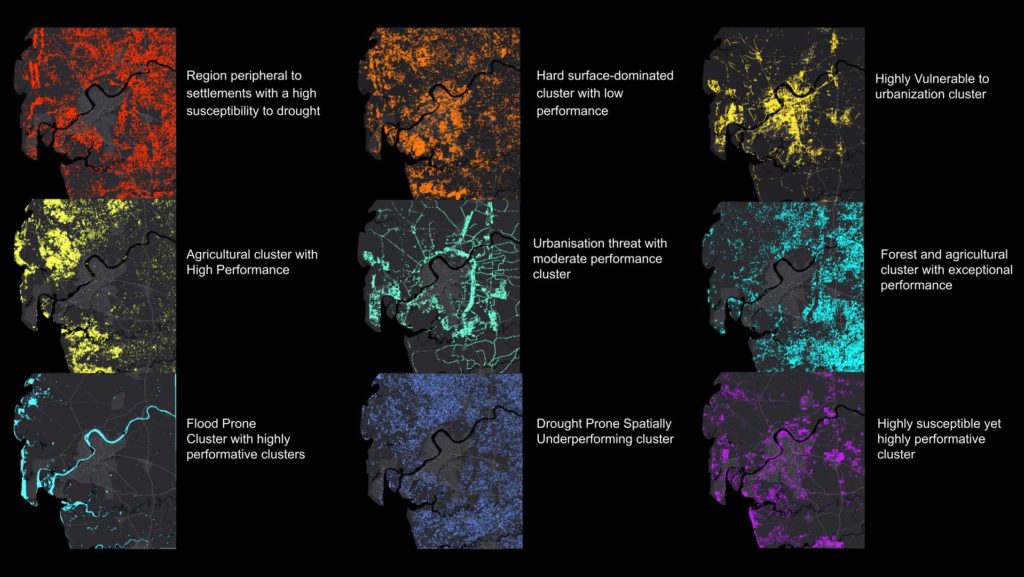
Each cluster has been studied in depth and behaviors are established to identify how geographically diverse areas have the same behaviour and also how the same geographical area could have more than one behavioral pattern. This indicates the presence of internal variations or sub-regions within the larger geographic boundary
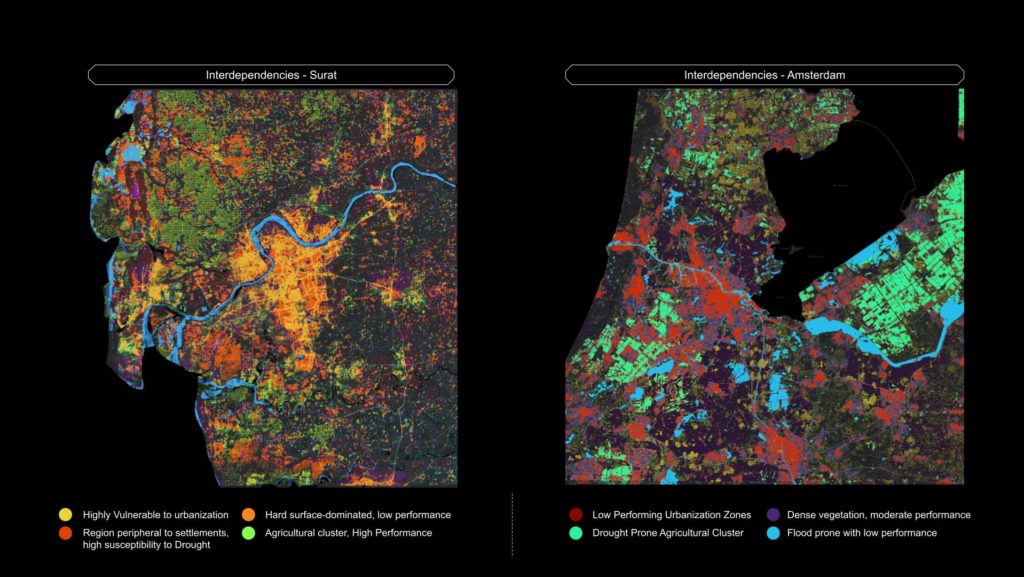
In both cities we find that one of the behaviour is the same which is the urban settlements with high susceptibility to urbanisation and very low Asset Performance. The most vulnerable clusters in Surat are the Hard surface-dominated cluster with low performance orange and the Regions peripheral to settlements with a high susceptibility to drought red while in amsterdam is the high drought prone agricultural cluster with low performance and the flood prone cluster. We can already start to observe how both cities behave in a different way by understanding interdependencies between parameters
STRATEGIES
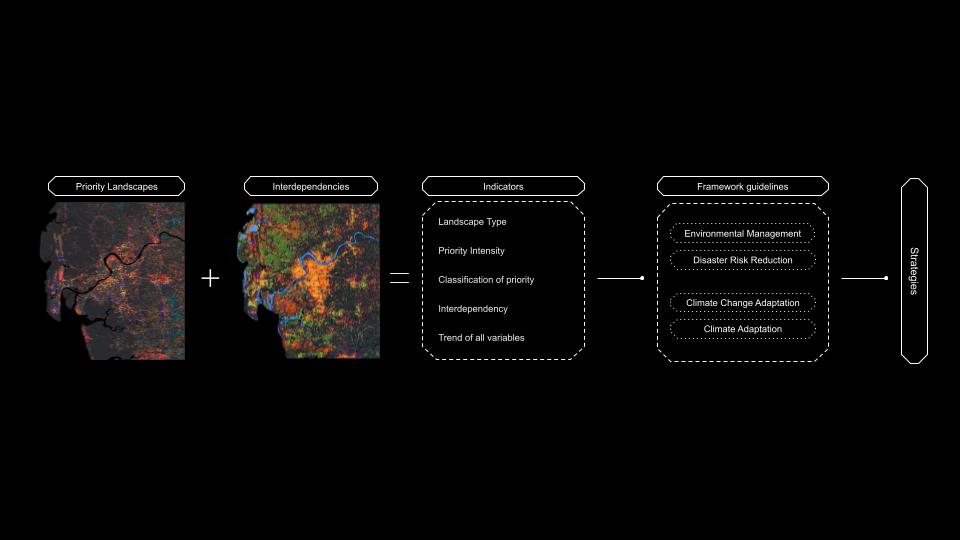
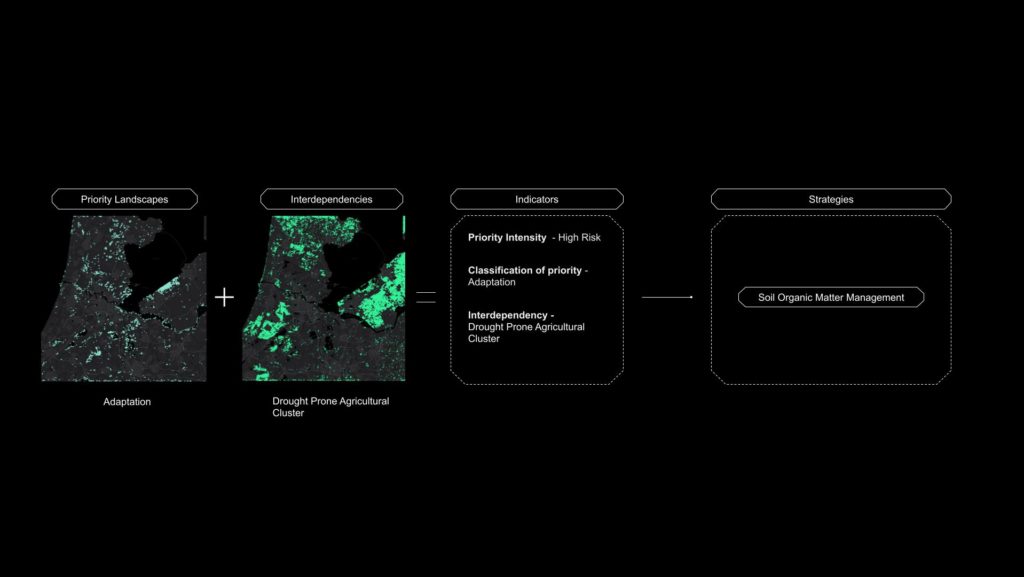
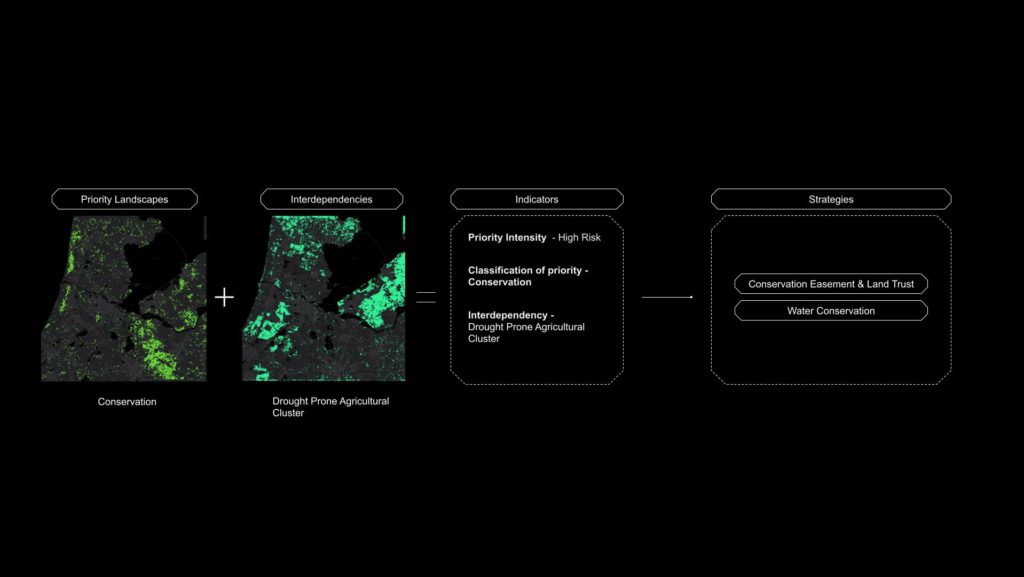
Observing these together provides a set of indicators for each 100m x 100m of the city. The priority landscape give us the land use of the landscape, the priority Urgency and its classification type. And the interrelationships and behaviors give us the cluster type with a comprehensive trend of all the variables used in the assessments. Using these indicators and referring to frameworks and guidelines certain strategies can be formulated.
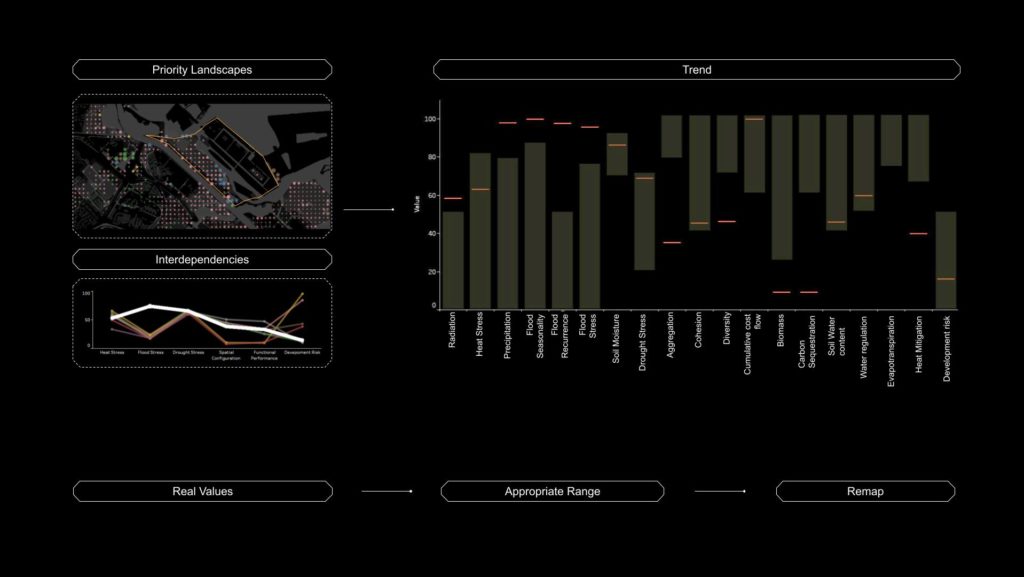
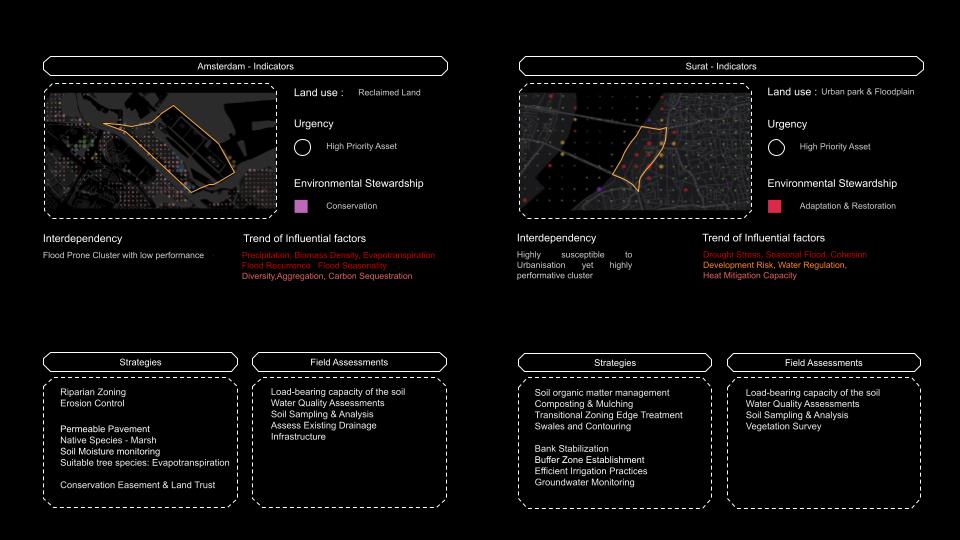
In conclusion, the EAR Tool is a comprehensive initiative focused on strengthening green infrastructure assets and achieving ecological balance in urban areas. By utilizing risk assessments, ecological metrics, and machine learning, the project provides valuable insights for sustainable land management and climate resilience. The project’s methodologies and analysis serve as a guide for decision-makers in addressing the challenges posed by environmental instability, fostering a more sustainable and resilient future for urban environments.
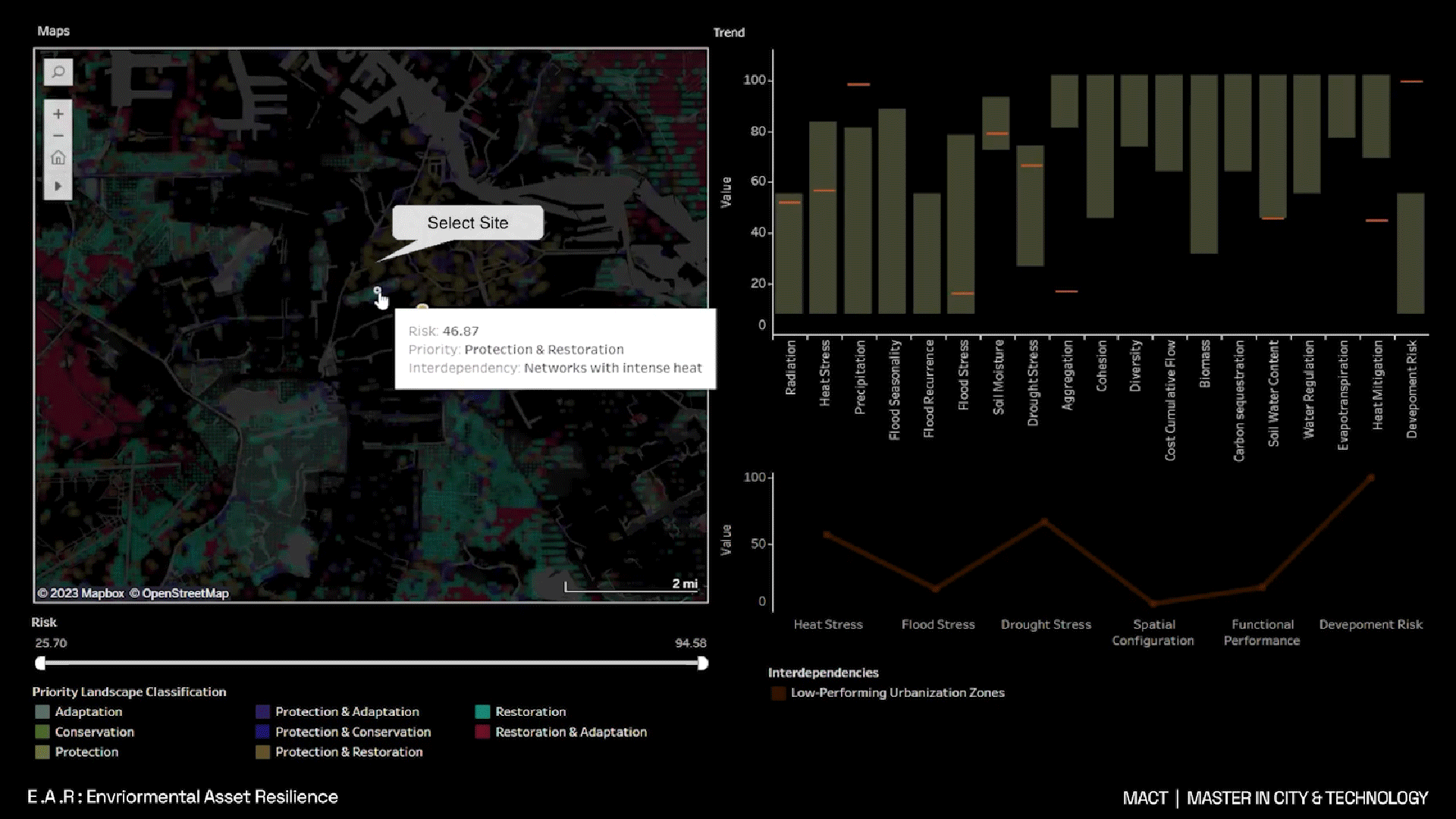
TOOL – https://drive.google.com/file/d/12cwIV7dPnyN2elLzWwAUgbLzaP2OhSS3/view