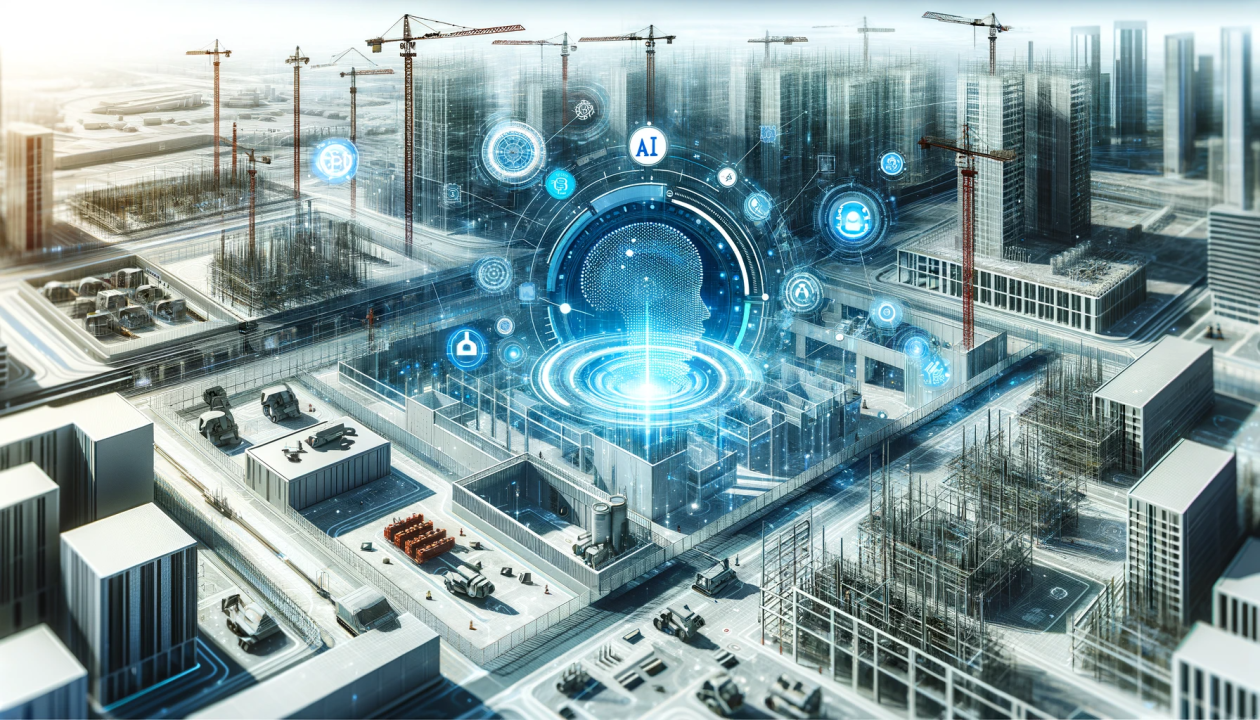
We’ve witnessed a fascinating shift from conventional to computational approaches, responsive designs, and the era of automation. Machine Learning, a term buzzing everywhere these days, has made its mark in our industry as well.
In architecture, ML, a subset of artificial intelligence, utilizes data to tackle challenges in computer-aided design by simulating various design alternatives for efficiency. Despite its infancy, ML faces adoption hurdles within architectural practice, notably due to limited collaboration between architects and data scientists, alongside issues like data quality and ethical considerations.
Practical applications include energy modeling with tools like “Delve,” analyzing natural light impact, and predictive maintenance such as “Neuron” by Arup, which monitors system health through real-time simulations using building sensor data. Autodesk Spacemaker employs a generative design for exploring alternatives based on predefined criteria.
The industry’s reliance on traditional methods presents a challenge to ML integration. Nonetheless, ML shows promise in generative design, energy modeling, and predictive maintenance, offering innovative solutions. In our podcasts, we’ll delve into the current status of ML in architectural practice and BIM, exploring methodologies and challenges hindering integration, as well as prospects for the future.
Podcast Research Statement
The nascent stage of Machine Learning adoption within architectural practice is hindered by challenges, including a lack of interdisciplinary collaboration between architects and data scientists, issues related to data quality, volume, variety, research limitations, data interoperability, and ethical considerations such as data privacy and concerns about potential job displacement.
Podcast Aim
Addressing challenges in collaboration, data quality, and ethics will be crucial in unlocking Machine Learning’s potential in architectural practice, yielding benefits like improved predictive analytics, resource optimization, project scheduling, risk management, and design optimization for enhanced efficiency and decision-making
Experts :
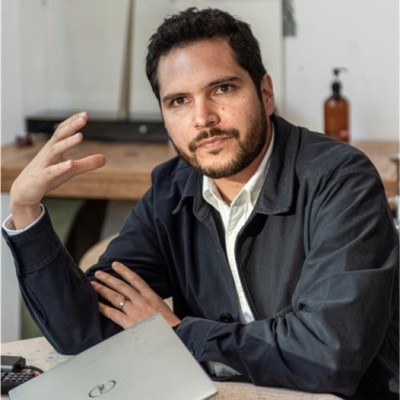
Pablo Zamorano, a senior associate and head of geometry and computational design at Heatherwick Studio, brings expertise in computational design, emerging technologies, and AI in architectural practice. One of his notable projects is the Expandable Surface Systems.
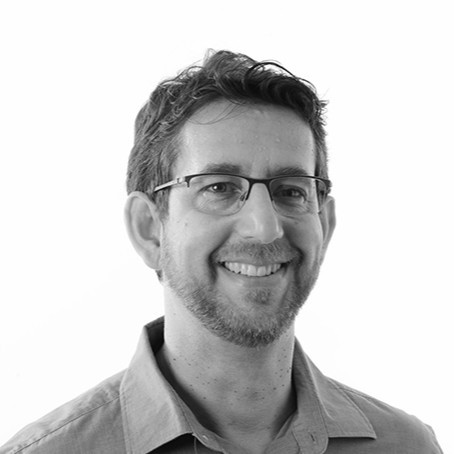
Guy Austern, holds a Ph.D. and is now a professor at the Technion – Israel Institute of Technology. He heads his own lab specializing in computational design and machine learning and also serves as Chief Science Officer at Beam Up. One of the significant projects is the latest paper on integrating context into architectural data using Graph Neural Networks.
Podcast Overview:
Across our podcasts, although centered on the same theme, variations arose due to the diverse expertise of our guests.
With Pablo, we explored the role of ML as a bridge between architecture and BIM. We discussed challenges within the AEC industry and contemplated the future impact of ML on architecture. This episode provided a strategic overview of AI and ML applications in architecture, emphasizing their potential to streamline processes.
In contrast, our conversation with Guy focused on a specific ML application in architecture. Starting from the convergence of architecture and computer science, given Guy’s dual bachelor’s degrees, we delved into his innovative work utilizing ML in BIM, including Graph Neural Networks and new terminology. Our discussion concluded with insights into the present and future status of ML in the industry. This episode delved into technical aspects, explaining how machines can be trained to interpret Architectural BIM data. Tailored to a niche audience, it serves as a valuable resource for those interested in ML and BIM integration.
Podcast Conclusion:
Both experts emphasized that machine learning holds significant potential for revolutionizing the AEC industry, addressing critical questions about optimizing design processes and driving advancements in business practices, construction methodologies, and environmental outcomes. Therefore, if technology, specifically machine learning, is the answer, the question lies in
“How to catalyze the transformation of the AEC industry to realize these potential benefits”