Data Encoding Seminar
MaCAD Digital Tools for DATA ENCODING & ML SEMINAR
Machine learning algorithms offer an alternative modelling paradigm for complex problems. Differently from analytical models where processes are computed using iterative time-based heavy calculations and pre-assigned property parameters, machine learning models learn by example through searching for an approximated relationship between the inputs and the targets. Once trained, they can be easily deployed within a design workflow to offer predictions. While these models offer advantages of accuracy and speed for iterative design, they are notoriously data-hungry. Many open source datasets are available online for training of state-of-the-art models, however these datasets are not relevant for architectural applications.
Dataset design is the enabler to using Machine Learning algorithms in different fields. While an existing ecology of algorithms provides architectures suitable to specific types of problems be classification or regression, the models must be trained on datasets that are specific to the problem they are expected to solve. They can be generated both computationally through heuristic algorithms, or by digitizing physical samples. Dataset design requires several criteria to be followed: Increased sample diversity, bias avoidance and ambiguity avoidance. These criteria ensure that the problem is well represented, with equal distribution and without confusion.
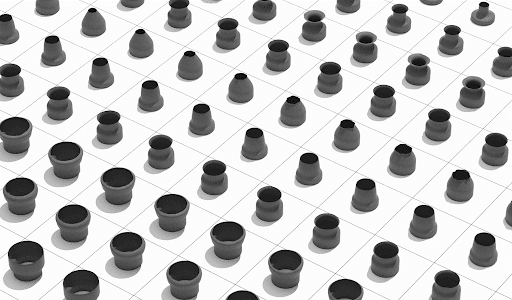
Source: Hesham Shawky & Cami Quinteros – Imaginary Vessels. MaCAD 2021
In this seminar, you will be introduced to conceptual perspectives around machine learning, dataset design and feature encoding. Through direct hands-on experience, you will explore the usage of machine learning to predict geometry. You will be tasked to develop a computational model to produce a generative dataset. Through the study of the parameter space and feature distribution and representation, you will propose a dataset encoding method, and evaluate it through the training of shallow models as well as artificial neural networks, and represent the predicted outcome in the design space.
Learning Objectives
At course completion the student will:
- Introduced to fundamental machine learning concepts and workflows.
- Understand notions of parameter space, data encoding and feature selection
- Acquire competences in developing generative datasets for architectural application
- Acquire competences in feature engineering such as dimensionality reduction, data analysis
- Acquire a hands-on experience in using state of the art machine learning libraries
- Develop appropriate representational methods and tools to showcase your findings
- Collaborate effectively within an online group-working context